The CNN vs. SNN Event-camera Dichotomy and Perspectives For Event-Graph Neural Networks
2023 DESIGN, AUTOMATION & TEST IN EUROPE CONFERENCE & EXHIBITION, DATE(2023)
摘要
Since neuromorphic event-based pixels and cameras were first proposed, the technology has greatly advanced such that there now exists several industrial sensors, processors and toolchains. This has also paved the way for a blossoming new branch of AI dedicated to processing the event-based data these sensors generate. However, there is still much debate about which of these approaches can best harness the inherent sparsity, low-latency and fine spatiotemporal structure of event-data to obtain better performance and do so using the least time and energy. The latter is of particular importance since these algorithms will typically be employed near or inside of the sensor at the edge where the power supply may be heavily constrained. The two predominant methods to process visual events - convolutional and spiking neural networks - are fundamentally opposed in principle. The former converts events into static 2D frames such that they are compatible with 2D convolutions, while the latter computes in an event-driven fashion naturally compatible with the raw data. We review this dichotomy by studying recent algorithmic and hardware advances of both approaches. We conclude with a perspective on an emerging alternative approach whereby events are transformed into a graph data structure and thereafter processed using techniques from the domain of graph neural networks. Despite promising early results, algorithmic and hardware innovations are required before this approach can be applied close or within the Event-based sensor.
更多查看译文
关键词
Event-camera,Edge AI,neuromorphic computing
AI 理解论文
溯源树
样例
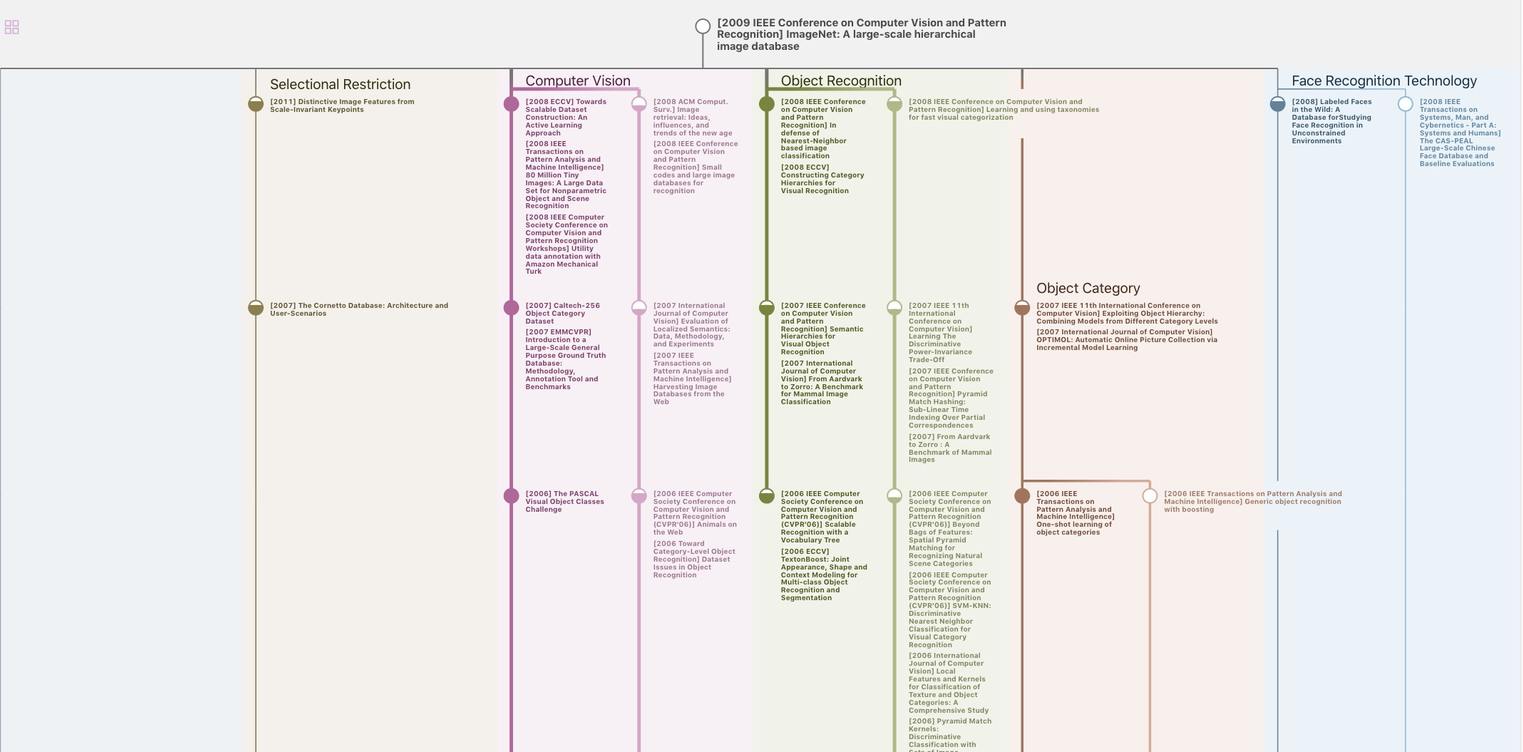
生成溯源树,研究论文发展脉络
Chat Paper
正在生成论文摘要