Energy-efficient Hardware Acceleration of Shallow Machine Learning Applications
2023 DESIGN, AUTOMATION & TEST IN EUROPE CONFERENCE & EXHIBITION, DATE(2023)
摘要
ML accelerators have largely focused on building general platforms for deep neural networks (DNNs), but less so on shallow machine learning (SML) algorithms. This paper proposes Axiline, a compact, configurable, template-based generator for SML hardware acceleration. Axiline identifies computational kernels as templates that are common to these algorithms and builds a pipelined accelerator for efficient execution. The dataflow graphs of individual ML instances, with different data dimensions, are mapped to the pipeline stages and then optimized by customized algorithms. The approach generates energy-efficient hardware for training and inference of various ML algorithms, as demonstrated with post-layout FPGA and ASIC results.
更多查看译文
AI 理解论文
溯源树
样例
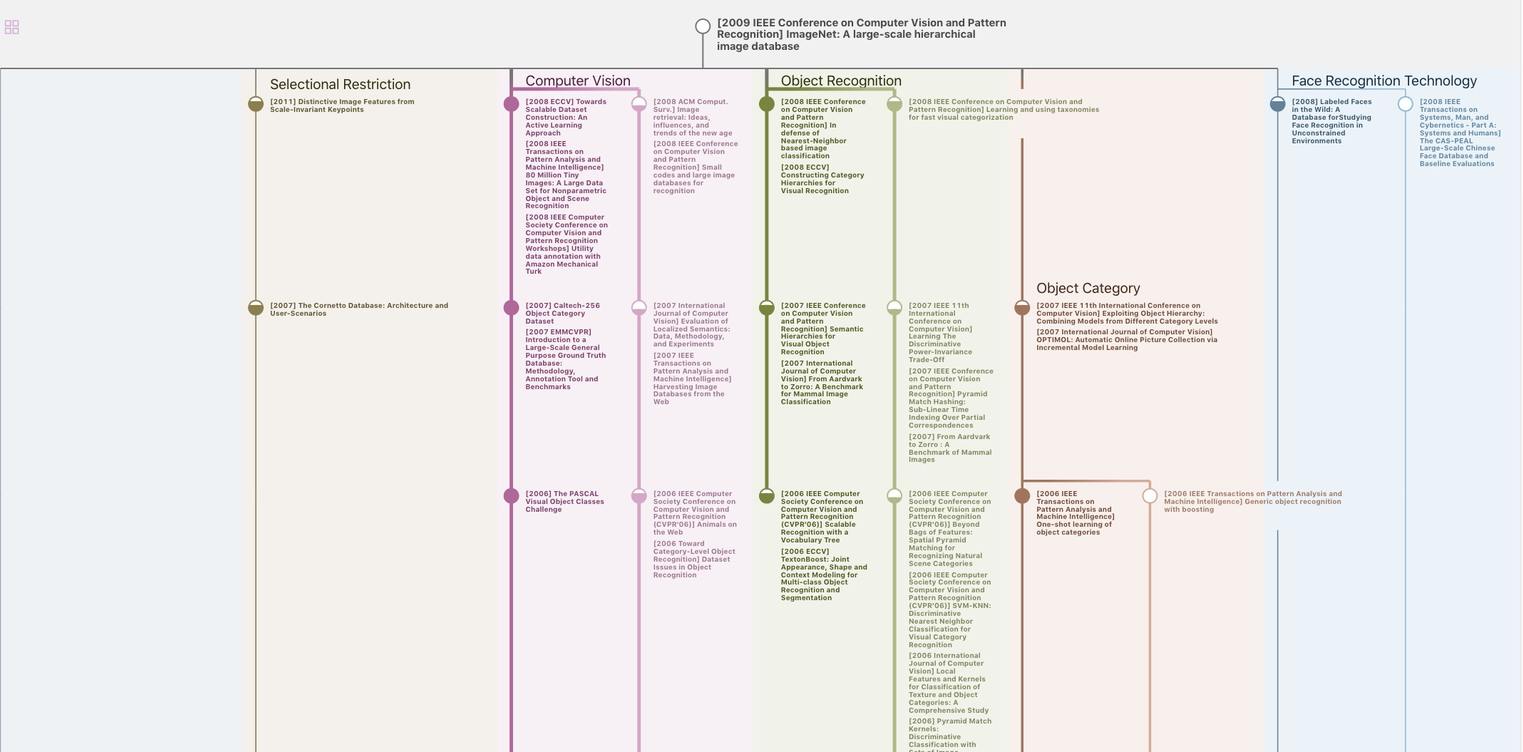
生成溯源树,研究论文发展脉络
Chat Paper
正在生成论文摘要