Mixing Data Augmentation Methods for Semantic Segmentation
Optimization and Learning(2023)
摘要
Deep learning models are the state-of-the-art approach to deal with semantic segmentation tasks. However, training deep models require a considerable amount of images that might be difficult to obtain. This issue can be faced by means of data augmentation techniques that generate new images by applying geometric or colour transformations, or more recently by mixing several images using techniques such as CutMix or CarveMix. Unfortunately, mixing strategies are usually implemented as ad-hoc methods and are difficult to incorporate into the pipeline to train segmentation models. In this work, we present a library that implements several mixing strategies for data augmentation in semantic segmentation tasks. In particular, we provide a set of callbacks that can be integrated into the training pipeline of FastAI segmentation models. We have tested the library with a vineyard dataset and show the benefits of combining mixing strategies with traditional data augmentation techniques; namely an improvement of almost 5% was achieved using these methods regarding models trained only with traditional data augmentation methods.
更多查看译文
AI 理解论文
溯源树
样例
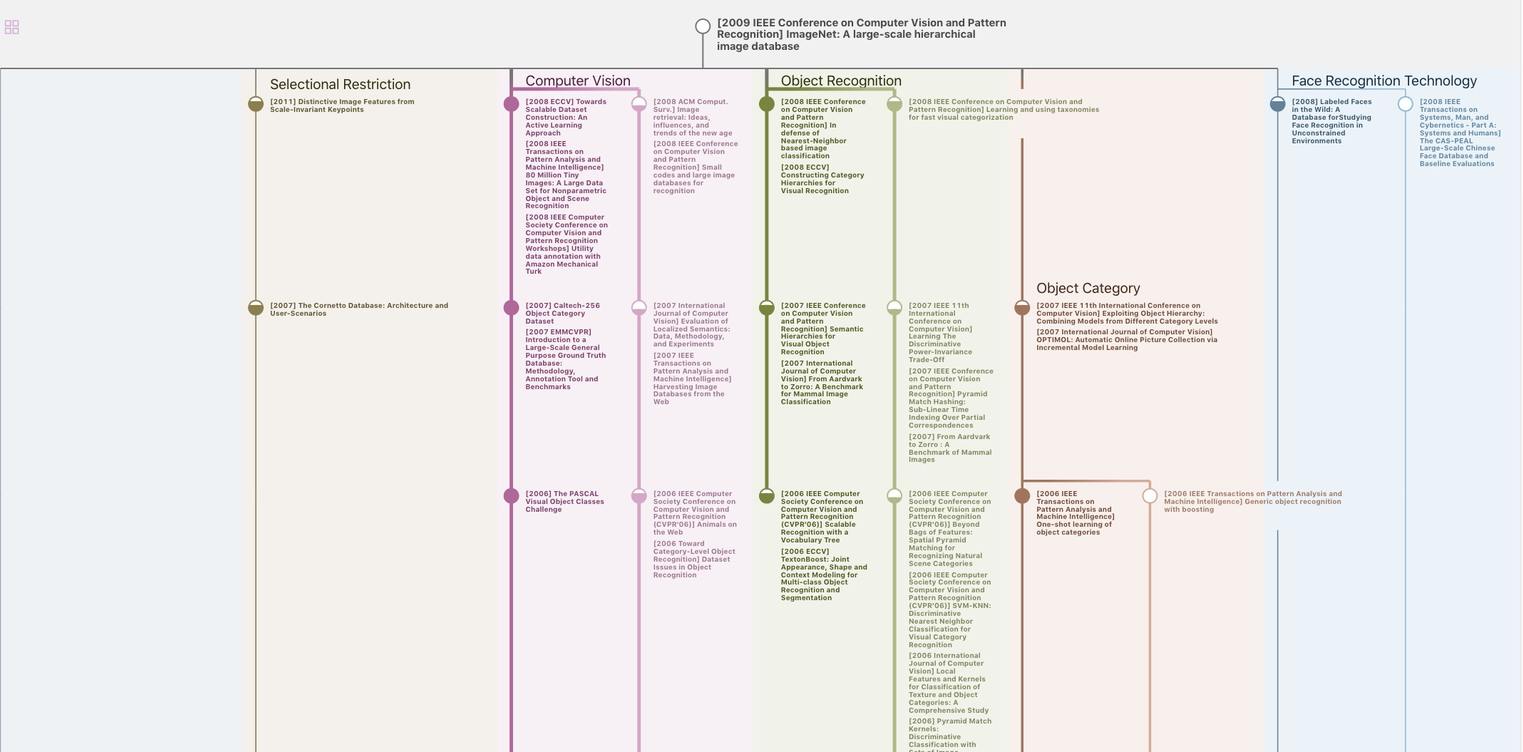
生成溯源树,研究论文发展脉络
Chat Paper
正在生成论文摘要