Drug-Target Graph based Recurrent Network for Drug Combination Prediction
Research Square (Research Square)(2022)
摘要
Abstract Compared with monotherapy, drug combination therapy has demonstrated more effective and powerful therapeutic effects in cancer treatment. However, due to the large size of the combinatorial space, experimental identification of synergistic drug combinations is cost- and time-consuming, even with high-throughput screens. With the accumulation of various types of drug informatics data, artificial intelligence techniques have become applicable for the prediction of synergistic drug combinations, thereby reducing the experimental workload and accelerating the discovery of combination therapy. Currently, the emerging graph neural network (GNN) has been applied to drug combination prediction and brings new insight on the discovery of combination therapy. In this study, we proposed Drug-Target Graph based Recurrent Network (DTGRN) to predict synergistic drug combinations in various cancer cell lines. Specifically, we proposed a Graph Recurrent Network-based encoder to learn a low-dimensional representation of features for each node and then predicted the synergy score of novel drug combinations based on these representations via a cell line-specific projection matrix. In DTGRN, gating mechanism and cell-line-level states are employed to ensure each node representation more comprehensively absorbs local and global information and potential association information. In the application of the cell line-specific drug-target heterogeneous graph, DTGRN significantly outperforms the state-of-the-arts and several classical machine learning algorithms. More prominently, the novel drug combinations predicted by DTGRN have been validated by computational analysis and in vitro experiments, confirming the high predictive performance of DTGRN. These drug combinations could be further evaluated as potential combination therapy strategy. In summary, these results indicate that DTGRN can serve as a powerful tool for integrating heterogeneous graph information to predict drug combinations and provide a promising way to discover novel synergistic drug combination for cancer treatment.
更多查看译文
关键词
recurrent network,prediction,combination,drug-target
AI 理解论文
溯源树
样例
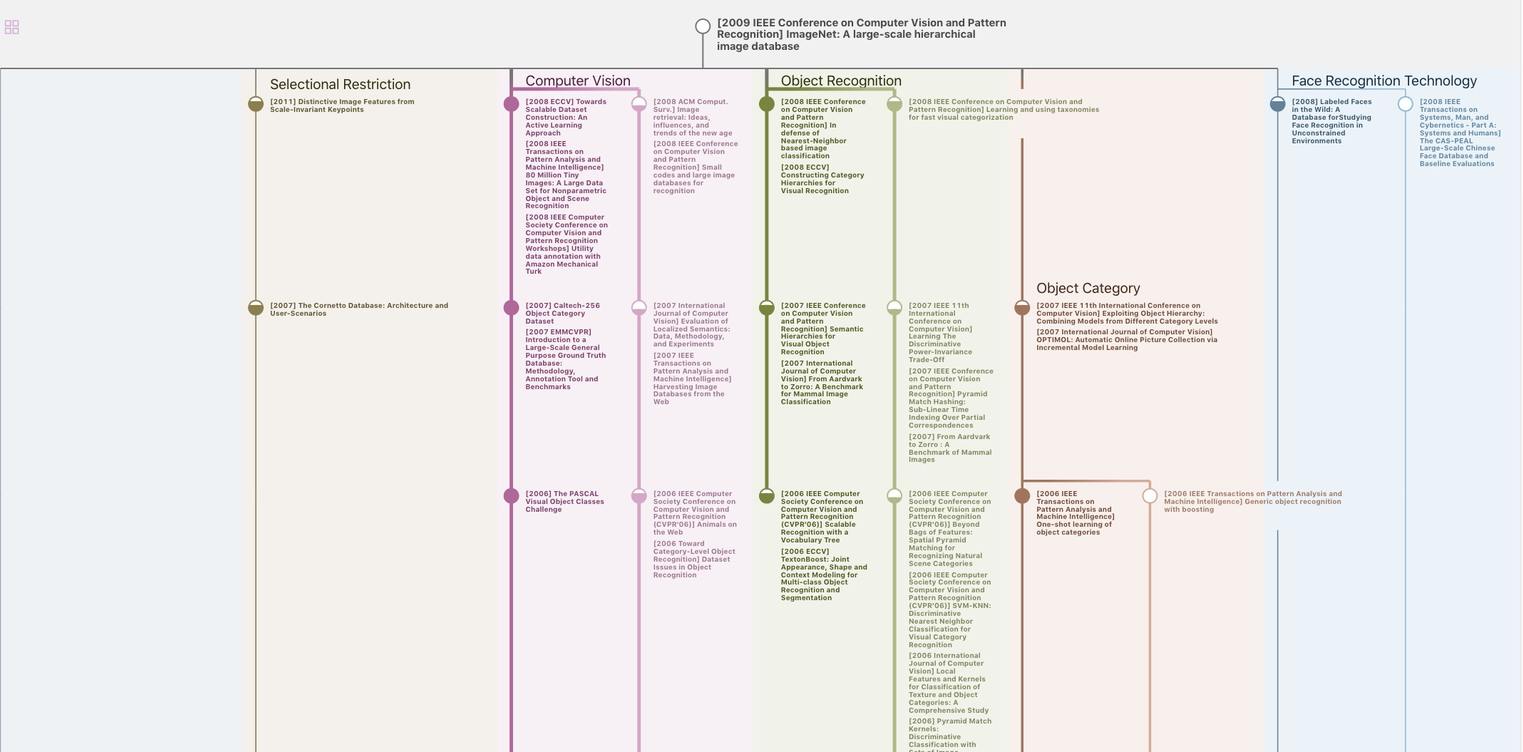
生成溯源树,研究论文发展脉络
Chat Paper
正在生成论文摘要