Smart Knowledge Transfer-based Runtime Power Management
2023 DESIGN, AUTOMATION & TEST IN EUROPE CONFERENCE & EXHIBITION, DATE(2023)
摘要
As Moore's law slows down, computing systems must pivot towards higher energy efficiency to continue scaling performance. Reinforcement learning (RL) performs more adaptively than conventional methods in runtime power management under varied hardware configurations and varying software workloads. However, prior works on either model-free or model-based RL approaches face a non-negligible challenge: relearning the policies to adapt to the new environment is unacceptably time-consuming, especially when encountering significant variances in workloads or hardware configurations. Moreover, existing research on accelerating learning has focused on the speedup while largely ignoring the efficiency degradation of the results. In this paper, we present a smart transfer-enabled Q-learning (STQL) approach to boost the learning process and guarantee the learning efficiency through a contradiction checking mechanism, which wisely evicts inappropriate transferred knowledge. Experiments on realistic applications show that the proposed method can speed up the learning process to up to 2.3x and achieve a 6.2% energy-delay product (EDP) reduction compared to the state-of-the-art design.
更多查看译文
关键词
power management,reinforcement learning,online learning,transfer learning,multicore system
AI 理解论文
溯源树
样例
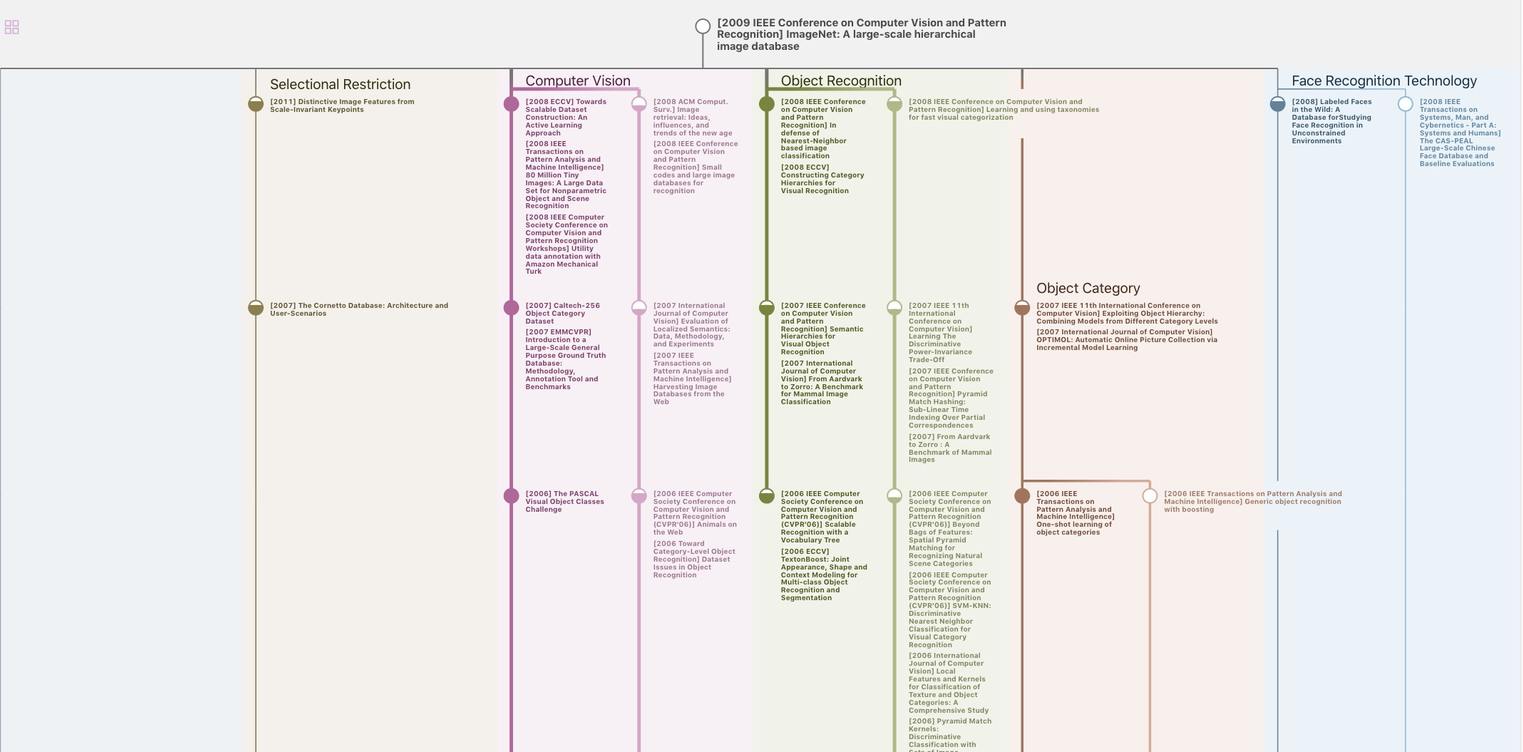
生成溯源树,研究论文发展脉络
Chat Paper
正在生成论文摘要