Deep Reinforcement Learning for Pin-Point Autonomous Lunar Landing: Trajectory Recalculation for Obstacle Avoidance
The Use of Artificial Intelligence for Space Applications(2023)
摘要
This work aims to present a method to perform autonomous precision landing—pin-point landing—on a planetary environment and perform trajectory recalculation for fault recovery where necessary. In order to achieve this, we choose to implement a Deep Reinforcement Learning—DRL—algorithm, i.e. the Soft Actor-Critic—SAC—architecture. In particular, we select the lunar environment for our experiments, which we perform in a simulated environment, exploiting a real-physics simulator modeled by means of the Bullet/PyBullet physical engine. We show that the SAC algorithm can learn an effective policy for precision landing and trajectory recalculation if fault recovery is made necessary—e.g. for obstacle avoidance.
更多查看译文
关键词
Autonomous landing, Reinforcement learning, Pin-point landing, Fault recovery, Trajectory recalculation
AI 理解论文
溯源树
样例
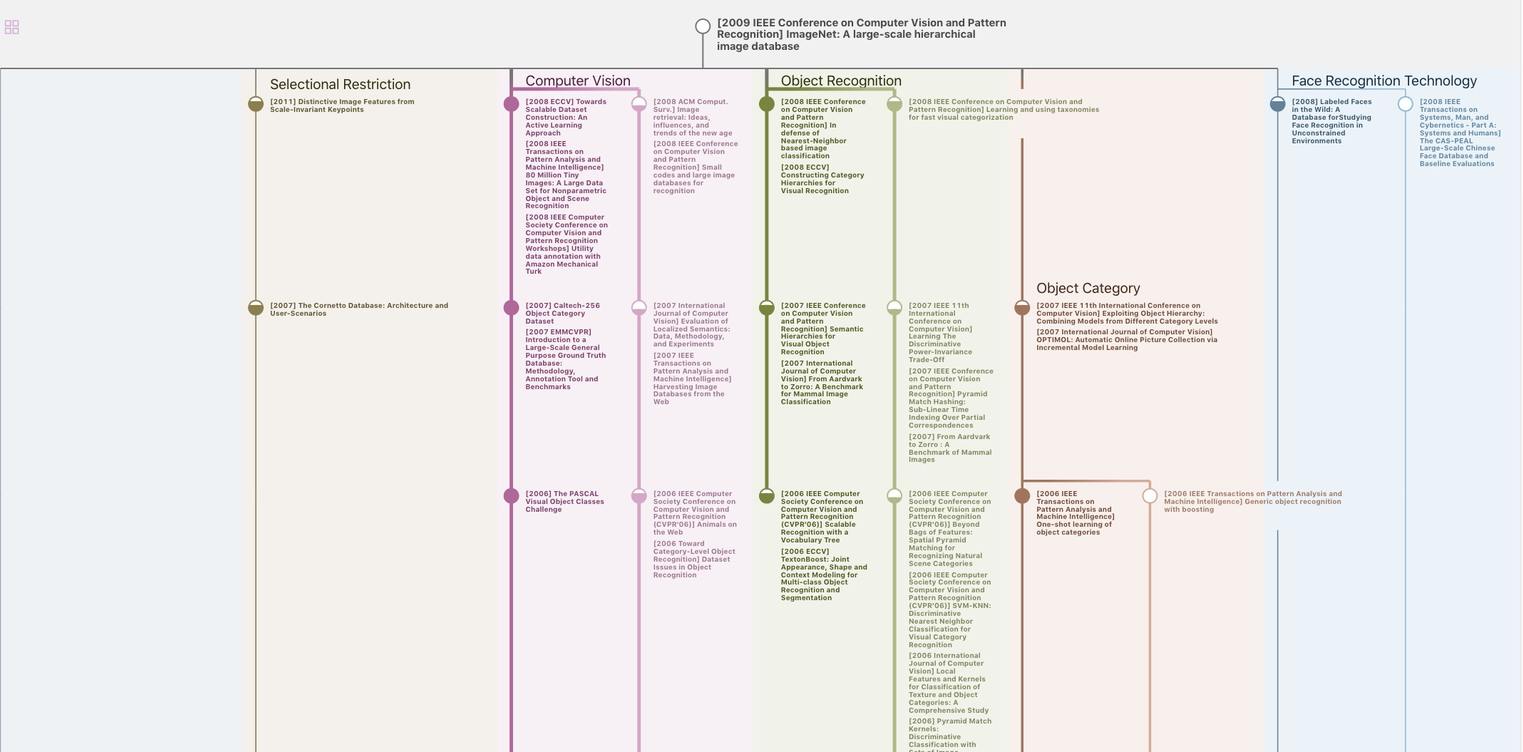
生成溯源树,研究论文发展脉络
Chat Paper
正在生成论文摘要