Quantitative comparison of root water uptake simulated by functional-structural root architecture models
crossref(2022)
摘要
<p>3D models of root growth, architecture and function are becoming important tools to aid the design of agricultural management schemes and the selection of beneficial root traits. While benchmarking is common for water and solute transport models in soil, 3D root-soil interaction models have not yet been systematically analysed. Several interacting processes might induce disagreement between models: root growth, sink term definitions of root water and solute uptake and representation of the rhizosphere. Schnepf et al. (2020) proposed a framework for quantitatively comparing such models. It builds upon benchmark scenarios that test individual components, followed by benchmark scenarios for the coupled root-rhizosphere-soil system.</p><p>Here we present the results of benchmarking different well-known models (“simulators”) with respect to water flow in soil, water flow in roots, and water flow and root water uptake in a coupled soil-root system for the case of a given prescribed root architecture as observed from an MRI experiment. The participating simulators are</p><p>CPlantBox and DuMux (Koch et al. 2021; Mai et al. 2019), R-SWMS (Javaux et al. 2008), OpenSimRoot (Postma et al. 2017) and ArchiSimple, RootTyp and SRI (Beudez et al. 2013; Pagès et al. 2014; Pagès et al. 2004).</p><p>In the benchmark scenarios that represent individual modules, the different simulators solved the same mathematical model but with different numerical approaches; all perform well with respect to the given analytical reference solution. For the coupled problem of root water uptake from a drying soil, the different simulators make different choices for the coupling of the different sub-problems. Thus, the results of the different simulators show a larger heterogeneity amongst each other.</p><p>We expect that this benchmarking will result in improved models, with which we can simulate various scenarios with greater confidence, avoiding that future work is based on accidental results caused by bugs, numerical errors or conceptual misunderstandings and will set a standard for model development.</p><p>Beudez N, Doussan C, Lefeuve-Mesgouez G, Mesgouez A (2013) Procedia Environmental Sciences 19: 37-46. doi:</p><p>Javaux M, Schröder T, Vanderborght J, Vereecken H (2008) Vadose Zone Journal 7: 1079-1088.</p><p>Koch T, Wu H, Schneider M (2021) Journal of Computational Physics: 110823.</p><p>Mai TH, Schnepf A, Vereecken H, Vanderborght J (2019) Plant and Soil 439: 273-292. doi: 10.1007/s11104-018-3890-4.</p><p>Pagès L, Bécel C, Boukcim H, Moreau D, Nguyen C, Voisin A-S (2014) Ecological Modelling 290: 76-84.</p><p>Pagès L, Vercambre G, Drouet J-L, Lecompte F, Collet C, Le Bot J (2004) Plant and Soil 258: 103-119.</p><p>Postma JA, Kuppe C, Owen MR, Mellor N, Griffiths M, Bennett MJ, Lynch JP, Watt M (2017) New Phytologist 215: 1274-1286.</p><p>Schnepf A, Black CK, Couvreur V, et al. (2020) Frontiers in Plant Science 11.</p>
更多查看译文
AI 理解论文
溯源树
样例
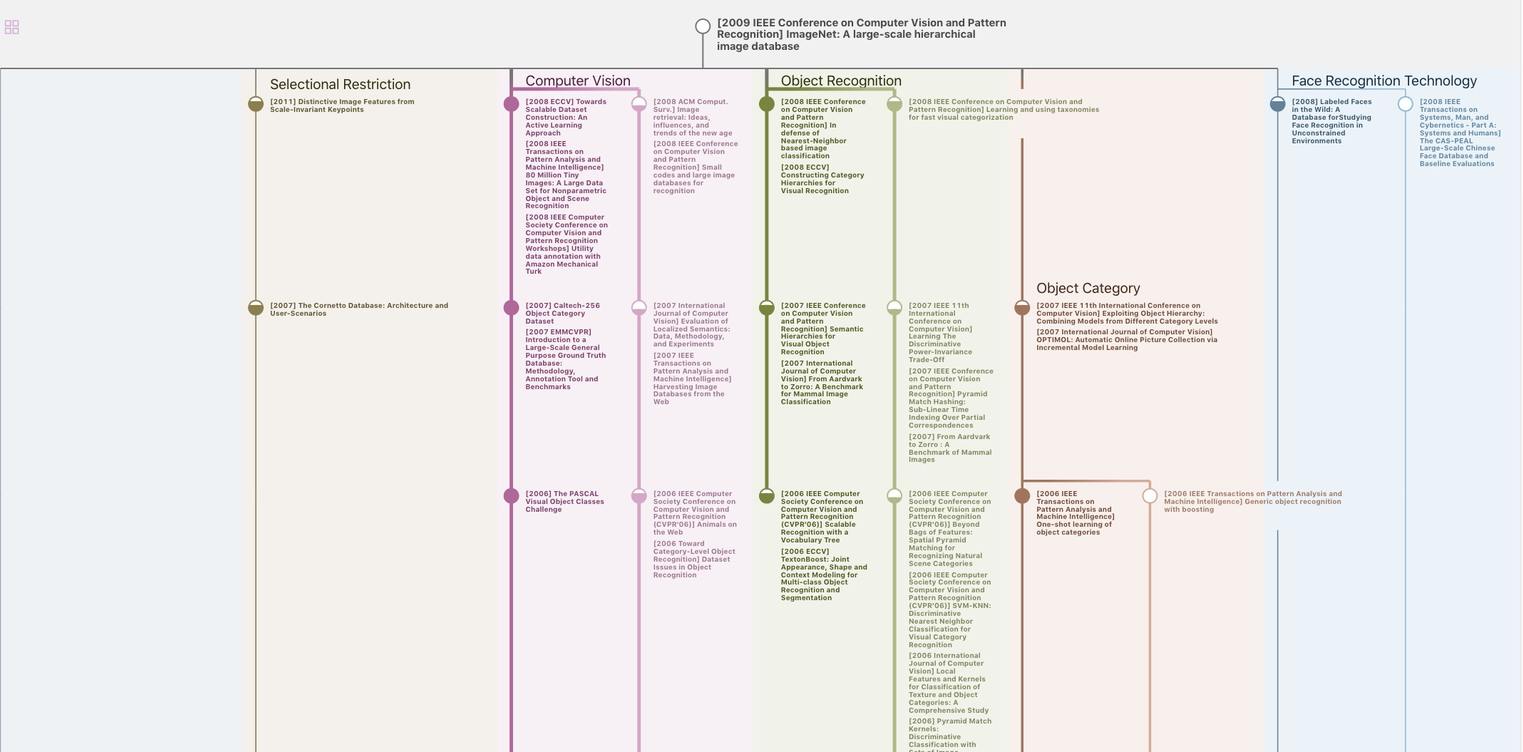
生成溯源树,研究论文发展脉络
Chat Paper
正在生成论文摘要