Single-cell Bayesian deconvolution of flow cytometry data
crossref(2022)
摘要
Flow cytometry enables monitoring protein abundance and activity at the single-cell level in a high-throughput manner, through the use of fluorescent labeling. Given the significant levels of autofluorescence emitted by cells at the spectral ranges used by this technique, removing the corresponding background signal is necessary for a correct assessment of cellular biochemistry. Existing methods for removing autofluorescence usually require dedicated resources, such as additional fluorescence channels or laser sources, which are costly and not universally accessible. Here, we have developed a computational method that enables autofluorescence subtraction without requiring dedicated measurement resources. The method uses a non-parametric Bayesian approach to deconvolve the target signal distribution from independent measurements of labeled and unlabeled cells readily available in a typical experiment. The distributions are approximated by mixtures of gamma functions, and the target distribution is obtained by sampling the posterior distribution using a Markov chain Monte Carlo approach. We tested the method systematically using synthetic data, and validated it using experimental data from mouse embryonic stem cells.
更多查看译文
AI 理解论文
溯源树
样例
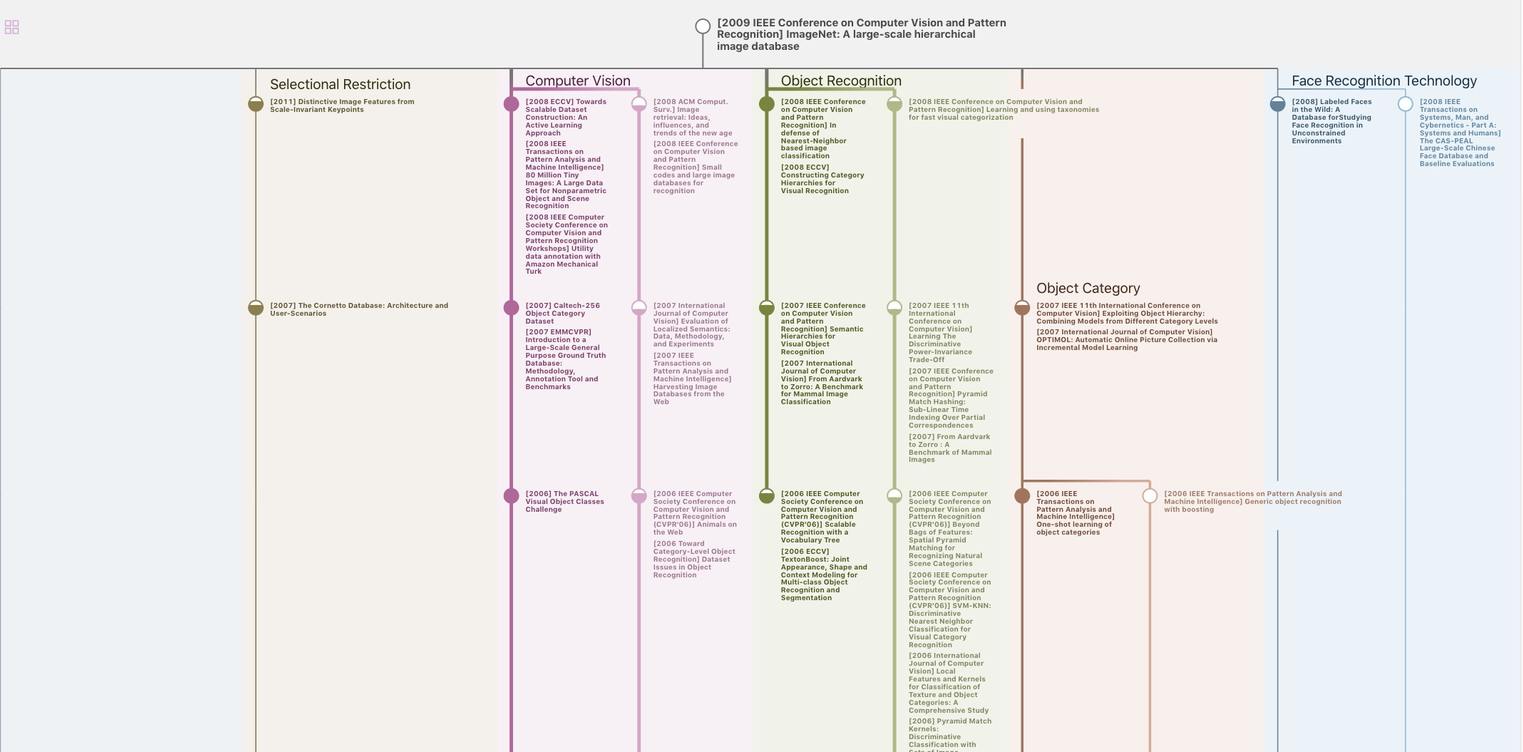
生成溯源树,研究论文发展脉络
Chat Paper
正在生成论文摘要