TinyPuff: Automated design of Tiny Smoking Puff Classifiers for Body Worn Devices
PROCEEDINGS OF THE 2023 8TH WORKSHOP ON BODY-CENTRIC COMPUTING SYSTEMS, BODYSYS 2023(2023)
摘要
Smoking is a significant cause of death and deterioration of health worldwide, affecting active and passive smokers. Cessation of smoking contributes to an essential health and wellness application owing to the broad range of health problems such as cancer, hypertension, and several cardiopulmonary diseases. Personalized smoking-cessation applications can be very effective in helping users to stop smoking if there are detections and interventions done at the right time. This requires real-time detection of smoking puffs. Such applications are made feasible by day-long monitoring and smoking puff detection from unobtrusive devices such as wearables. This paper proposes a deep inference technique for the real-time detection of smoking puffs on a wearable device. We show that a simple, sequential Convolutional Neural Network (CNN) using only 6-axis Inertial signals can be utilized in place of complex and resource-consuming Deep Learning models. The accuracy achieved is comparable to State-of-the-Art techniques with an F1 score of 0.81, although the model size is tiny - 114 kB. Such small models can be deployed on the lowest configuration hardware platforms, achieving accurate but high-speed, low-power inference on conventional smartwatches. We ensure that the auto-designed models are directly compatible with resource-constrained platforms such as TensorFlow Lite and TensorFlow Lite for Microcontrollers (TFLM) without requiring further use of model reduction and optimization techniques. Our proposed approach will allow affordable wearable device manufacturers to run smoking detection on their devices, as it is tiny enough to fit TinyML platforms and is only dependent on IMU sensors that are universally available.
更多查看译文
关键词
Puff Detection,NAS,Inertial Signals,Wearable health
AI 理解论文
溯源树
样例
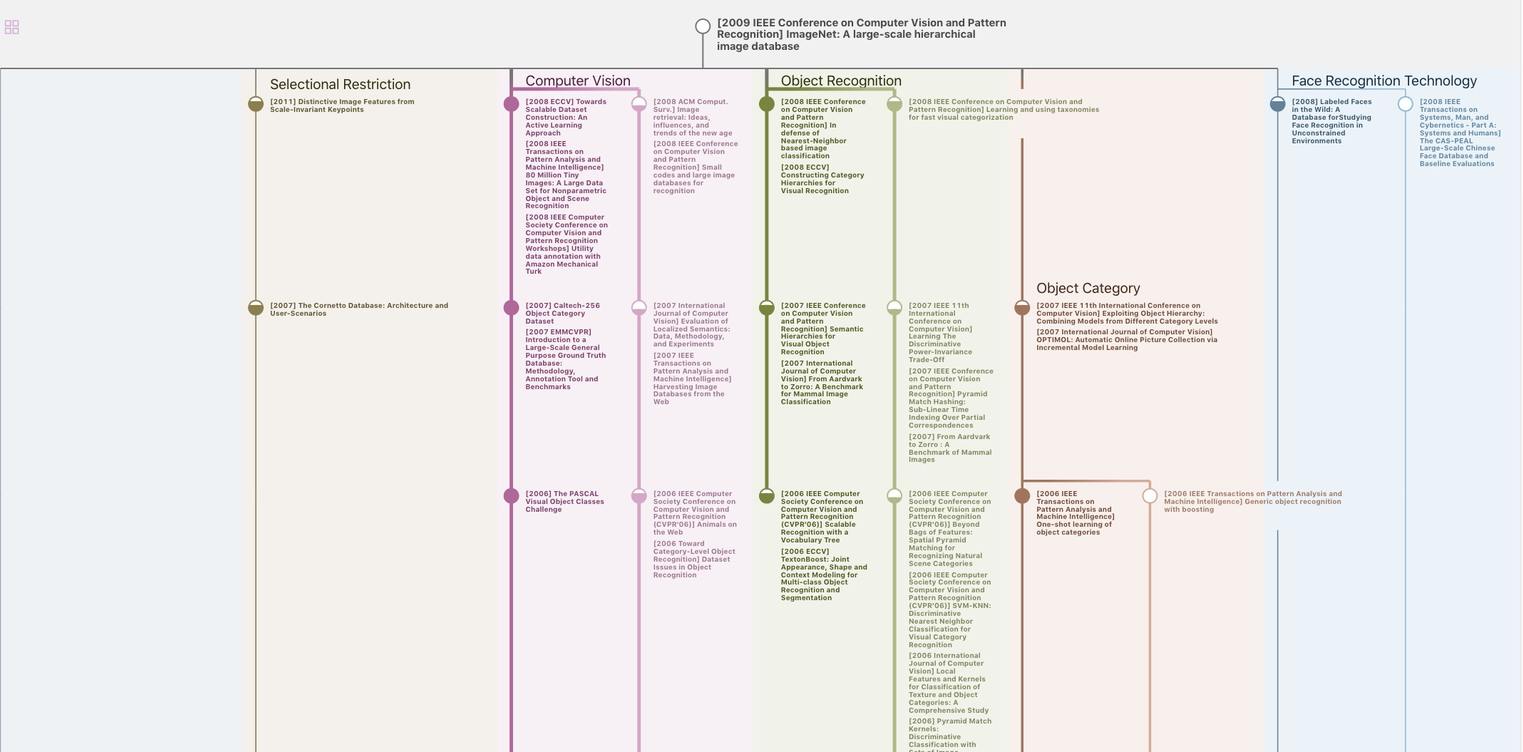
生成溯源树,研究论文发展脉络
Chat Paper
正在生成论文摘要