Error hotpots of the modelled global terrestrial water storage interannual variation
crossref(2022)
摘要
<p>Terrestrial water storage (TWS) is a socially (e.g., floods and drought) and scientifically (e.g., water and carbon cycles) important variable. Hydrological models have been extensively used to study variations in TWS but the models are showing significant uncertainties, especially for the interannual variability (IAV). It is therefore essential for TWS IAV studies to further improve model accuracy, calling for a better understanding of the TWS IAV simulation error. We conducted a covariance matrix analysis to spatially attribute the contributions to global TWS IAV and its simulation error by two hydrological models: 1) a parsimonious process-based one, implemented in the Strategies to INtegrate Data and BiogeochemicAl moDels (SINDBAD) framework, and 2) a hybrid one, the hybrid hydrological model (H2M), which combines a dynamic neural network and a water balance concept. Both models were calibrated against observation-based data streams for evapotranspiration, snow water equivalent, and runoff, as well as against the Gravity Recovery and Climate Experiment (GRACE) satellite observations of TWS. Both models indicate that the global TWS IAV is largely driven by some regions such as Amazon, Zambezi, Mekong basins, and India. The analysis also identified hotspots of the global TWS IAV error from river basins (e.g., Amazon, Paraná, Congo, and Mekong basins) and inland water bodies (e.g., the Laurentian Great Lakes). Excluding those hotspots in the global integration, the 12-month running mean of the global TWS IAV showed a large improvement: R2 of the global TWS IAV time series was improved from 0.62 to 0.82 for SINDBAD and from 0.62 to 0.88 for H2M. Therefore, the model simulation of the global TWS can efficiently be improved by focusing on correcting the hotspot regions. Comparing GRACE and modelled TWS IAV time series revealed various potential sources of errors, including anthropogenic factors (e.g., reservoir management) and model structure (e.g., insufficient storage capacity and missing storage processes), while the latter was prevalent over many regions. A further comparison to surface water data could characterize the hotspots as areas of 1) more dynamic surface water, and 2) wetlands (i.e., near-inland water bodies). These characteristics of hotspots imply that surface water processes (e.g., seasonal inundation) are relevant for understanding global TWS IAV and are underestimated in the two tested models, calling for further improvement in that respect. Our approach presents a general avenue to identify model simulation errors for global data streams and can guide efficient model development.</p>
更多查看译文
AI 理解论文
溯源树
样例
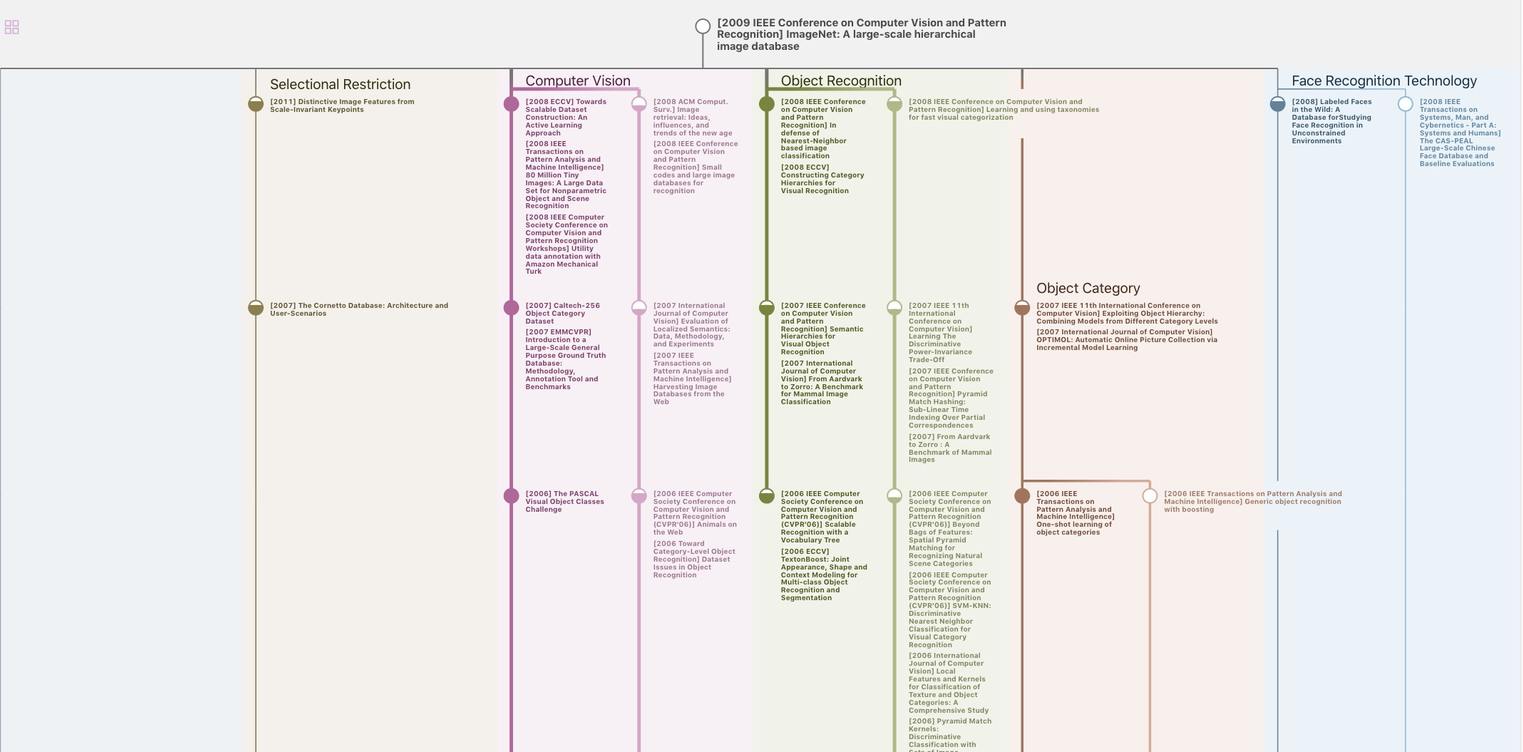
生成溯源树,研究论文发展脉络
Chat Paper
正在生成论文摘要