Simultaneous Detection and Estimation of False Data Injection Attacks in Cyber-Physical Battery Systems using a Learning Observer
ICCAD(2023)
摘要
This work is to present a learning observer-based method for simultaneous detection and estimation of false data injection attacks (FDIAs) to the cyber-physical battery systems. The original battery system in a state-space formulation is transformed into two separate subsystems: one contains both disturbances and the FDIAs and the second one is free from disturbances but subject to FDIAs. A learning observer is then designed for the second subsystem such that the FDIA signals can be estimated and further detected without being affected by the disturbances. This makes the proposed learning observer-based detection and estimation method is robust to disturbances and false declaration of FDIAs can be avoided. Another advantage of the proposed method is that the computing load is low because of the design of a reduced-order learning observer. With a three-cell battery string, a simulation study is employed to verify the effectiveness of proposed detection and estimation method for the FDIAs.
更多查看译文
关键词
Cyber-physical battery systems,false data injection attacks,learning observers,system transformation
AI 理解论文
溯源树
样例
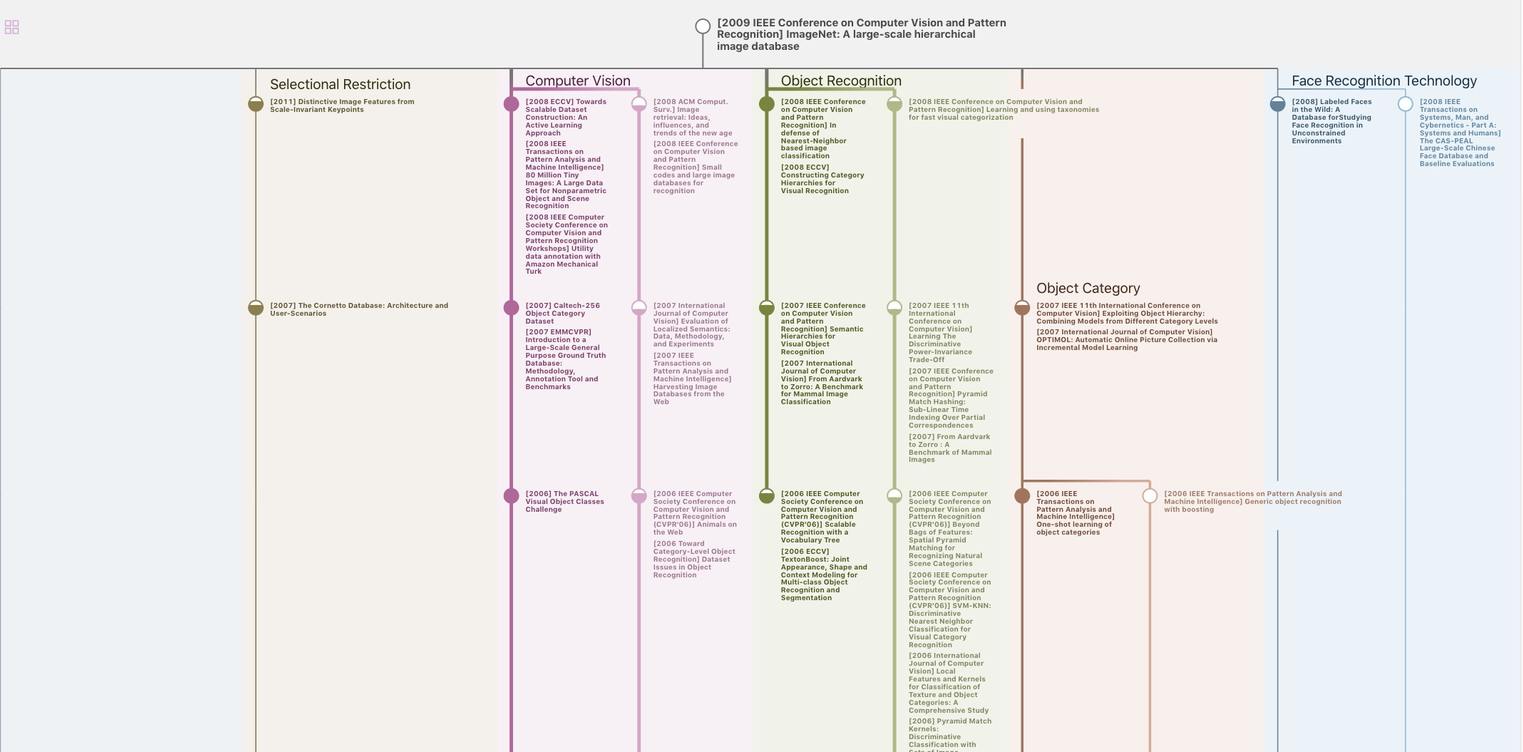
生成溯源树,研究论文发展脉络
Chat Paper
正在生成论文摘要