Argania Forest Change Detection from Sentinel-2 Satellite Images Using U-Net Architectures
International Conference on Advanced Intelligent Systems for Sustainable Development(2023)
摘要
Land Cover Change Detection (LCCD) is an important task from the perspective of regional resource monitoring, land use and environmental planning. One of the major concerns for maintaining biodiversity is deforestation. Meanwhile, the recent advances in the field of image processing by Convolutional Neural Networks (CNNs) and the wide availability of high spatiotemporal resolution satellite imagery provide an unprecedented configuration for the implementation of effective and scalable forest cover change monitoring systems. The objective of this study is to evaluate the performance of two of the most effective deep learning architectures, namely, U-Net and Improved U-Net for the mapping of forest cover to identify deforestation areas between two dates. To this end, two 10m resolution satellite images from the Sentinel-2 satellite covering the Argania forest, an UNESCO Biosphere reserve in the Souss-Massa region in Morocco were used. The implemented methods involve training CNN with two different patch sizes (32–16) in a post-classification framework to compare the classification maps and deduce the change map. The classification accuracy of the two models reached 100% with an advantage to U-Net with an average of Intersection-Over-Union (mIoU) equal to 0.851. The obtained results indicated that Argania forest areas were decreasing over the years, while residential areas and greenhouses were expanding.
更多查看译文
关键词
satellite,forest,detection,u-net
AI 理解论文
溯源树
样例
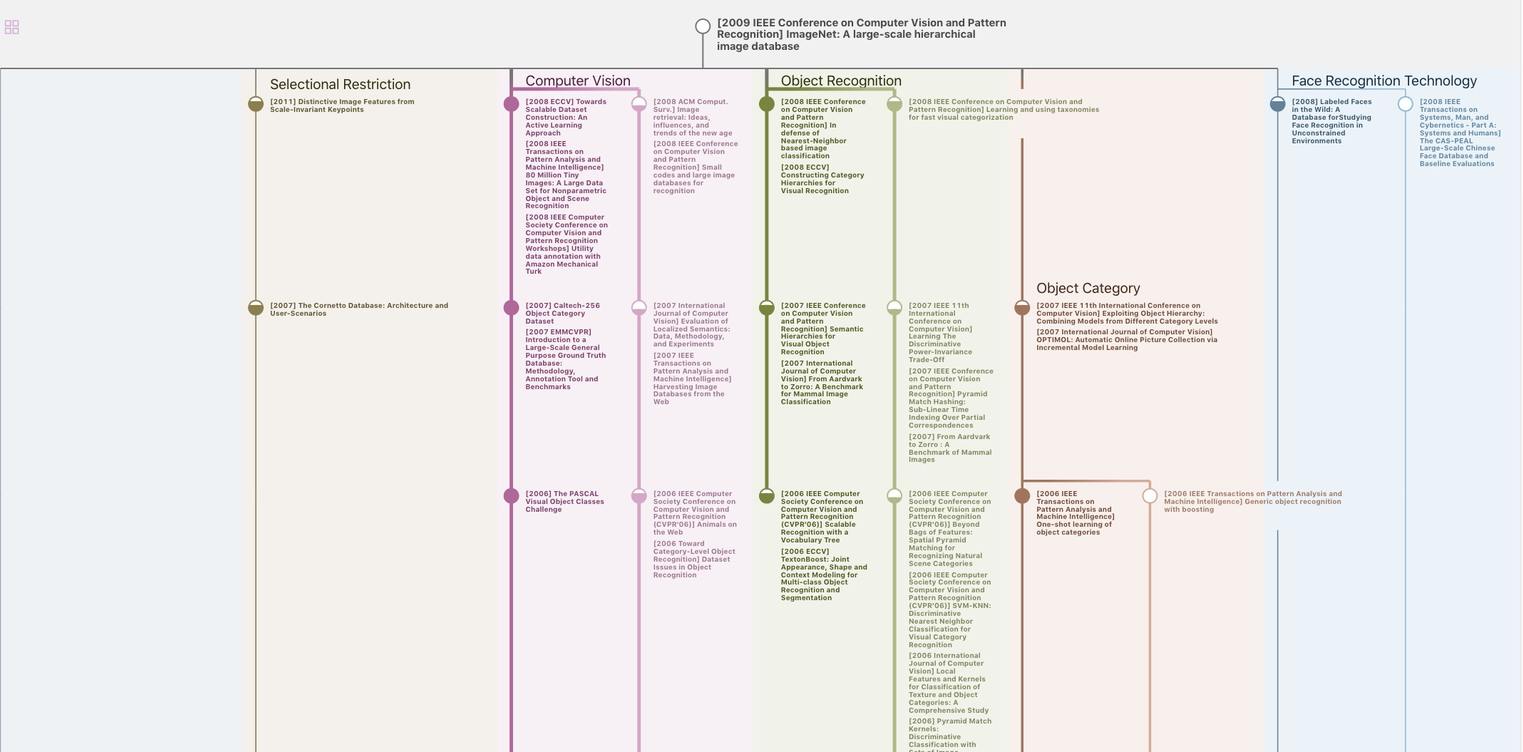
生成溯源树,研究论文发展脉络
Chat Paper
正在生成论文摘要