EdgePC: Efficient Deep Learning Analytics for Point Clouds on Edge Devices
PROCEEDINGS OF THE 2023 THE 50TH ANNUAL INTERNATIONAL SYMPOSIUM ON COMPUTER ARCHITECTURE, ISCA 2023(2023)
摘要
Recently, point cloud (PC) has gained popularity in modeling various 3D objects (including both synthetic and real-life) and has been extensively utilized in a wide range of applications such as AR/VR, 3D reconstruction, and autonomous driving. For such applications, it is critical to analyze/understand the surrounding scenes properly. To achieve this, deep learning based methods (e.g., convolutional neural networks (CNNs)) have been widely employed for higher accuracy. Unlike the deep learning on conventional 2D images/videos, where the feature computation (matrix multiplication) is the major bottleneck, in point cloud-based CNNs, the sample and neighbor search stages are the primary bottlenecks, and collectively contribute to 54% (up to 80%) of the overall execution latency on a typical edge device. While prior efforts have attempted to solve this issue by designing custom ASICs or pipelining the neighbor search with other stages, to our knowledge, none of them has tried to "structurize" the unstructured PC data for improving computational efficiency. In this paper, we first explore the opportunities of structurizing PC data using Morton code (which is originally designed to map data from a high dimensional space to one dimension, while preserving spatial locality) and observe that there is a huge scope to "skip" the sample and neighbor search computation by operating on the "structurized" PC data. Based on this, we propose two approximation techniques for the sampling and neighbor search stages. We implemented our proposals on an NVIDIA Jetson AGX Xavier edge GPU board. The evaluation results collected on six different workloads show that our design can accelerate the sample and neighbor search stages by 3.68x (up to 5.21x) with minimal impact on inference accuracy. This acceleration in turn results in 1.55x speedup in the end-to-end execution latency and saves 33% of energy expenditure.
更多查看译文
关键词
Point Cloud,Deep Neural Network,Approximation,Energy-efficiency,Edge Device
AI 理解论文
溯源树
样例
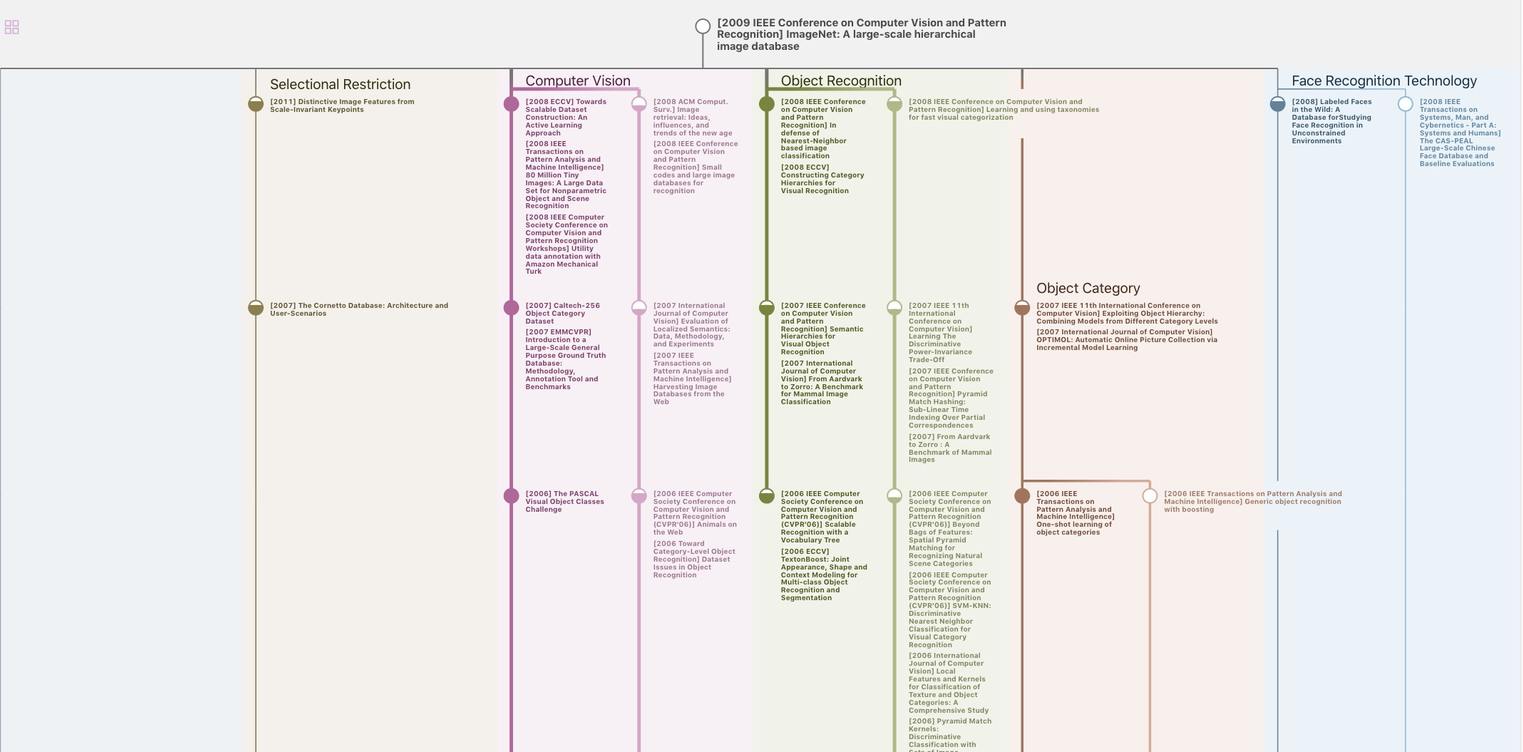
生成溯源树,研究论文发展脉络
Chat Paper
正在生成论文摘要