Mobility network reveals the impact of spatial vaccination heterogeneity on COVID‐19
crossref(2021)
摘要
Abstract Massive vaccination is one of the most effective epidemic control measures. Because one’s vaccination decision is shaped by social processes (e.g., socioeconomic sorting and social contagion), the pattern of vaccine uptake tends to show strong social and spatial heterogeneity, such as urban-rural divide and clustering. Examining through network perspectives, here we quantify the impact of spatial vaccination heterogeneity on COVID outbreaks and offer policy recommendations on location-based vaccination campaigns. Leveraging fine-grained mobility data and computational models, we investigate two network effects—the “hub effect” (hubs in the mobility network usually have higher vaccination rates) and the “homophily effect” (neighboring places tend to have similar vaccination rates). Applying Bayesian deep learning and fine-grained epidemic simulations, we show a negative effect of homophily and a positive effect of highly vaccinated hubs on reducing COVID-19 case counts; these two effects are estimated to jointly increase the total cases by approximately 10% in the U.S. Moreover, inspired by these results, we propose a vaccination campaign strategy that targets a small number of regions with the largest gain in protective power. Our simulation shows that we can reduce the number of cases by 20% by only vaccinating an additional 1% of the population. Our study suggests that we must examine the interplay between vaccination patterns and mobility networks beyond the overall vaccination rate, and that accurate location-based targeting can be equally if not more important than improving the overall vaccination rate.
更多查看译文
AI 理解论文
溯源树
样例
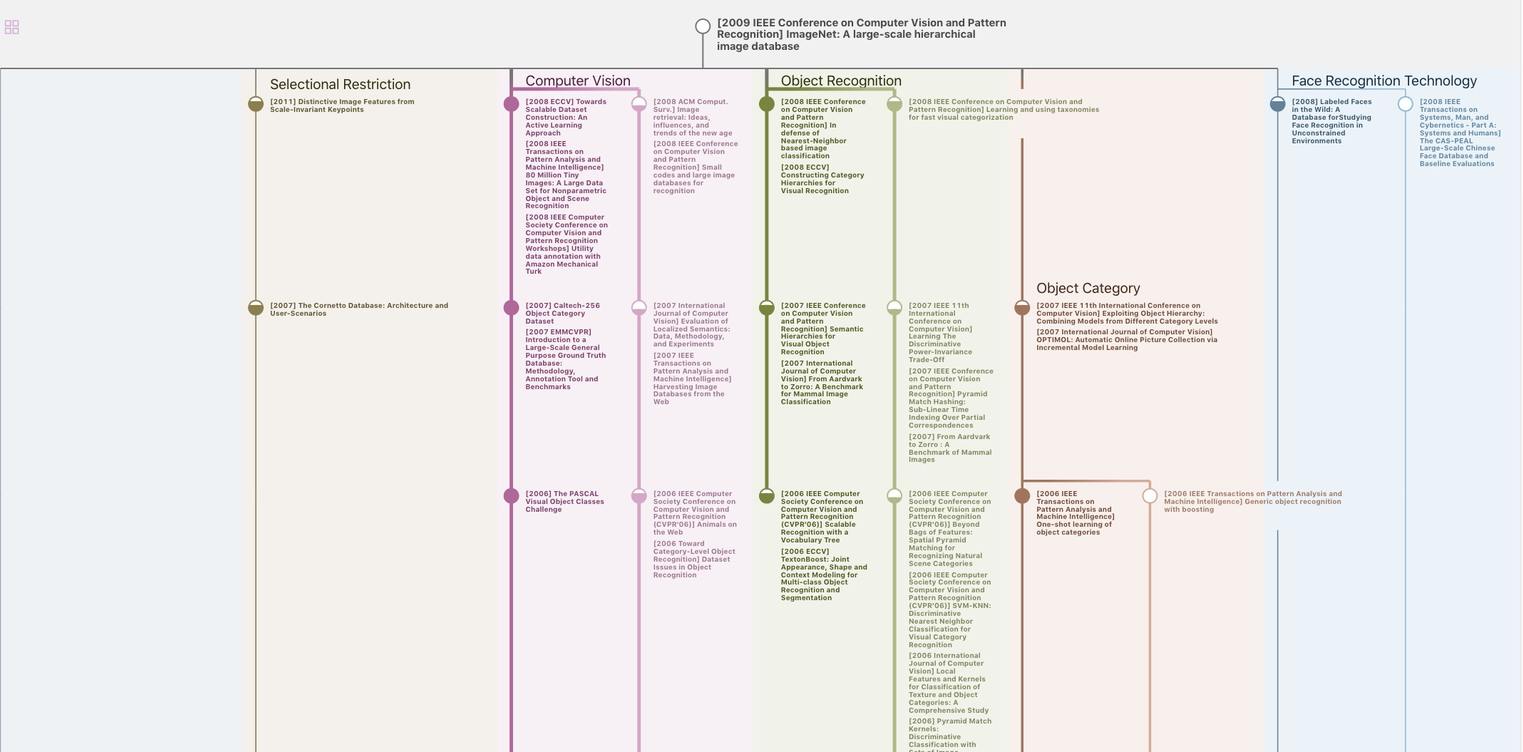
生成溯源树,研究论文发展脉络
Chat Paper
正在生成论文摘要