Effective Information Retrieval, Question Answering and Abstractive Summarization on Large-Scale Biomedical Document Corpora.
NLDB(2023)
Abstract
During the COVID-19 pandemic, a concentrated effort was made to collate published literature on SARS-Cov-2 and other coronaviruses for the benefit of the medical community. One such initiative is the COVID-19 Open Research Dataset which contains over 400,000 published research articles. To expedite access to relevant information sources for health workers and researchers, it is vital to design effective information retrieval and information extraction systems. In this article, an IR approach leveraging transformer-based models to enable question-answering and abstractive summarization is presented. Various keyword-based and neural-network-based models are experimented with and incorporated to reduce the search space and determine relevant sentences from the vast corpus for ranked retrieval. For abstractive summarization, candidate sentences are determined using a combination of various standard scoring metrics. Finally, the summary and the user query are utilized for supporting question answering. The proposed model is evaluated based on standard metrics on the standard CovidQA dataset for both natural language and keyword queries. The proposed approach achieved promising performance for both query classes, while outperforming various unsupervised baselines.
MoreTranslated text
Key words
abstractive summarization,effective information retrieval,question answering,large-scale
AI Read Science
Must-Reading Tree
Example
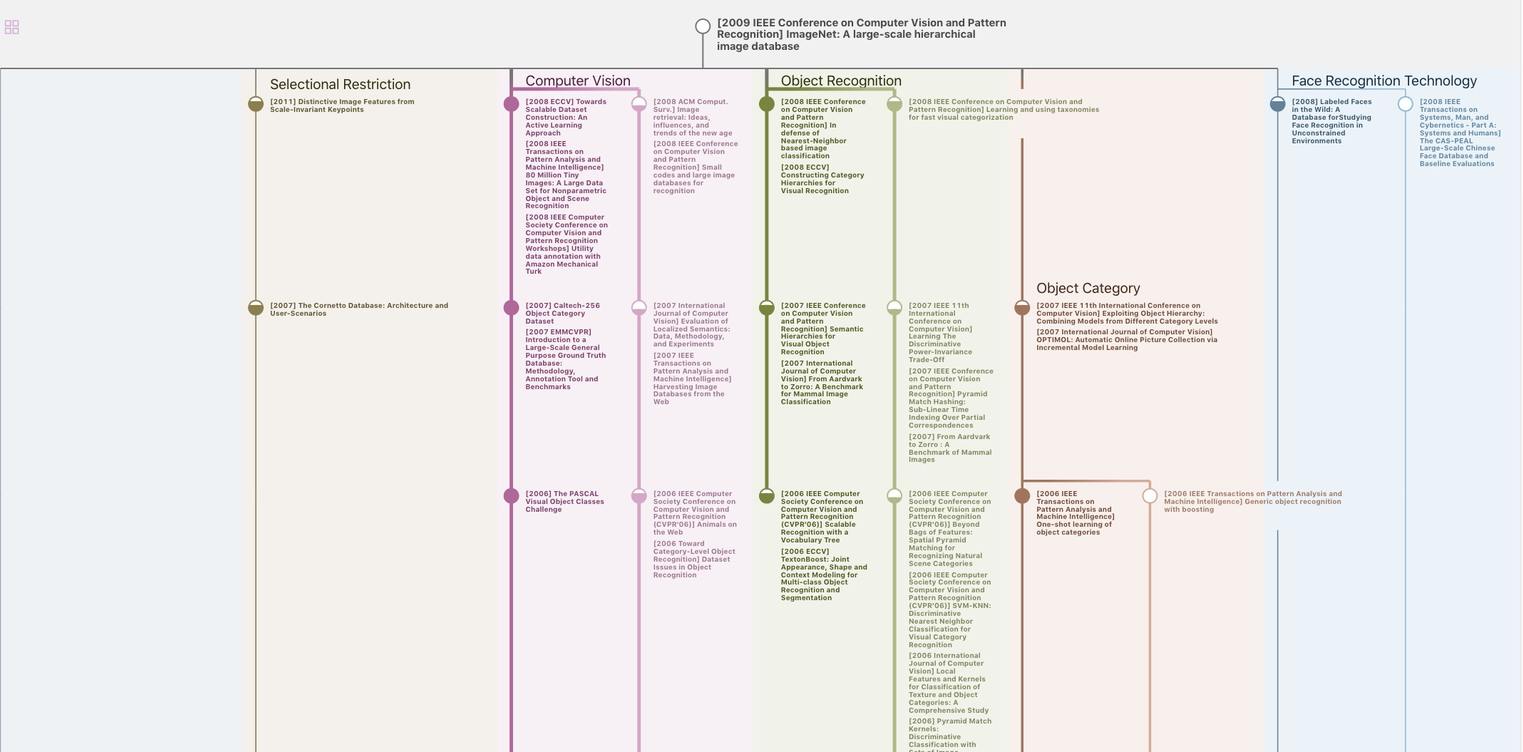
Generate MRT to find the research sequence of this paper
Chat Paper
Summary is being generated by the instructions you defined