S2DGAN: Generating Dual-energy CT from Single-energy CT for Real-time Determination of Intracerebral Hemorrhage
INFORMATION PROCESSING IN MEDICAL IMAGING, IPMI 2023(2023)
摘要
Timely determination of whether there is intracerebral hemorrhage after thrombectomy is essential for follow-up treatment. But, this is extremely challenging with standard single-energy CT (SECT), because blood and contrast agents (injected during thrombectomy) have similar CT values under a single energy spectrum. In contrast, dualenergy CT (DECT) employs two different energy spectra, thus allowing to differentiate between hemorrhage and contrast extravasation in real time, based on energy-related attenuation characteristics between blood and contrast. However, compared to SECT scanners, DECT scanners have limited popularity due to high price. To address this dilemma, in this paper we first attempt to generate pseudo DECT images from a SECT image for real-time diagnosis of hemorrhage. More specifically, we propose a SECT-to-DECT generative adversarial network (S2DGAN), which is a 3D transformer-based multi-task learning framework equipped with a shared attention mechanism. Among them, the transformer-based architecture can guide S2DGAN to focus more on high-density areas (crucial for hemorrhage diagnosis) during the generation. Meanwhile, the introduced multi-task learning strategy and shared attention mechanism enable S2DGAN to model dependencies between interconnected generation tasks, improving generation performance while significantly reducing model parameters and computational complexity. Validated on clinical data, S2DGAN can generate DECT images better than state ofthe-art methods and achieve an accuracy of 90% in hemorrhage diagnosis based only on SECT images.
更多查看译文
关键词
intracerebral hemorrhage,dual-energy,single-energy,real-time
AI 理解论文
溯源树
样例
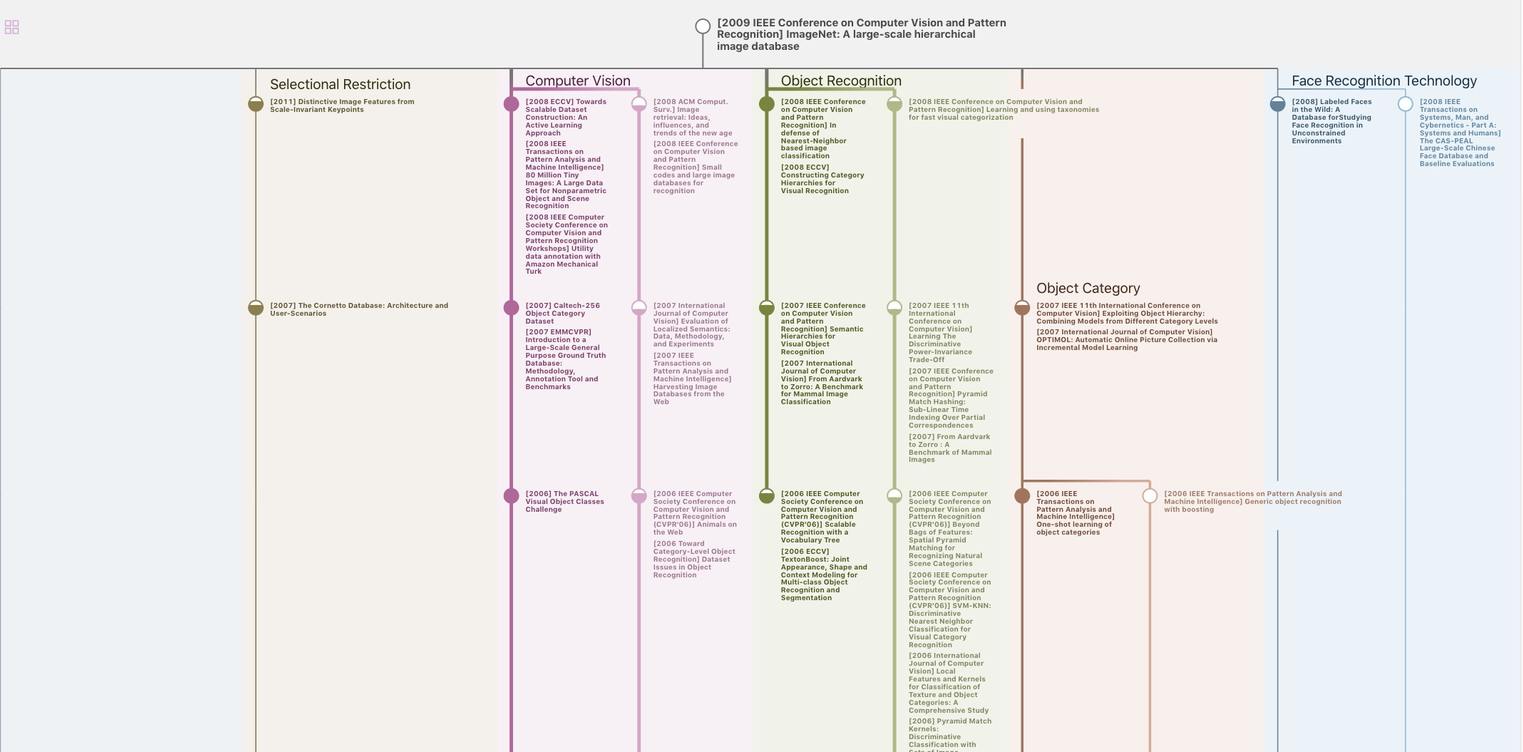
生成溯源树,研究论文发展脉络
Chat Paper
正在生成论文摘要