Content recommendation systems in mental healthcare: Practical application and formative evaluation (Preprint)
crossref(2022)
摘要
BACKGROUND Recommender systems have great potential in mental health care to provide self-guided content to supplement the mental health journey for patients scalably; however, traditional filtering approaches often have skewed input data distributions, are static or may not account for changes in symptoms and clinical presentation. In this study, we describe and evaluate two knowledge-based content recommendation system models as part of Ginger, an on-demand mental health platform that seek to address the issues above. OBJECTIVE In this study, we describe and evaluate two knowledge-based content recommendation system models as part of Ginger, an on-demand mental health platform that seek to address the issues above. METHODS We developed two models to provide content recommendations for the Ginger mental health app content. First, a method that uses members' responses to onboarding questions to recommend content cards from the content library of the mental health platform. Second, a dynamic conversation-based recommendation method that matches the semantic similarity between the content of a conversation and the text description of content cards to make recommendations suitable for a conversational snippet. As a measure of success for these recommendation models, we examined the relevance of content cards to members’ conversations with their coach and completion rates of selected content within the app measured over 14018 users. RESULTS Conversation-based recommendation models performed better than random recommendations for all lengths of conversational sessions considering fractions of relevant cards as well as very relevant cards. In an offline analysis, conversation-based recommendations had a 16.1% higher relevance rate (for the top 5 recommended cards) averaged across sessions of varying lengths as compared to a random control algorithm. Comparing completion rates of content delivered in the app to over 14018 users, conversation-based content recommendations that had 11.4% higher completion rates per card than onboarding response based recommendations (P=.003) and 26.1% higher than random recommendations (P=.005)). CONCLUSIONS Recommender systems can help scale and supplement digital mental health care with relevant content and self-care recommendations. Conversation-based recommendation models allow for dynamic recommendations based on information gathered during the course of text-based coaching sessions, which is a critical capability given the changing nature of mental health over the course of treatment.
更多查看译文
AI 理解论文
溯源树
样例
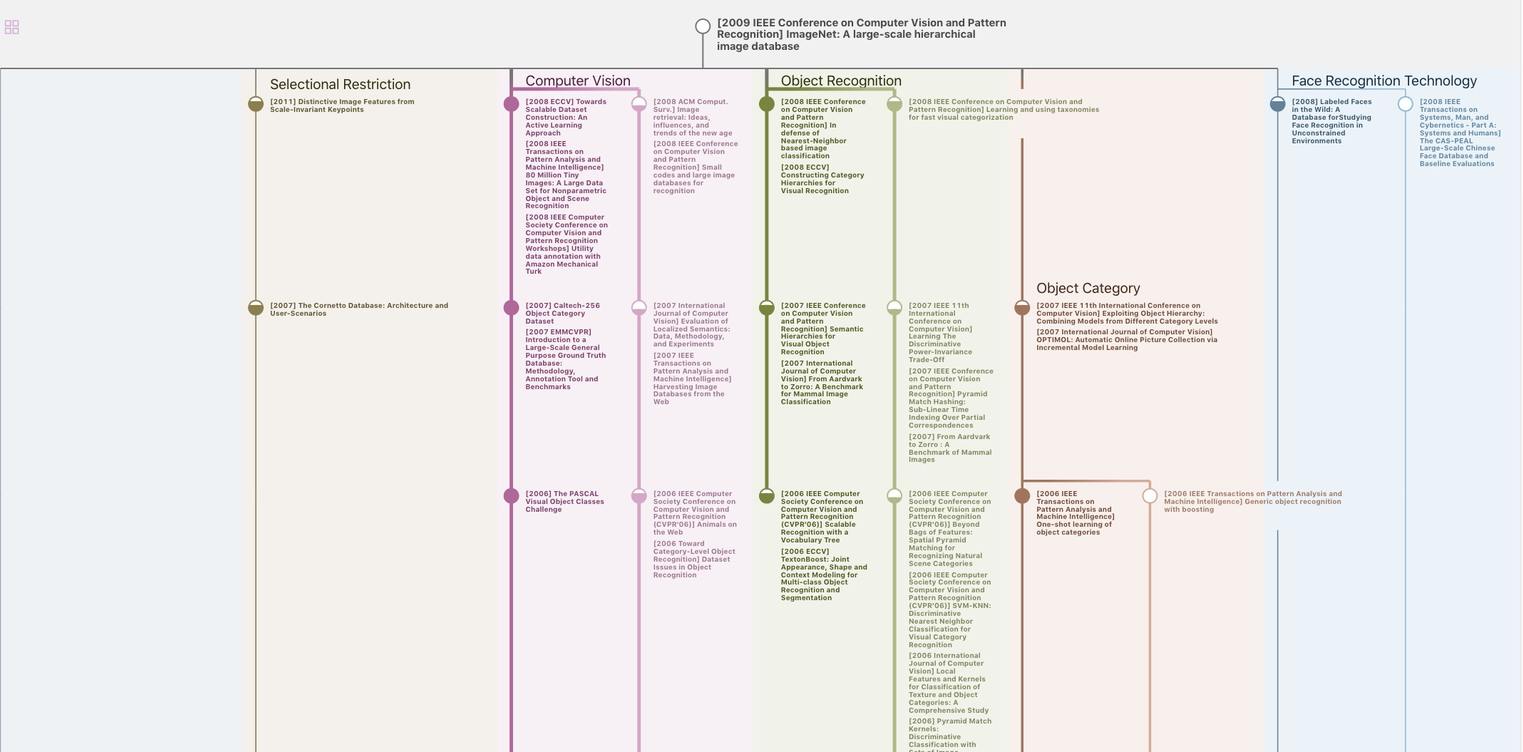
生成溯源树,研究论文发展脉络
Chat Paper
正在生成论文摘要