ReLU Neural Networks, Polyhedral Decompositions, and Persistent Homolog
CoRR(2023)
摘要
A ReLU neural network leads to a finite polyhedral decomposition of input space and a corresponding finite dual graph. We show that while this dual graph is a coarse quantization of input space, it is sufficiently robust that it can be combined with persistent homology to detect homological signals of manifolds in the input space from samples. This property holds for a variety of networks trained for a wide range of purposes that have nothing to do with this topological application. We found this feature to be surprising and interesting; we hope it will also be useful.
更多查看译文
关键词
persistent homolog,polyhedral decompositions,neural networks
AI 理解论文
溯源树
样例
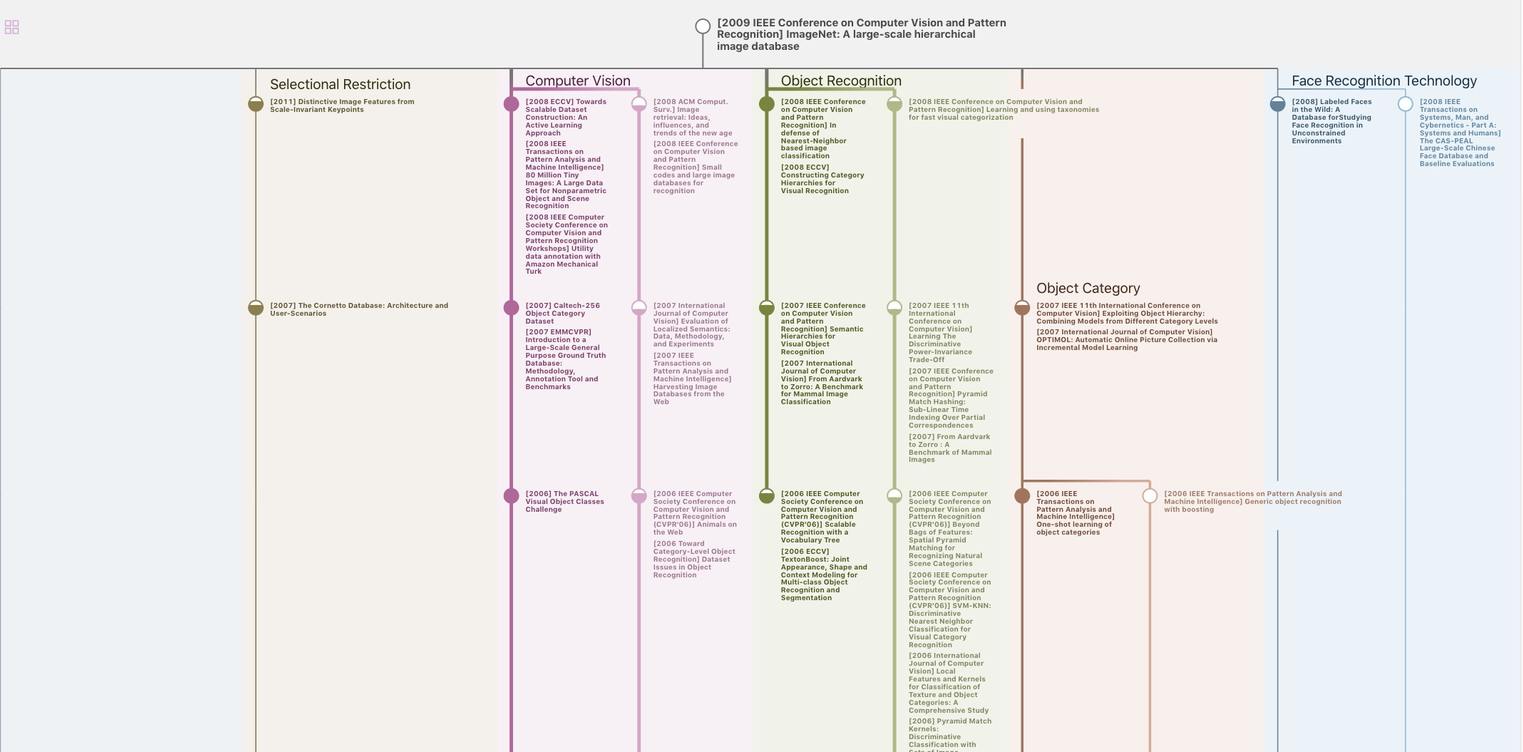
生成溯源树,研究论文发展脉络
Chat Paper
正在生成论文摘要