Weakly-Supervised Multimodal Learning for Predicting the Gender of Twitter Users
Natural Language Processing and Information Systems(2023)
Abstract
Social media platforms, e.g. Twitter, are significant sources of information, with various users posting vast amounts of content every day. Analyzing such content has the potential to offer valuable insights for commercial and research purposes. To gain a comprehensive understanding of the information, it is crucial to consider the demographics of users, with gender being a particularly important factor. Nevertheless, the gender of Twitter’s users is not usually available. Predicting the gender of Twitter’s users from tweet data becomes more challenging. In this paper, we introduce a weakly supervised method to automatically build the supervision data. The experimental result show that our weak supervision component could generate well-annotated data automatically with an accuracy rate exceeding 85%. Furthermore, we conduct a comparative analysis of various multimodal learning architectures to predict the gender of Twitter users using weak supervision data. In the study, five multimodal learning architectures: 1) Early Fusion, 2) Late Fusion, 3) Dense Fusion, 4) Caption Fusion, and 5) Ensemble Fusion, are proposed. The experimental results on the evaluation data indicate that Caption Fusion outperforms the other multimodal learning architectures and baselines.
MoreTranslated text
Key words
Gender Prediction, Multimodal Learning, Deep Learning, Transformer-based Models, Tweet Data
AI Read Science
Must-Reading Tree
Example
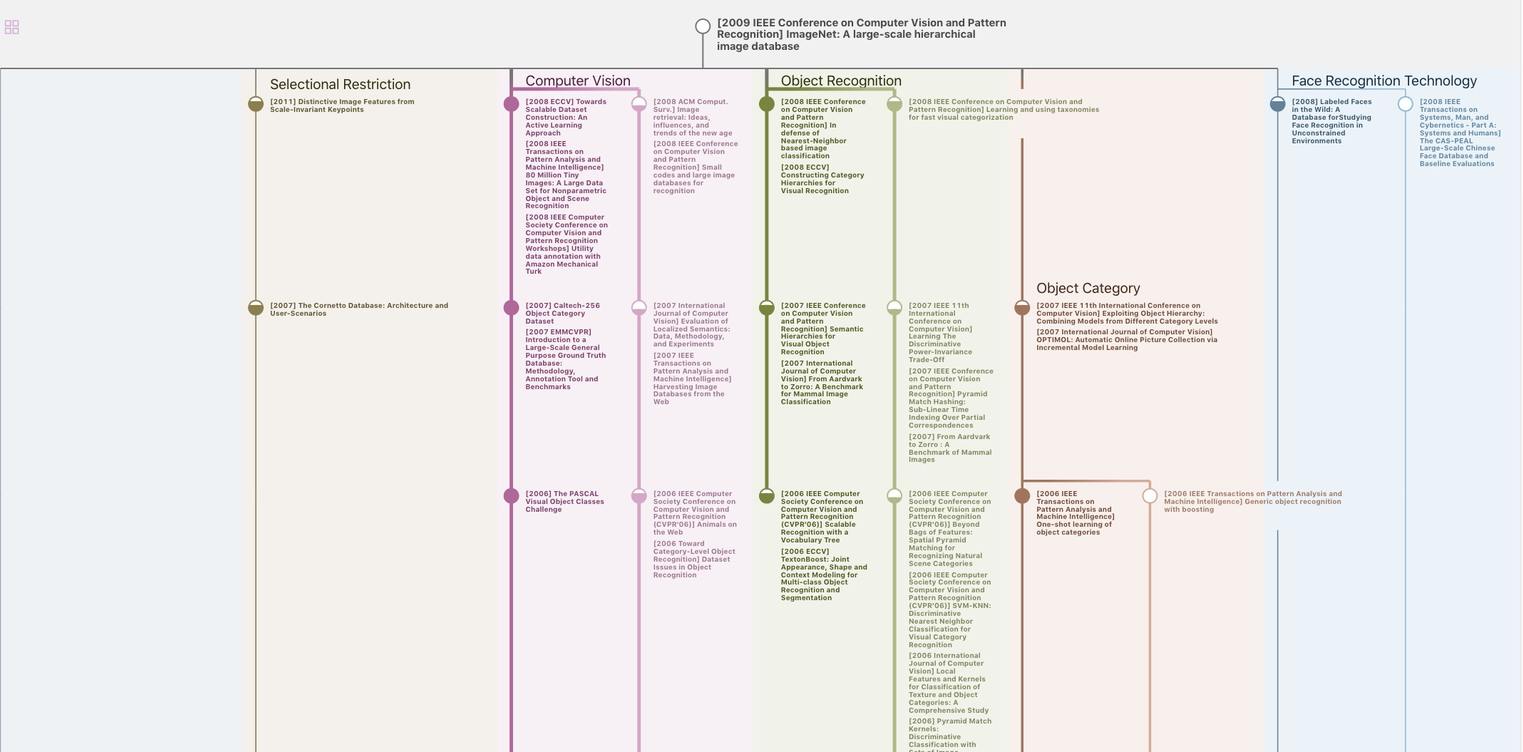
Generate MRT to find the research sequence of this paper
Chat Paper
Summary is being generated by the instructions you defined