Loss-Customised Probabilistic Energy Time Series Forecasts Using Automated Hyperparameter Optimisation
PROCEEDINGS OF THE 2023 THE 14TH ACM INTERNATIONAL CONFERENCE ON FUTURE ENERGY SYSTEMS, E-ENERGY 2023(2023)
摘要
To mitigate climate change, renewable energy sources are increasingly integrated into the energy system. Due to their volatility and uncertainty, smart grid applications are required to deal with this uncertainty and maintain grid stability. To operate effectively and in an automated manner, each of these applications requires a quantification of the uncertainty in future demand and generation, which probabilistic forecasts can provide. Furthermore, these applications often require specific probabilistic forecast properties, such as coverage rate and sharpness. However, existing probabilistic forecasts cannot be easily customised to exhibit these required properties. Therefore, we present a novel approach that creates loss-customised probabilistic forecasts using automated hyperparameter optimisation based on custom loss metrics. We combine a deterministic base forecaster and a conditional Invertible Neural Network to include specified uncertainty in a deterministic forecast. This uncertainty is defined by automated hyperparameter optimisation based on flexible and adaptable loss metrics, enabling the generation of loss-customised probabilistic forecasts with different properties without computationally expensive retraining. We evaluate our approach on four real-world data sets and compare the generated loss-customised forecasts with three state-of-the-art probabilistic forecasting benchmarks. We show that our approach generates probabilistic forecasts that can be customised to achieve state-of-the-art performance in either Continuous Ranked Probability Score, Pinball Loss, or Coverage Rate Error, depending on the selected customised loss metric.
更多查看译文
关键词
probabilistic forecasting,conditional invertible neural network,automated machine learning,energy time series
AI 理解论文
溯源树
样例
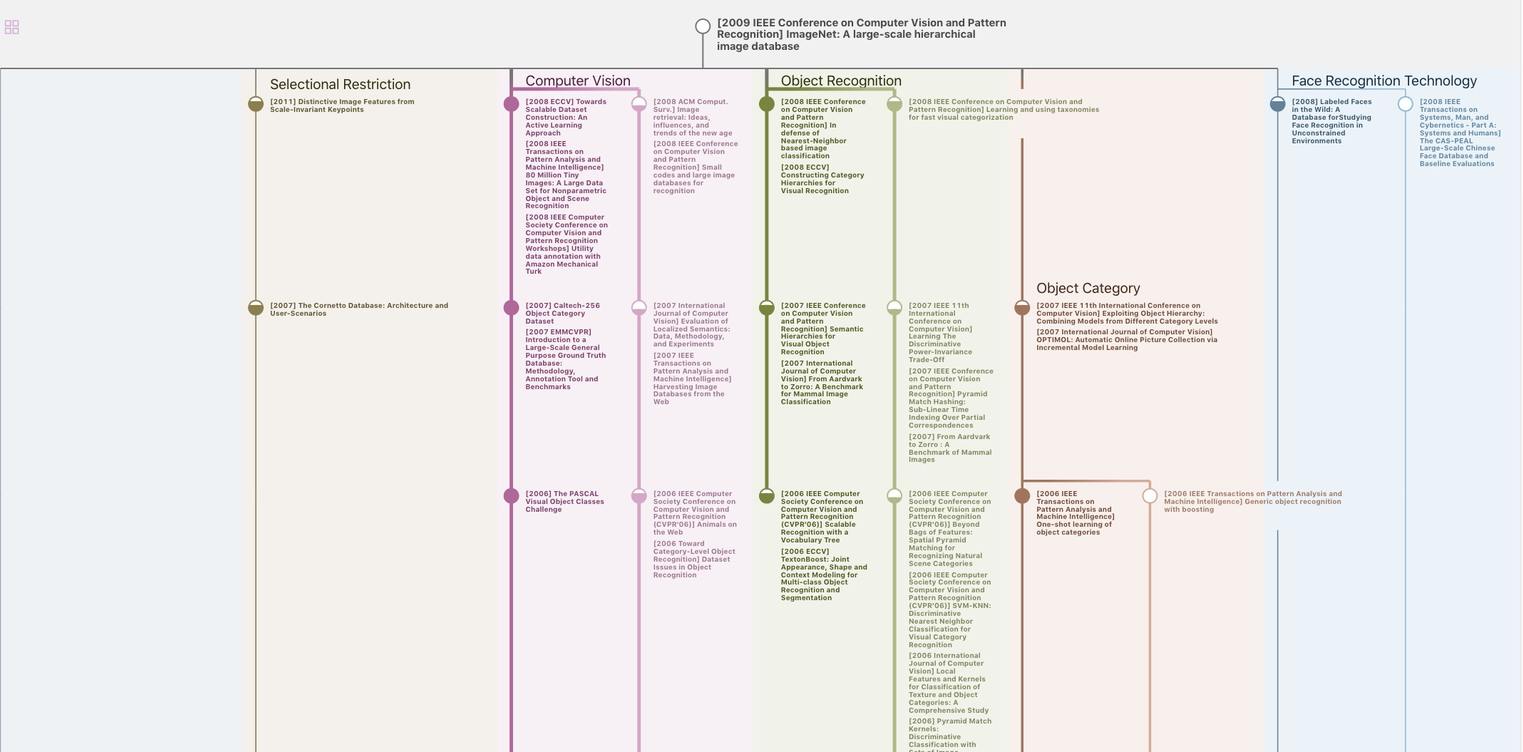
生成溯源树,研究论文发展脉络
Chat Paper
正在生成论文摘要