VAE4OBS: Denoising ocean bottom seismograms using variational autoencoders
crossref(2022)
Abstract
<p>Data from ocean-bottom seismometers (OBS) are inherently more challenging than their land counterpart because of their noisy environment. Primary and secondary microseismic noises corrupt the recorded time series. Additionally, anthropogenic (e.g., ships) and animal noise (e.g., Whales) contribute to a complex noise that can make it challenging to use traditional filtering methods (e.g., broadband or Gabor filters) to clean and extract information from these seismograms. <br><br>OBS deployments are laborious, expensive, and time-consuming. The data of these deployments are crucial in investigating and covering the "blind spots" where there is a lack of station coverage. It, therefore, becomes vital to remove the noise and retrieve earthquake signals recorded on these seismograms.<br><br>We propose analysing and processing such unique and challenging data with Machine Learning (ML), particularly Deep Learning (DL) techniques, where conventional methods fail. We present a variational autoencoder (VAE) architecture to denoise seismic waveforms with the aim to extract more information than previously possible. We argue that, compared to other fields, seismology is well-posed to use ML and DL techniques thanks to massive datasets recorded by seismograms. <br><br>In the first step, we use synthetic seismograms (generated with Instaseis) and white noise to train a deep neural network. We vary the signal-to-noise ratio during training. Such synthetic datasets have two advantages. First, we know the signal and noise (as we have injected the noise ourselves). Second, we can generate large training and validation datasets, one of the prerequisites for high-quality DL models.<br><br>Next, we increased the complexity of input data by adding real noise sampled from land and OBS to the synthetic seismograms. Finally, we apply the trained model to real OBS data recorded during the RHUM-RUM experiment.<br><br>We present the workflow, the neural network architecture, our training strategy, and the usefulness of our trained models compared to traditional methods.</p>
MoreTranslated text
AI Read Science
Must-Reading Tree
Example
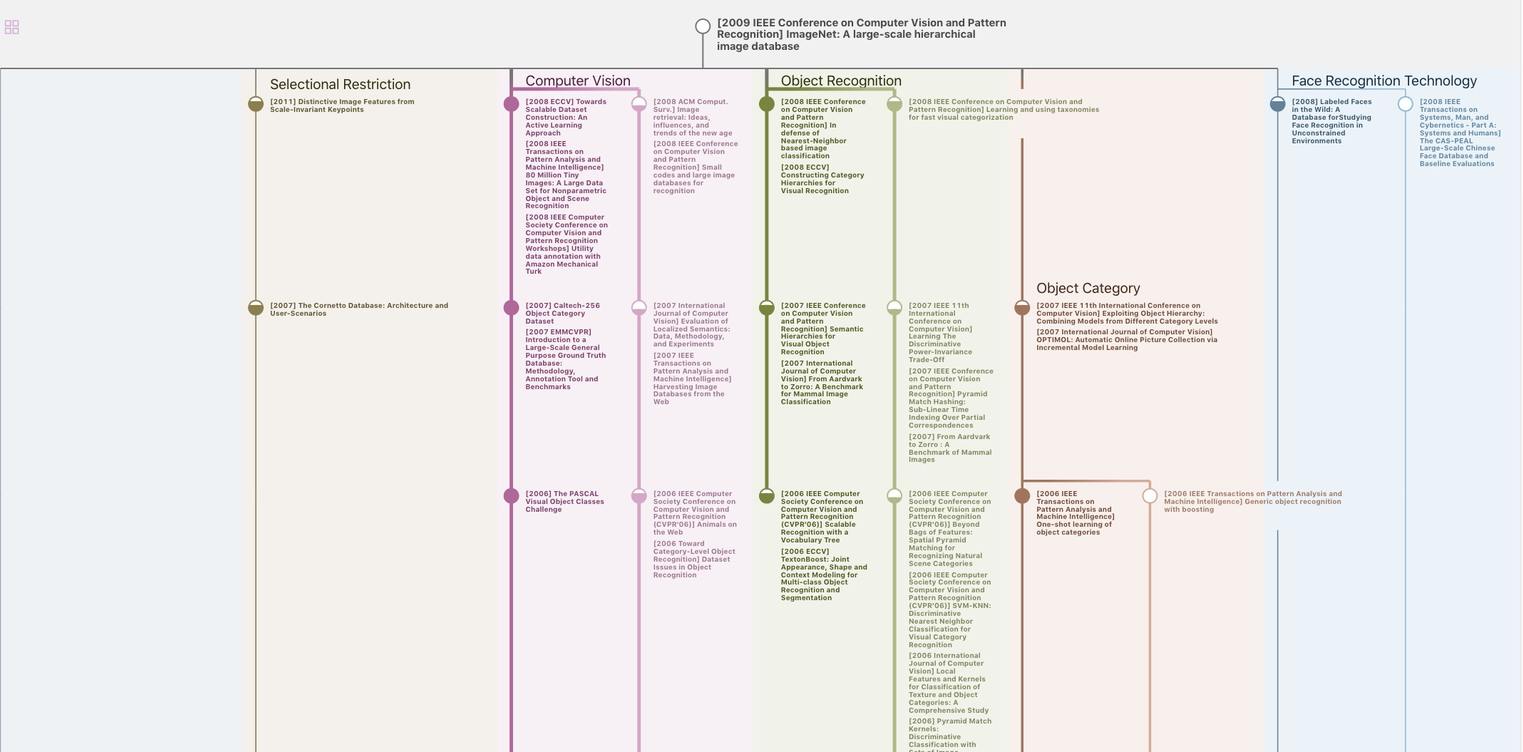
Generate MRT to find the research sequence of this paper
Chat Paper
Summary is being generated by the instructions you defined