Settlement Growth Prediction exploiting EO-based Time Series with the Spatio-Temporal Matrix Approach: a Case Study for the City of Hue, Vietnam.
JURSE(2023)
Abstract
Satellite-based Earth observation (EO) time series data possess enormous potential for analyzing the past and forecasting future trends of urban/settlement development. While historic settlement extent maps with high spatial resolution can be generated from EO data, detailed local information such as intra-urban recreation spaces or restricted areas for specific land use types are hard to acquire. In order to overcome this data gap from which many modelling approaches suffer, the Spatio-Temporal Matrix (STM) was developed. The STM provides spatial and temporal characteristics of a target pixels’ neighborhood to be used for predicting the future urban/settlement growth with a machine learning approach. In this study, a multi-layer perceptron (MLP) was employed to utilize the STM for the settlement growth prediction of the City of Hue, Vietnam. The SLEUTH model was used as a benchmark for the performance evaluation. The results show that the STM-based model achieved a high accuracy in settlement growth modelling. Compared to the SLEUTH model, the STM approach simulated less growth in restricted areas without having to rely on external datasets.
MoreTranslated text
Key words
Spatio-temporal matrix,urban growth,settlement growth,prediction,EO data,time series,machine learning
AI Read Science
Must-Reading Tree
Example
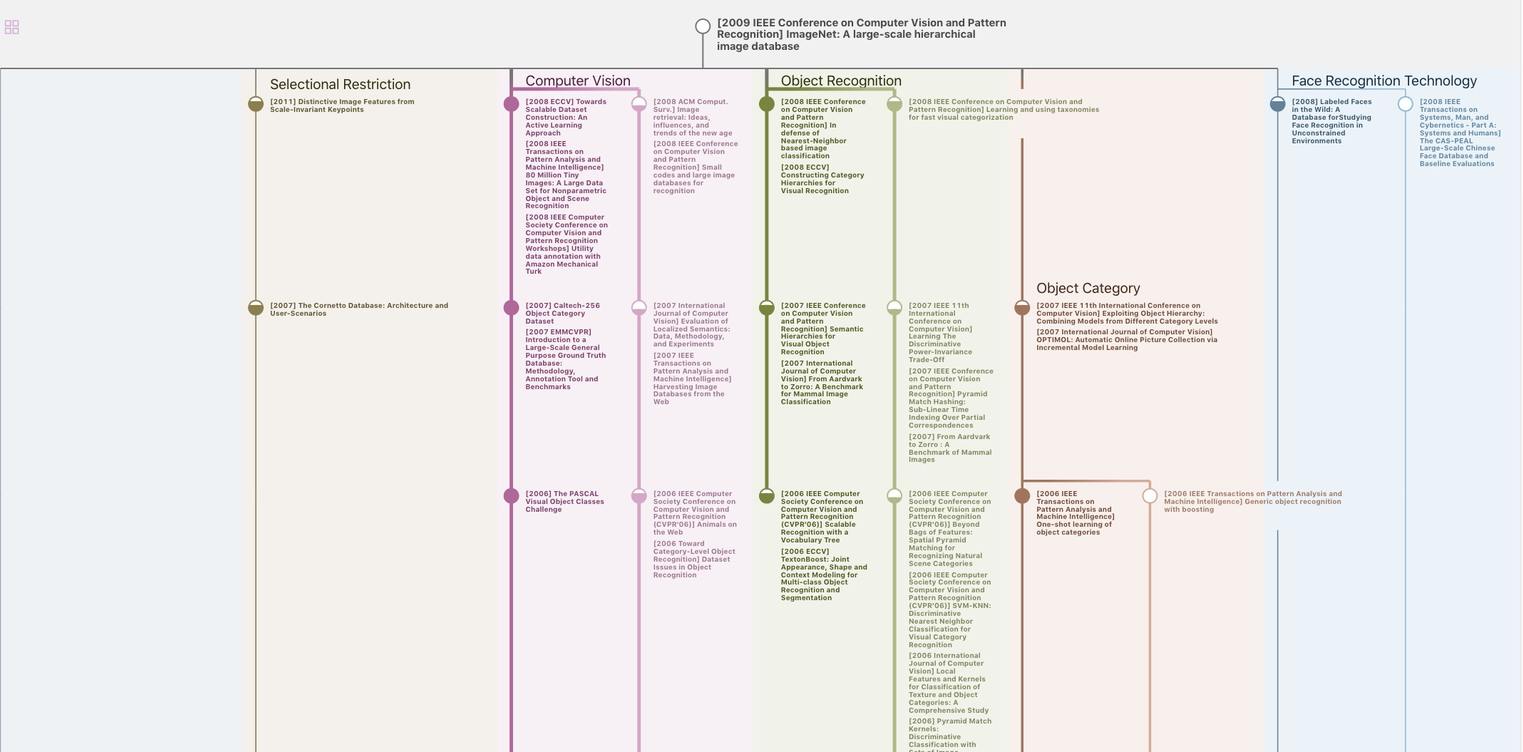
Generate MRT to find the research sequence of this paper
Chat Paper
Summary is being generated by the instructions you defined