MiniAnDE: a reduced AnDE ensemble to deal with microarray data.
EANN(2023)
摘要
This article focuses on the supervised classification of datasets with a
large number of variables and a small number of instances. This is the case,
for example, for microarray data sets commonly used in bioinformatics. Complex
classifiers that require estimating statistics over many variables are not
suitable for this type of data. Probabilistic classifiers with low-order
probability tables, e.g. NB and AODE, are good alternatives for dealing with
this type of data. AODE usually improves NB in accuracy, but suffers from high
spatial complexity since $k$ models, each with $n+1$ variables, are included in
the AODE ensemble. In this paper, we propose MiniAnDE, an algorithm that
includes only a small number of heterogeneous base classifiers in the ensemble,
i.e., each model only includes a different subset of the $k$ predictive
variables. Experimental evaluation shows that using MiniAnDE classifiers on
microarray data is feasible and outperforms NB and other ensembles such as
bagging and random forest.
更多查看译文
关键词
microarray,reduced miniande ensemble,data
AI 理解论文
溯源树
样例
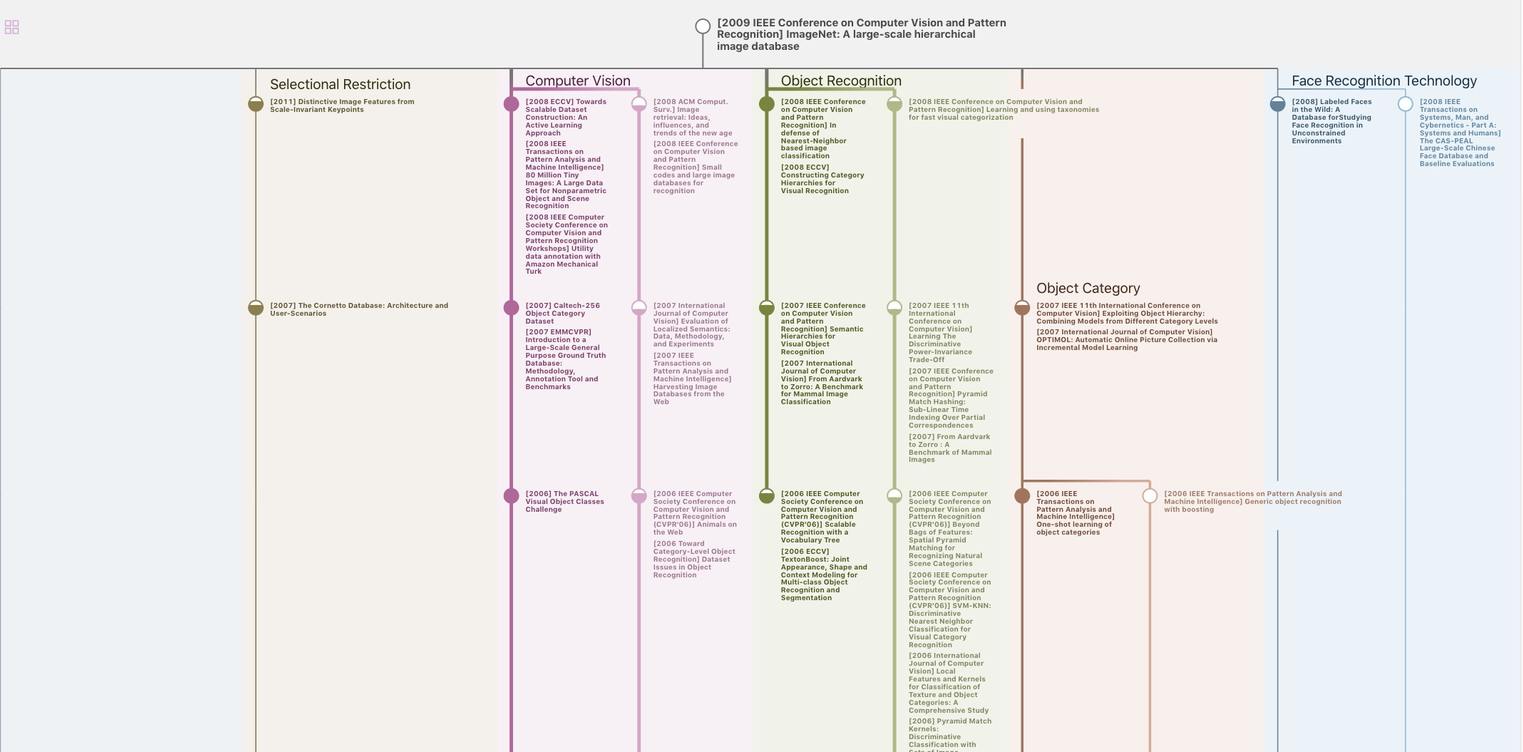
生成溯源树,研究论文发展脉络
Chat Paper
正在生成论文摘要