A benchmark for probabilistic seasonal streamflow forecasting over North America
crossref(2022)
摘要
<p>Seasonal streamflow forecasts represent critical operational inputs for water sectors and society, for instance for spring flood early warning, water supply, hydropower generation, and irrigation scheduling. Initial hydrological conditions (e.g., snow cover and soil moisture) are an important driver of hydrological predictions on these timescales. In high-latitude and/or high-altitude basins across North America, and the basins downstream of these headwaters, snow is one of the main sources of runoff generation. As a result, data-driven forecasting from snow observations is a well-established approach for operational seasonal streamflow forecasting in the USA (Fleming et al., 2021) and Canada (Zahmatkesh et al., 2019).</p><p>As part of the Global Water Futures programme (GWF), we are advancing capabilities for probabilistic streamflow forecasting over North America. The first aim of this work is to benchmark probabilistic seasonal streamflow predictability across the continent. To this end, a data-driven probabilistic seasonal streamflow hindcasting system is being developed and implemented for basins with a nival regime across North America. It uses snow water equivalent measurements from the recent update of the Canadian historical Snow Water Equivalent dataset (CanSWE, 1928–2020; Vionnet et al., 2021) and the Natural Resources Conservation Service (NRCS) manual snow surveys and the SNOTEL automatic snow pillow in the USA. These datasets are gap filled using quantile mapping based on neighbouring snow and precipitation stations (SCDNA dataset; Tang et al., 2020), and subsequently transformed into principal components. These principal components are then used as predictors into a regression model, to generate ensemble hindcasts of streamflow volumes for basins across North America. Preliminary results indicate that this approach is skilful (i.e., better than streamflow climatology) for basins across the Canadian Rockies during the snowmelt season.</p><p><strong>References</strong></p><p>Fleming, S. W., Garen, D. C., Goodbody, A. G., McCarthy, C. S., and Landers, L. C.: Assessing the new Natural Resources Conservation Service water supply forecast model for the American West: A challenging test of explainable, automated, ensemble artificial intelligence. Journal of Hydrology, 602, https://doi.org/10.1016/j.jhydrol.2021.126782, 2021.</p><p>Tang, G., Clark, M. P., Newman, A. J., Wood, A. W., Papalexiou, S. M., Vionnet, V., and Whitfield, P. H.: SCDNA: a serially complete precipitation and temperature dataset for North America from 1979 to 2018, Earth Syst. Sci. Data, 12, 2381–2409, https://doi.org/10.5194/essd-12-2381-2020, 2020.</p><p>Vionnet, V., Mortimer, C., Brady, M., Arnal, L., and Brown, R.: Canadian historical Snow Water Equivalent dataset (CanSWE, 1928–2020), Earth Syst. Sci. Data, 13, 4603–4619, https://doi.org/10.5194/essd-13-4603-2021, 2021.</p><p>Zahmatkesh, Z., Sanjeev Kumar, J., Coulibaly, P., and Stadnyk, T.: An overview of river flood forecasting procedures in Canadian watersheds, Canadian Water Resources Journal / Revue canadienne des ressources hydriques, 44, 3, https://doi.org/10.1080/07011784.2019.1601598, 2019.</p>
更多查看译文
AI 理解论文
溯源树
样例
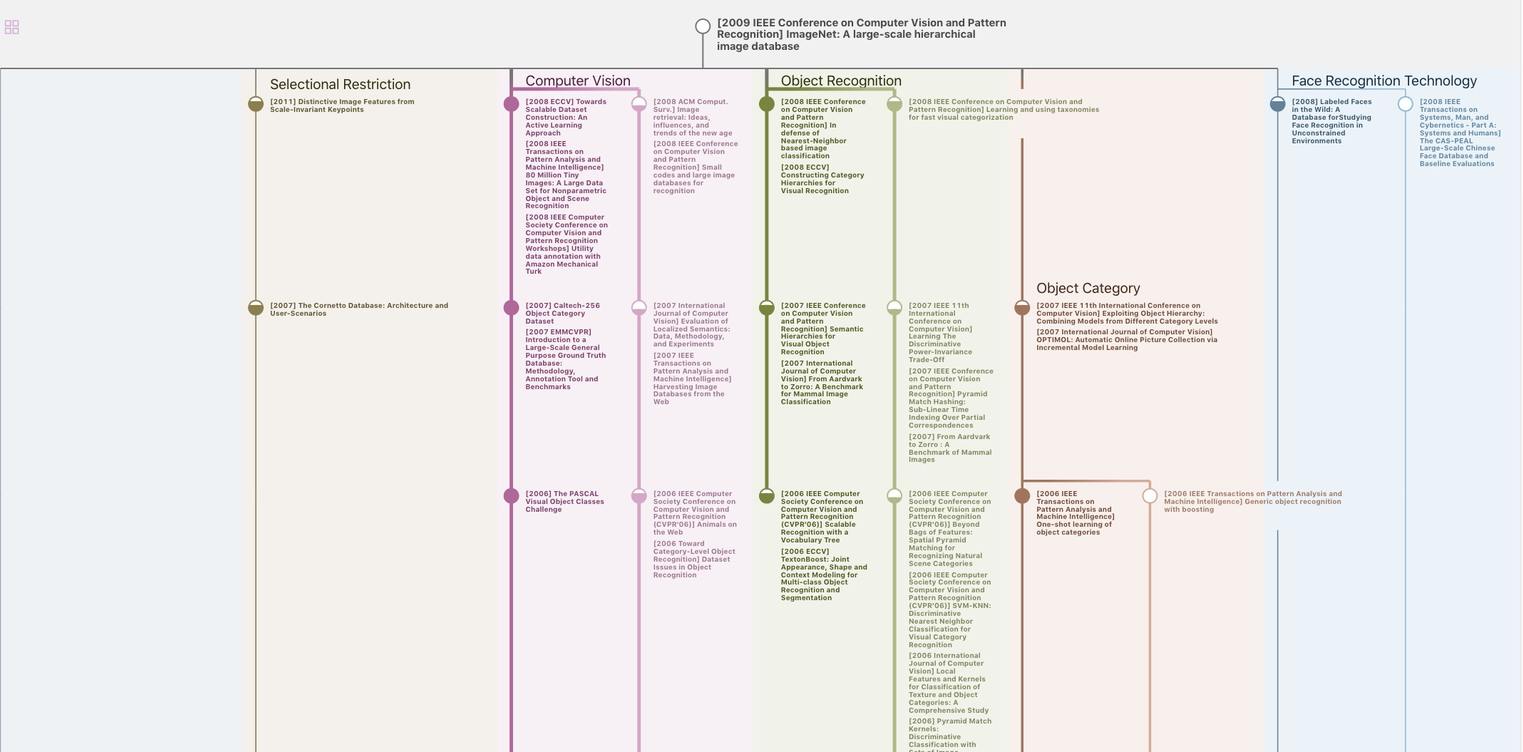
生成溯源树,研究论文发展脉络
Chat Paper
正在生成论文摘要