Robust Hypergraph-Augmented Graph Contrastive Learning for Graph Self-Supervised Learning
2023 2ND ASIA CONFERENCE ON ALGORITHMS, COMPUTING AND MACHINE LEARNING, CACML 2023(2023)
摘要
Graph contrastive learning has emerged as a promising method for self-supervised graph representation learning. The traditional framework conventionally imposes two graph views generated by leveraging graph data augmentations. Such an approach focuses on leading the model to learn discriminative information from graph local structures, which brings up an intrinsic issue that the model partially fails to obtain sufficient discriminative information contained by the graph global information. To this end, we propose a hypergraph-augmented view to empower the self-supervised graph representation learning model to better capture the global information from nodes and corresponding edges. In the further exploration of the graph contrastive learning, we discover a principal challenge undermining conventional contrastive methods: the false negative sample problem, i.e., specific negative samples actually belong to the same category of the anchor sample. To address this issue, we take the neighbors of nodes into consideration and propose the robust graph contrastive learning. In practice, we empirically observe that the proposed hypergraph-augmented view can further enhance the robustness of graph contrastive learning by adopting our framework. Based on these improvements, we propose a novel method called Robust Hypergraph-Augmented Graph Contrastive Learning (RH-GCL). We conduct various experiments in the settings of both transductive and inductive node classification. The results demonstrate that our method achieves the state-of-the-art (SOTA) performance on different datasets. Specifically, the accuracy of node classification on Cora dataset is 84.4%, which is 1.1% higher than that of GRACE. We also perform the ablation study to verify the effectiveness of each part of our proposed method.
更多查看译文
关键词
graph contrastive learning,self-supervised,hypergraph,multi-view
AI 理解论文
溯源树
样例
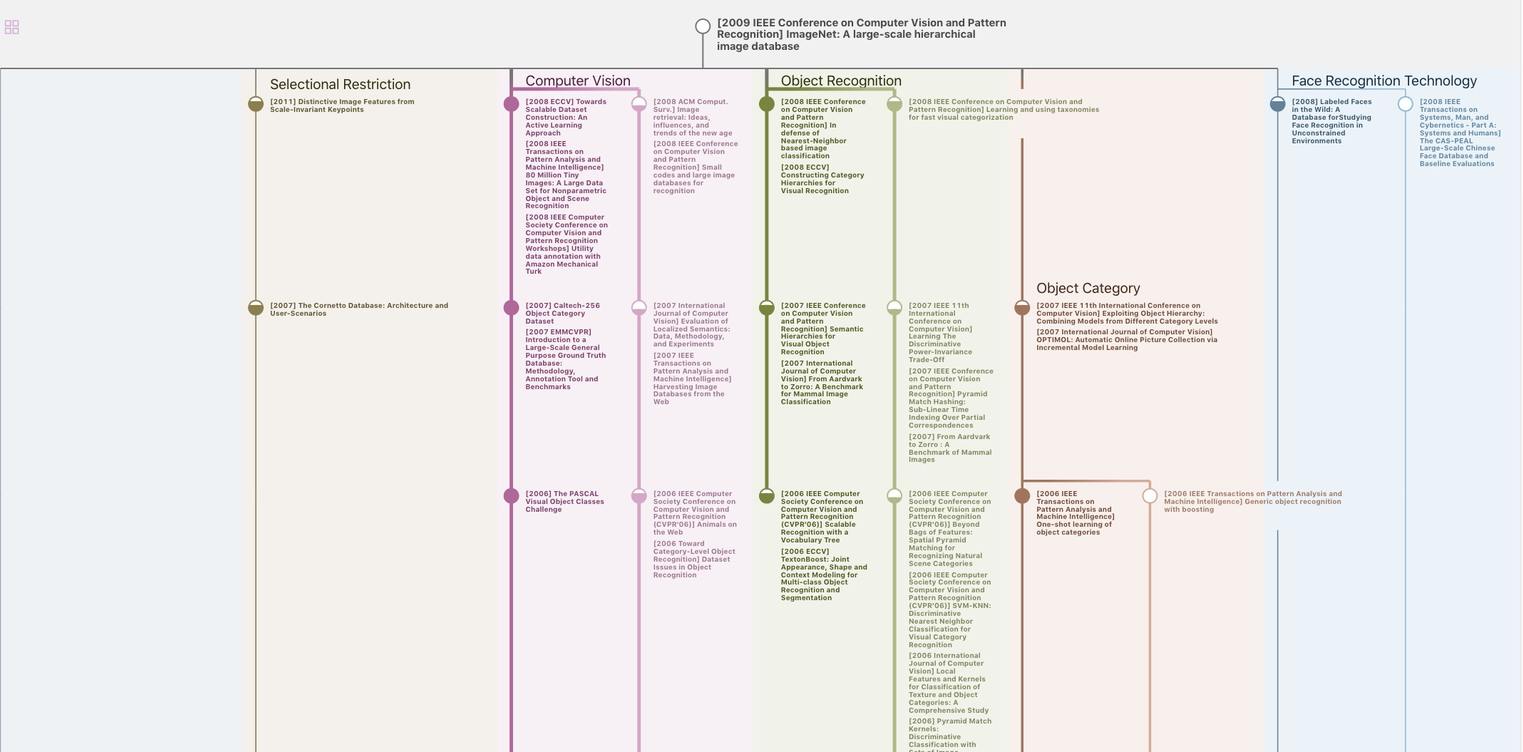
生成溯源树,研究论文发展脉络
Chat Paper
正在生成论文摘要