Multi-task Student Teacher Based Unsupervised Domain Adaptation for Address Parsing.
ADVANCES IN KNOWLEDGE DISCOVERY AND DATA MINING, PAKDD 2023, PT IV(2023)
关键词
Named Entity Recognition,Address parsing,Unsupervised Domain Adaptation
AI 理解论文
溯源树
样例
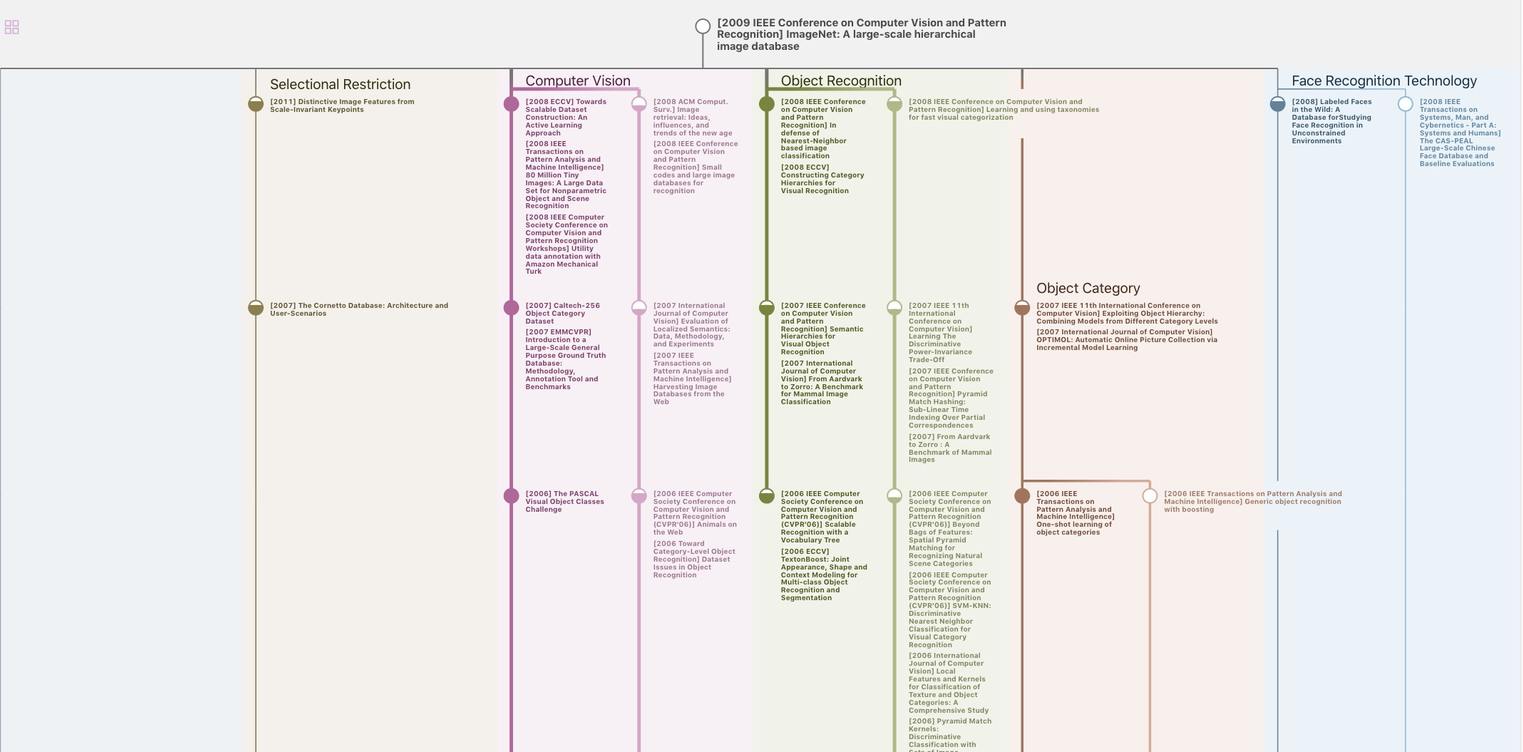
生成溯源树,研究论文发展脉络
Chat Paper
正在生成论文摘要