A Multi-channel Input Framework for Structure Displacement Response Prediction Using Convolutional Neural Network
Building for the Future: Durable, Sustainable, Resilient(2023)
摘要
Accurate prediction of structure’s displacement response is imperative to evaluate structure performance. With the aid of recent advances in deep learning, a data-driven model based on encoder-decoder convolutional neural network (CNN) is proposed to conduct full-field structure displacement response analysis, efficiently and accurately. A multi-channel input framework is developed to transform detailed physical features, including geometry, boundary conditions, and loads, into CNN-learnable data. The boundary conditions and loads are considered variables and mapped by approximation distance functions (ADFs) in the input framework. The proposed model is evaluated on two structures, including the simply supported plate, and wall with a changing opening. In the experiments, the relative errors of typical point displacement predictions are below 0.79%, and 0.70%, respectively. The testing results on walls with changing openings show the root mean square error (RMSE) of full-field displacement predictions is less than 0.072 and the coefficient of determination (R2) is over 0.9992. The results show that the proposed model can make accurate and efficient load-displacement assessments with the multi-channel data framework and avoid laborious repetitive modeling and training, for a class of structures with changing geometry and realistic size.
更多查看译文
关键词
structure displacement response prediction,neural network,multi-channel
AI 理解论文
溯源树
样例
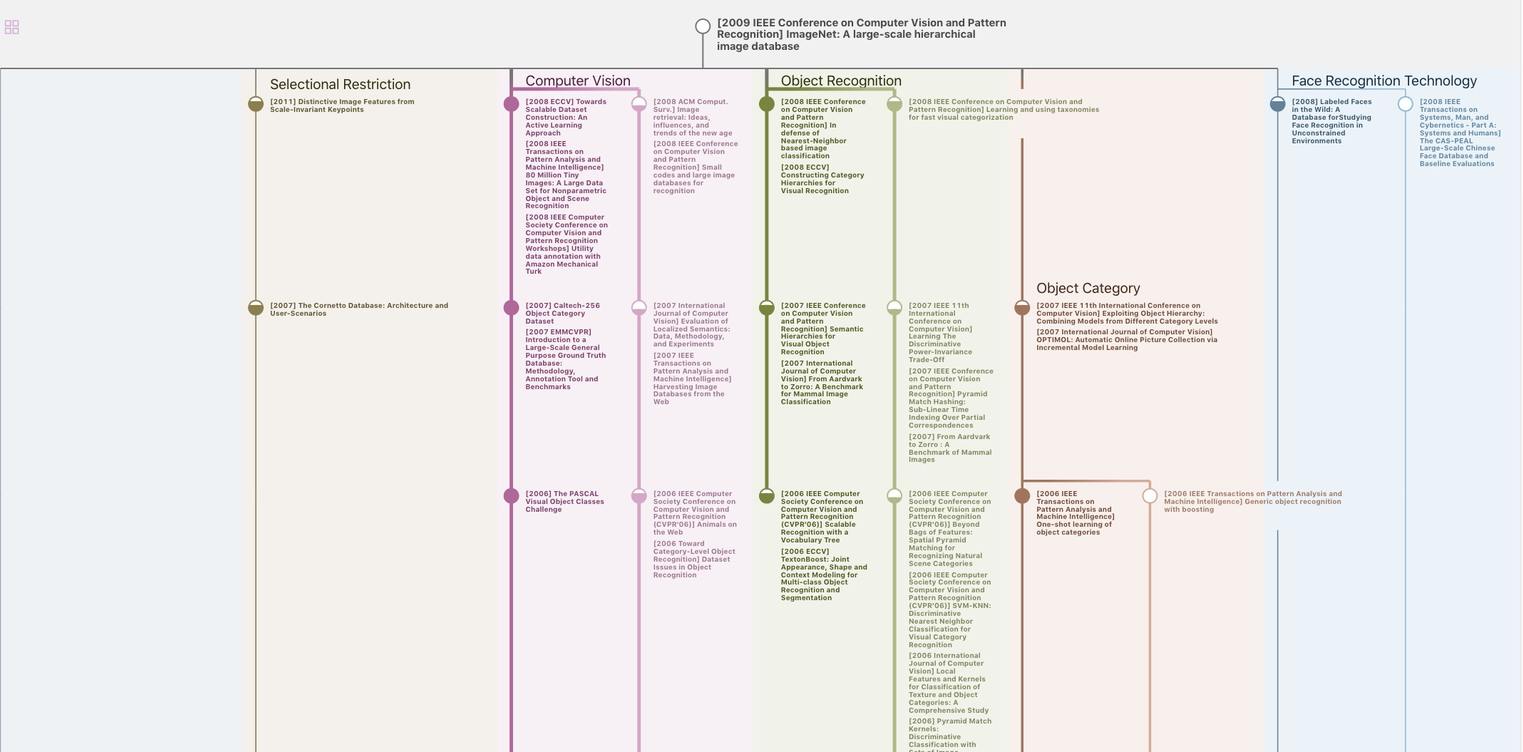
生成溯源树,研究论文发展脉络
Chat Paper
正在生成论文摘要