A Critical Analysis of Classifier Selection in Learned Bloom Filters: The Essentials.
EANN(2023)
Abstract
It is well known that Bloom Filters have a performance essentially independent of the data used to query the filters themselves, but this is no more true when considering Learned Bloom Filters. In this work we analyze how the performance of such learned data structures is impacted by the classifier chosen to build the filter and by the complexity of the dataset used in the training phase. Such analysis, which has not been proposed so far in the literature, involves the key performance indicators of space efficiency, false positive rate, and reject time. By screening various implementations of Learned Bloom Filters, our experimental study highlights that only one of these implementations exhibits higher robustness to classifier performance and to noisy data, and that only two families of classifiers have desirable properties in relation to the previous performance indicators.
MoreTranslated text
Key words
learned bloom filters,classifier selection
AI Read Science
Must-Reading Tree
Example
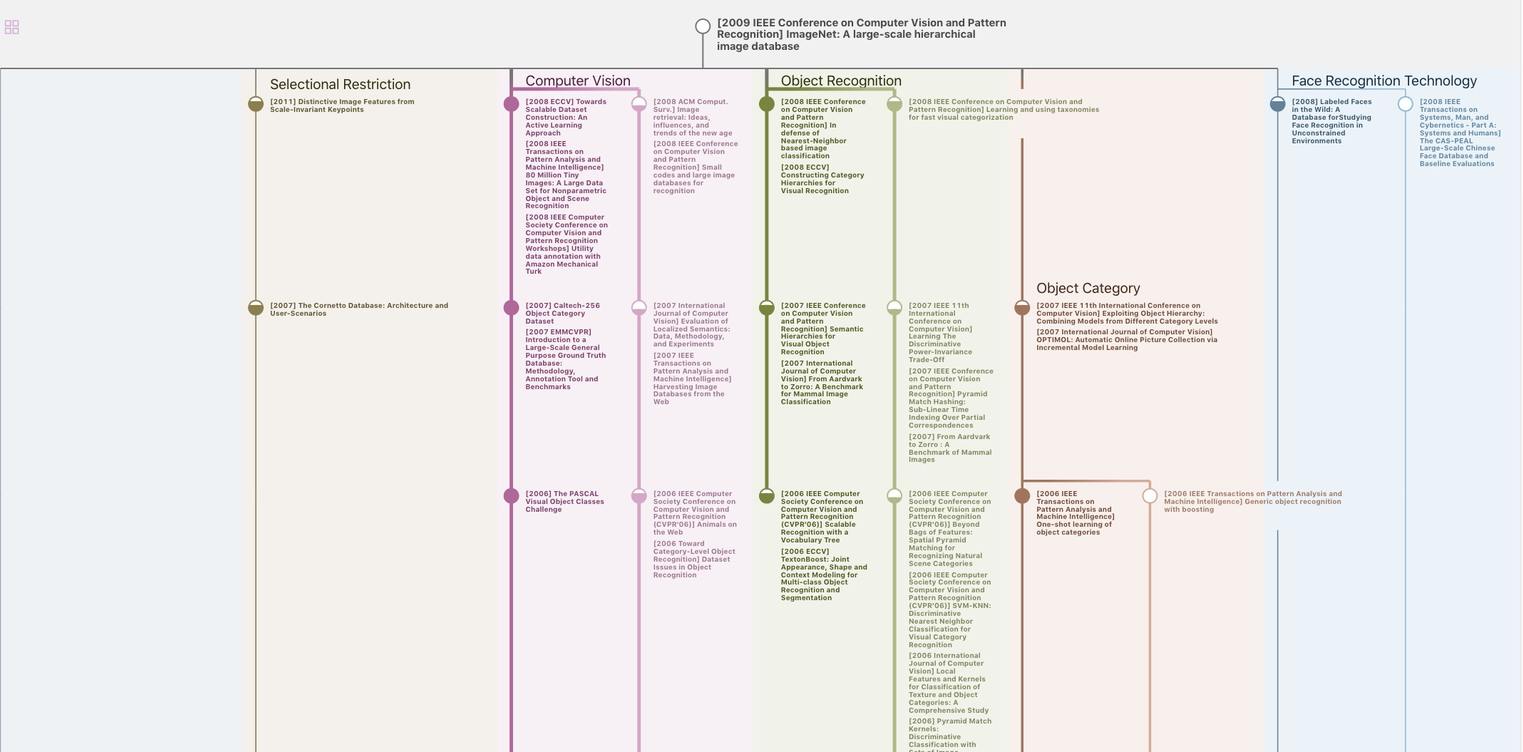
Generate MRT to find the research sequence of this paper
Chat Paper
Summary is being generated by the instructions you defined