Maritime Federated Learning for Decentralized On-Ship Intelligence.
AIAI (2)(2023)
摘要
Maritime trade unavoidably influences the economy, transportation and market worldwide. Deploying efficient, privacy-preserving and environmental-friendly solutions in maritime environments requires a continuous global effort. There are many obstacles associated with the centralized optimization for on-sail ships, including data security violation, signaling overhead and high-latency response. Contradictorily, the emerging Federated Learning (FL) paradigm has been proposed as an efficient solution for promoting data privacy, low latency and high communication efficiency. In this context, this paper proposes a maritime-based FL scheme to ensure distributed, collaborative and secure optimization for enabling predictive on-ship intelligence. A scenario targeting the accurate prediction of the Primary Engine Power (PEP) of large cargo ships is considered, as the output PEP is directly proportional to fuel consumption. Based on real data, several Machine Learning (ML) models were tested and validated, in terms of their PEP prediction accuracy, with all models exploiting both weather- and ship-related data. Simulation results towards the selection of the PEP predictor, as well as the quantification of the FL scheme efficacy over traditional benchmarks were carried out, achieving a beneficial equilibrium between the prediction accuracy, data privacy and communication efficiency.
更多查看译文
关键词
learning,intelligence,on-ship
AI 理解论文
溯源树
样例
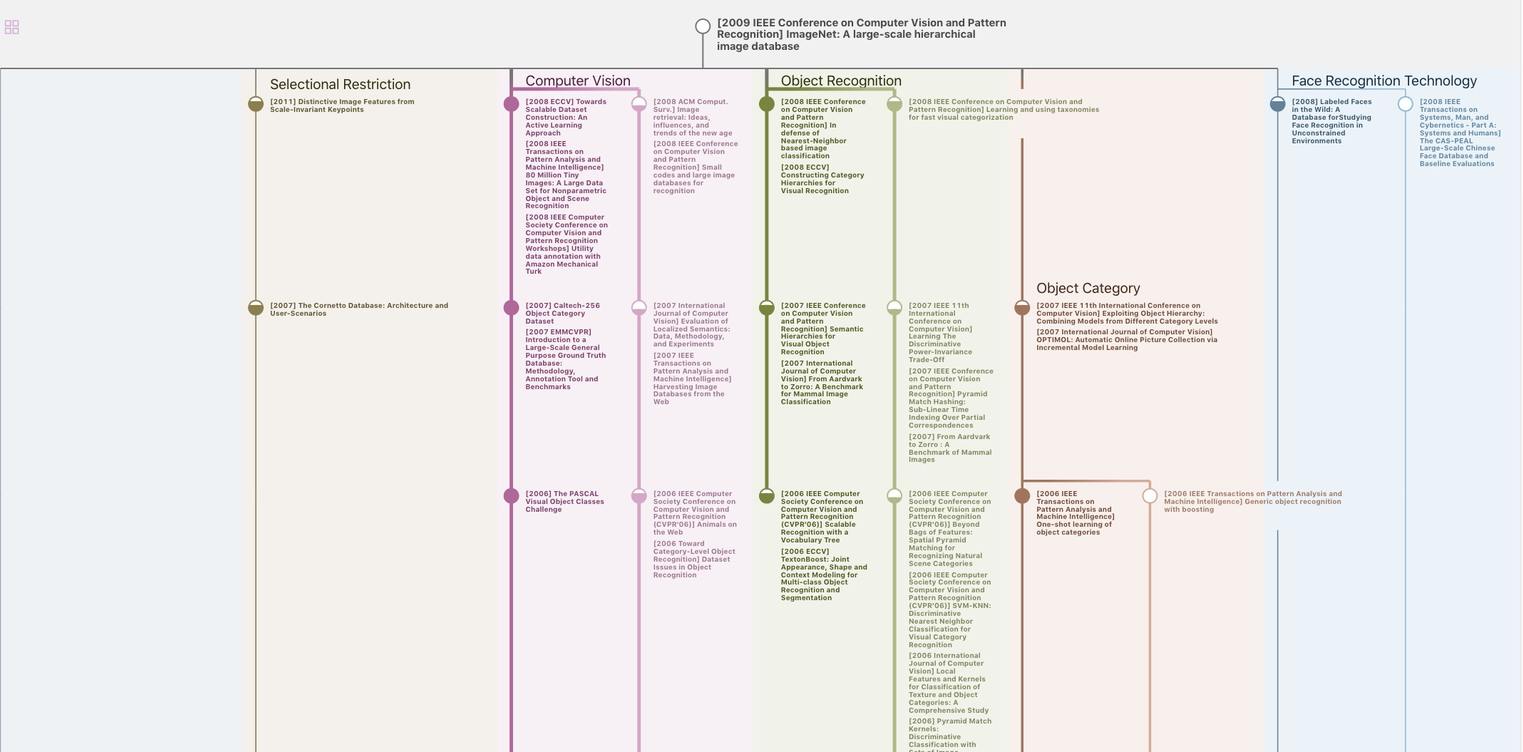
生成溯源树,研究论文发展脉络
Chat Paper
正在生成论文摘要