Zero-Shot Nuclei Detection via Visual-Language Pre-trained Models
MEDICAL IMAGE COMPUTING AND COMPUTER ASSISTED INTERVENTION, MICCAI 2023, PT VI(2023)
摘要
Large-scale visual-language pre-trained models (VLPM) have proven their excellent performance in downstream object detection for natural scenes. However, zero-shot nuclei detection on H&E images via VLPMs remains underexplored. The large gap between medical images and the web-originated text-image pairs used for pre-training makes it a challenging task. In this paper, we attempt to explore the potential of the object-level VLPM, Grounded Language-Image Pre-training (GLIP) model, for zero-shot nuclei detection. Concretely, an automatic prompts design pipeline is devised based on the association binding trait of VLPM and the image-to-text VLPM BLIP, avoiding empirical manual prompts engineering. We further establish a self-training framework, using the automatically designed prompts to generate the preliminary results as pseudo labels from GLIP and refine the predicted boxes in an iterative manner. Our method achieves a remarkable performance for label-free nuclei detection, surpassing other comparison methods. Foremost, our work demonstrates that the VLPM pretrained on natural image-text pairs exhibits astonishing potential for downstream tasks in the medical field as well. Code will be released at github.com/VLPMNuD.
更多查看译文
关键词
Nuclei Detection,Unsupervised Learning,Visual-Language Pre-trained Models,Prompt Designing,Zero-shot Learning
AI 理解论文
溯源树
样例
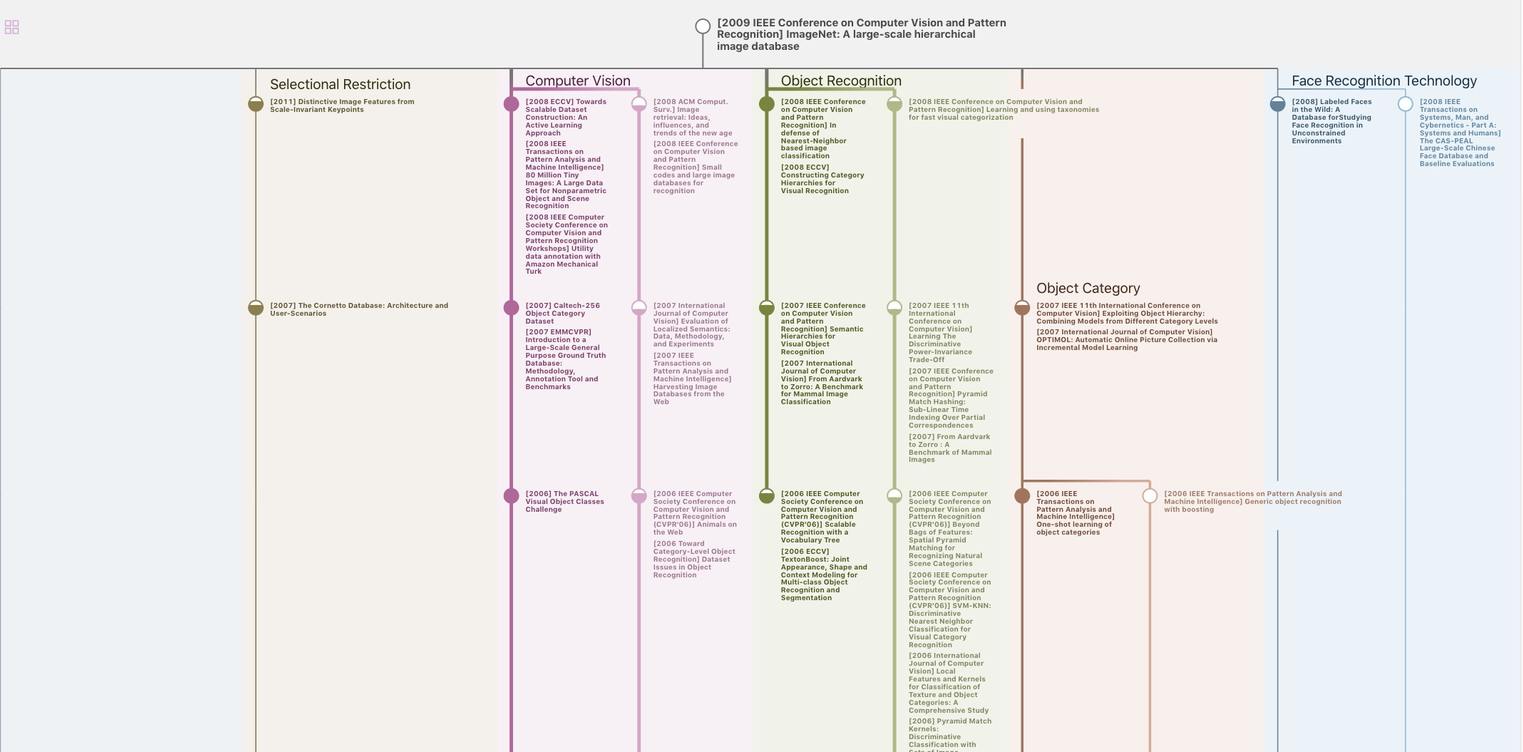
生成溯源树,研究论文发展脉络
Chat Paper
正在生成论文摘要