Beyond Neural-on-Neural Approaches to Speaker Gender Protection
CoRR(2023)
摘要
Recent research has proposed approaches that modify speech to defend against gender inference attacks. The goal of these protection algorithms is to control the availability of information about a speaker's gender, a privacy-sensitive attribute. Currently, the common practice for developing and testing gender protection algorithms is "neural-on-neural", i.e., perturbations are generated and tested with a neural network. In this paper, we propose to go beyond this practice to strengthen the study of gender protection. First, we demonstrate the importance of testing gender inference attacks that are based on speech features historically developed by speech scientists, alongside the conventionally used neural classifiers. Next, we argue that researchers should use speech features to gain insight into how protective modifications change the speech signal. Finally, we point out that gender-protection algorithms should be compared with novel "vocal adversaries", human-executed voice adaptations, in order to improve interpretability and enable before-the-mic protection.
更多查看译文
关键词
adversarial speech,gender inference,neural classifiers,interpretablity,attribute inference
AI 理解论文
溯源树
样例
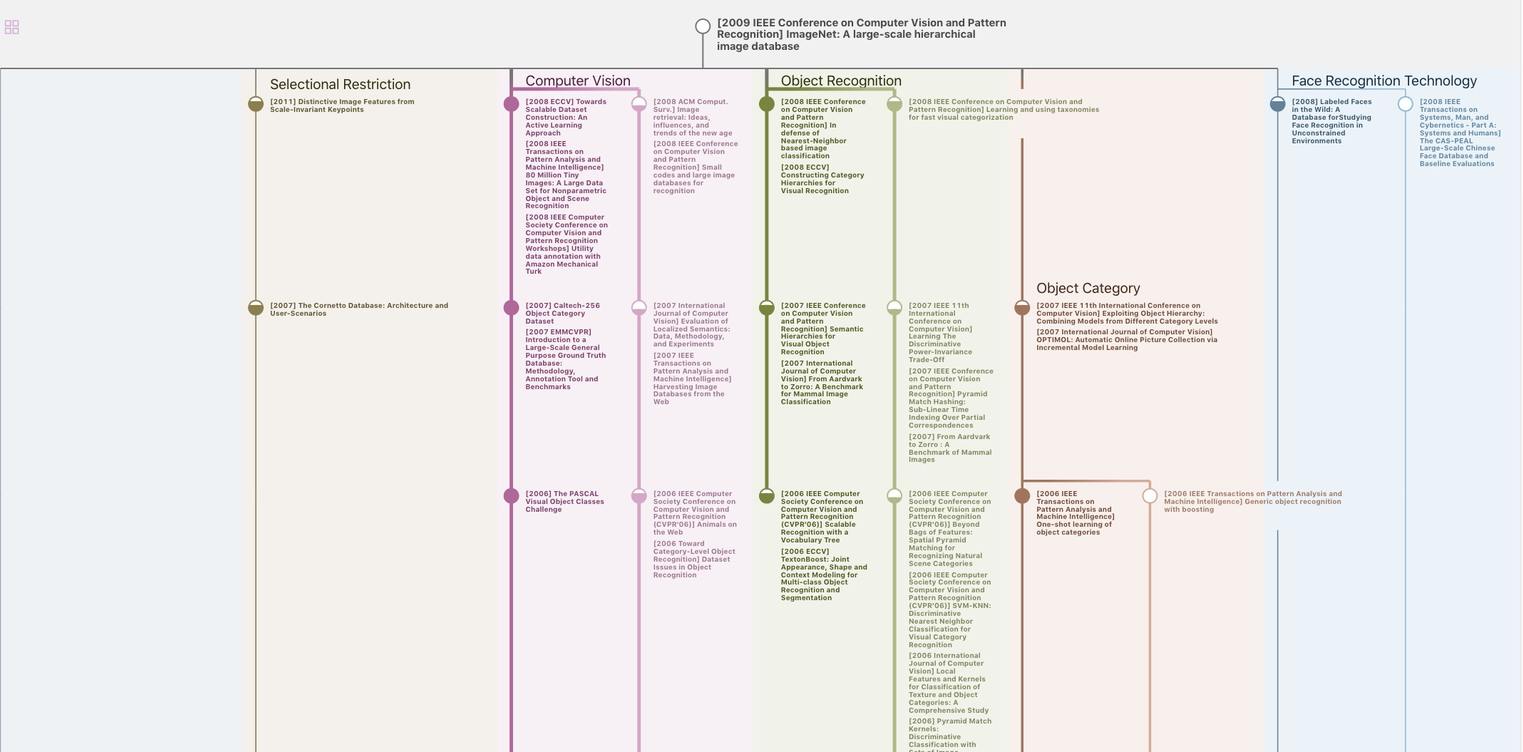
生成溯源树,研究论文发展脉络
Chat Paper
正在生成论文摘要