S2S Extreme Weather Featurization: A Global Skill Assessment Study
crossref(2022)
摘要
<p><span>A more accurate characterization of S2S </span><span>extremes</span> <span>may result in great positive </span><span>societal impact. </span><span>Featurized S2S forecasts in the form of risk or extreme indices</span> <span>will aid in </span><span>disaster </span><span>response</span><span> (especially for drought and flood events), inform disease outbreaks and heatwave onset, persistence, and decay.</span><span> In this study, we identify a set of ECMWF-derived extreme weather indices that have spatio-temporal windows of opportunity for better-than-climatology skill. We report on the correlation between ECMWF-derived indices and ground-truth values.  The selected indices can be calculated directly form probabilistic daily forecas</span><span>ts, or alternatively, by training specialized ML-models to process ensembles in a multi-task learning setup. Our goal is to find better approaches to communicate S2S climate risk by deploying a set of ECMWF-derived climate forecast products.<br></span></p>
更多查看译文
AI 理解论文
溯源树
样例
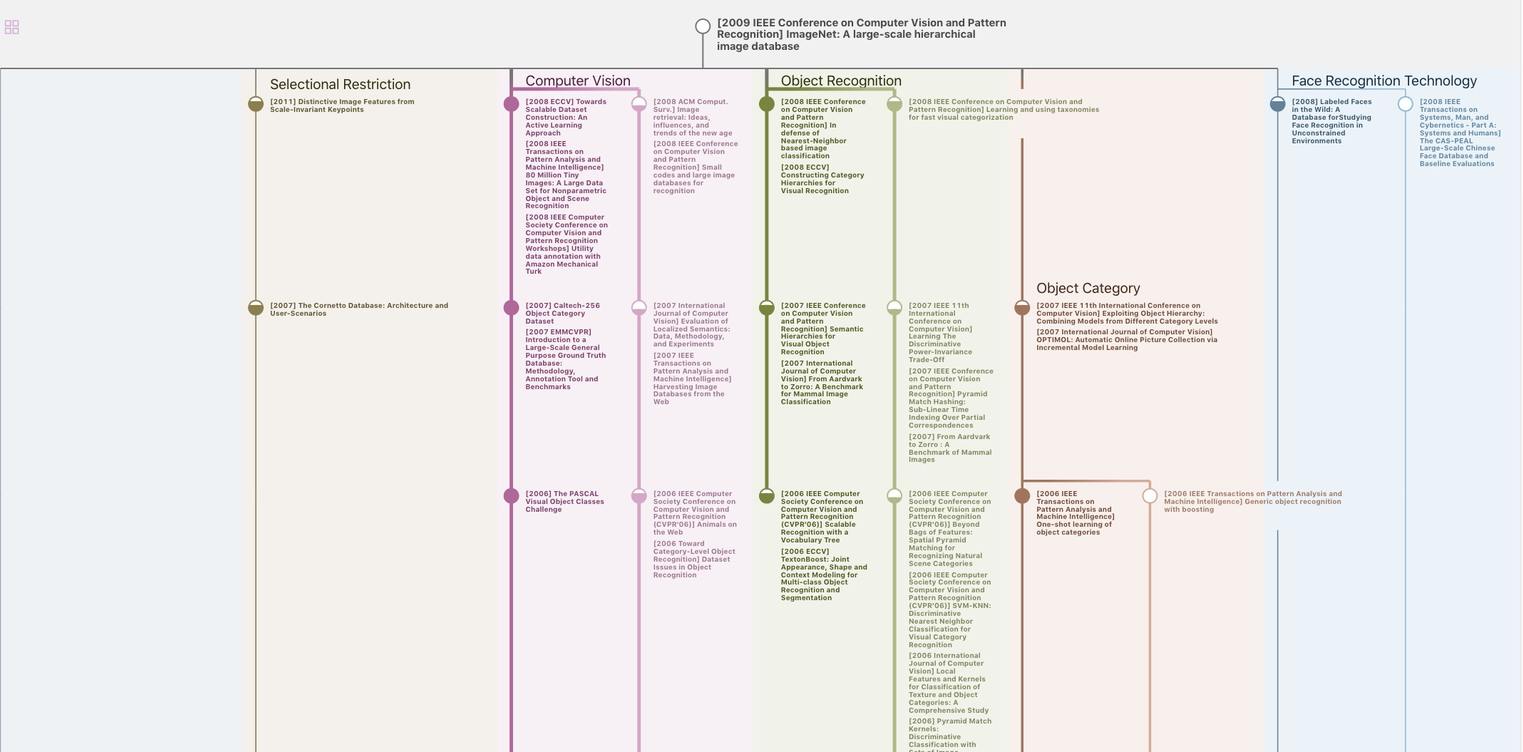
生成溯源树,研究论文发展脉络
Chat Paper
正在生成论文摘要