Architecting Efficient Multi-modal AIoT Systems
PROCEEDINGS OF THE 2023 THE 50TH ANNUAL INTERNATIONAL SYMPOSIUM ON COMPUTER ARCHITECTURE, ISCA 2023(2023)
摘要
Multi-modal computing ((MC)-C-2) has recently exhibited impressive accuracy improvements in numerous autonomous artificial intelligence of things (AIoT) systems. However, this accuracy gain is often tethered to an incredible increase in energy consumption. Particularly, various highly-developed modality sensors devour most of the energy budget, which would make the deployment of (MC)-C-2 for real-world AIoT applications a difficult challenge. To address the above issue, we propose AMG, an innovative HW/SW co-design solution tailored to multi-modal AIoT systems. The key behind AMG is modality gating (throttling) that allows for adaptively sensing and computing modalities for different tasks. This is non-trivial since we must balance situational awareness, energy conservation, and execution latency. AMG achieves our goal with two first-of-its-kind designs. 1) It introduces a novel decoupled modality sensor architecture to support partial throttling of modality sensors. Doing so allows one to greatly save AIoT power but maintains sensor data flow. 2) AMG also features a smart power management strategy based on the new architecture, allowing the device to initialize and tune itself with the optimal configuration. It can predict whether a reasonable degree of accuracy will be satisfied during runtime, and react proactively to remediate the gating process. Extensive evaluation based on our prototype system confirms that AMG improves the AIoT lifespan by 74.5% to 133.7% with the same energy budget while meeting the performance requirements.
更多查看译文
关键词
Edge Artificial Intelligence,Autonomous Embedded Systems,Multimodal Computing,Modality Gating,Energy Efficiency
AI 理解论文
溯源树
样例
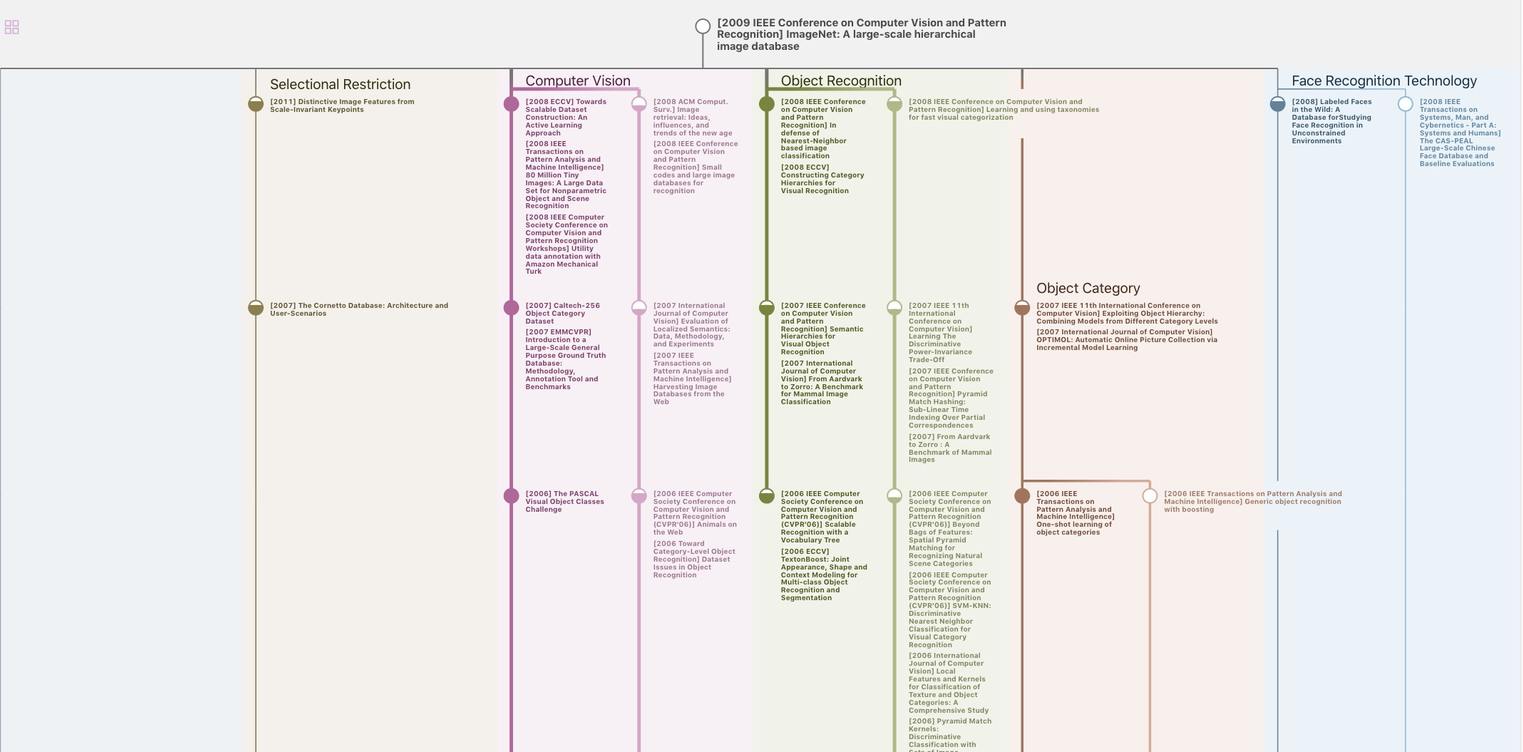
生成溯源树,研究论文发展脉络
Chat Paper
正在生成论文摘要