An empirical comparison between polygenic risk scores and machine learning for case/control classification
Research Square (Research Square)(2022)
摘要
Abstract BackgroundWe compared the procedure to calculate polygenic risk scores and machine learning for simulated data, devised a way to compare machine learning results with PRS, and highlighted the required files formats for PRS calculation and machine learning model training. For PRS calculation, we used three tools: Plink, PRSice, and Lassosum, and for the machine learning algorithm, we used artificial neural networks. ResultsBased on our survey, we cannot say machine learning is better or polygenic risk scores because it depends on the phenotype under consideration. The average classification AUC of PRSice, Plink, Lassosum, and Machine learning was 0.27, 0.3, 0.35, and 0.87 on simulated data. ConclusionThis article presents the comparison method in an automated way, ultimately assisting in various analyses. For instance, datasets with different heritability or genetic variations can be generated, and the effect on machine learning algorithms' accuracy and PRS's accuracy can be studied. Such analyses may require the generation of multiple datasets, calculation of PRS, and training machine learning model, which can be done quickly using the code segments and scripts provided in this manuscript. Apart from that, we compared the steps of PRS calculation with machine learning and found some steps are optional in machine learning.
更多查看译文
关键词
polygenic risk scores,machine learning,case/control classification
AI 理解论文
溯源树
样例
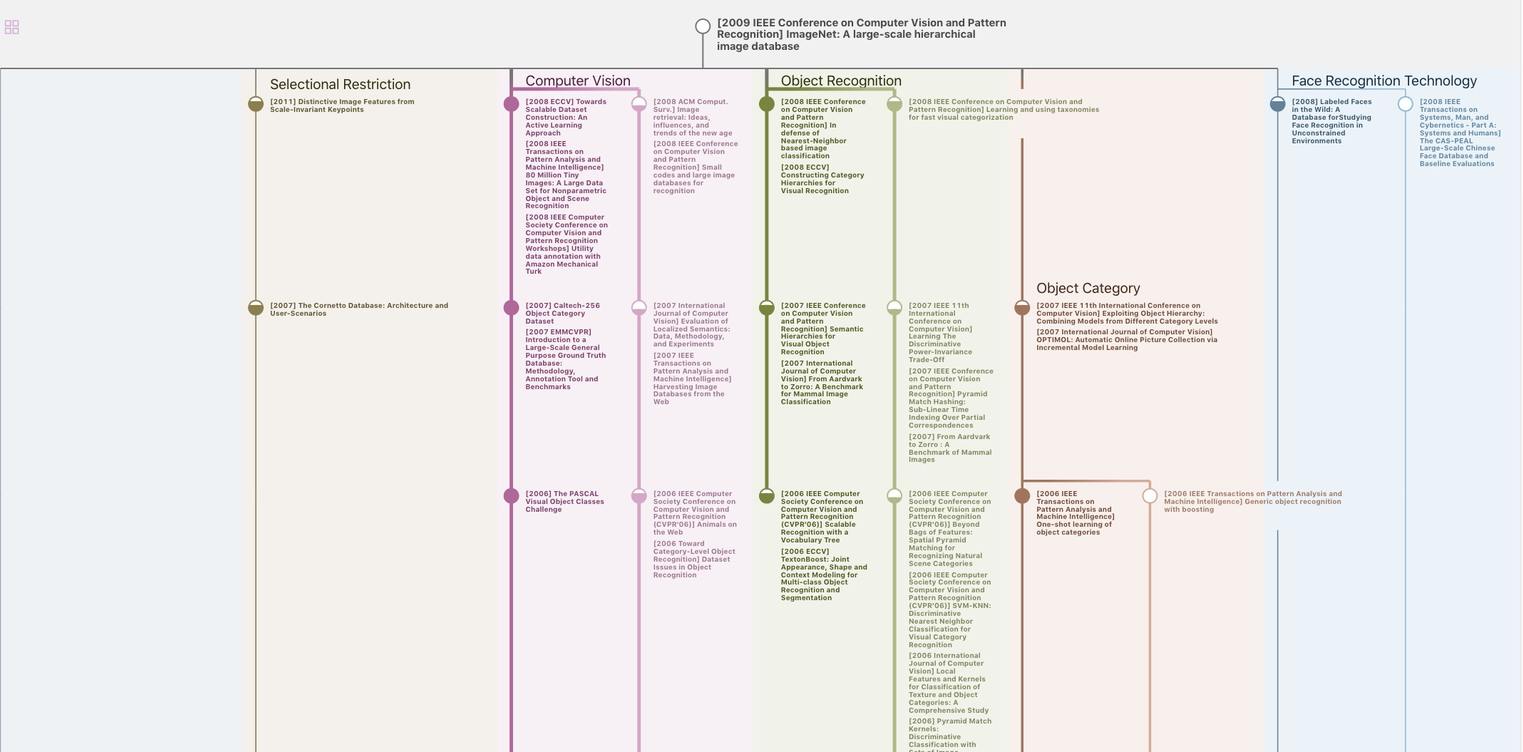
生成溯源树,研究论文发展脉络
Chat Paper
正在生成论文摘要