Bus Journey Time Prediction with Machine Learning: An Empirical Experience in Two Cities
Web and Wireless Geographical Information Systems(2023)
摘要
With increasing urbanisation, and a growing population, transport within cities has never been more important. Buses are the most widespread form of transport worldwide, often being cheaper and more flexible than rail, but also less reliable. Long term bus journey time predictions are important for advanced journey planning and scheduling of bus services. For this reason, several machine/deep learning techniques have been defined to predict bus journey time. Still, due to the number of involved factors, such as complexity and noise in bus data, road network topology, etc., accurate predictions remain elusive. In this paper we aim at validating some Machine Learning methods recently shown to be effective in the literature, on new bus datasets from Dublin and Genoa. The analysis of the results shows some interesting insights into bus networks, highlighting that the accuracy of the predictions is strongly related to the standard deviation of the whole journey times. It emerges that some bus routes show consistency in the prediction error across methods, and for these routes it makes sense to use methods that are fast and computationally efficient, as there is no benefit to applying more complex algorithms. We use features of the route data distribution to develop an explanatory model for the consistency of the route across methods, with a coefficient of determination (
$$R^2$$
) of 0.94. Finally, we identify a systematic anomaly in the data in Dublin that alters the performance of the methods.
更多查看译文
关键词
bus journey time prediction,machine learning,cities
AI 理解论文
溯源树
样例
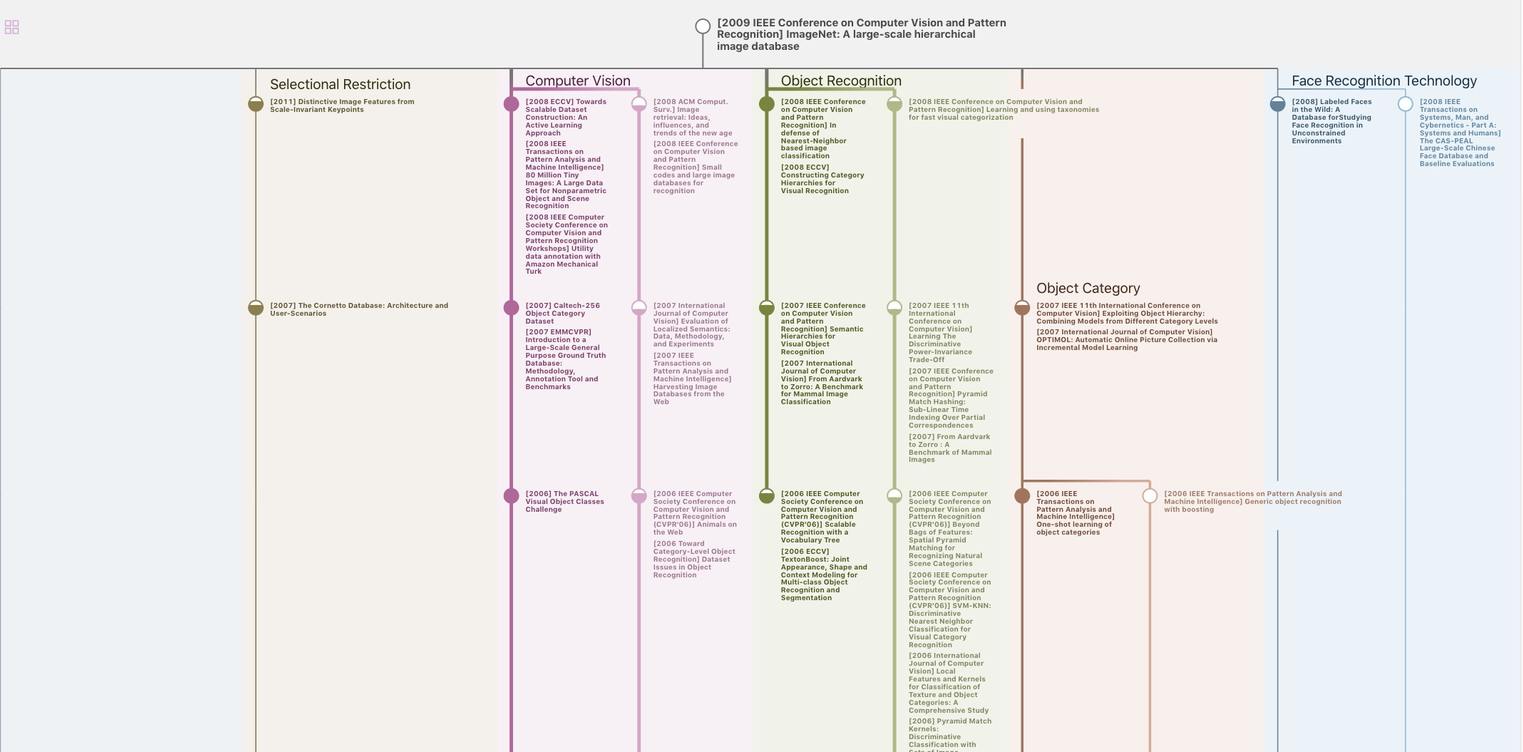
生成溯源树,研究论文发展脉络
Chat Paper
正在生成论文摘要