Application of an adaptable multimodal machine learning approach to predict COVID-19 outcomes for hospital resource management
Research Square (Research Square)(2022)
摘要
Abstract Beyond the health consequences, coronavirus disease 2019 (COVID-19) has shown the inadequacies and limitations of healthcare systems all over the world. Predictions of good and bad outcomes could improve lockdown and curfew policies and reduce the COVID-19 health impact. We describe a multimodal machine learning (ML) approach for predicting early hospital discharge and other key clinical outcomes, such as respiratory worsening, intensive care unit admission or the need for rescue therapy. Using this model, our hospital occupancy could have decreased by about 50% during the first wave of the pandemic. Our model used both raw laboratory results (LR) and electronic health records (EHR), which allow better anticipation of worsening through selective reporting of LRs. Our approach could be a useful, customizable, and adaptable hospital resource management tool, especially to assist in interventions targeted at reducing COVID-19 infection rates.
更多查看译文
关键词
hospital resource management,adaptable multimodal machine,machine learning
AI 理解论文
溯源树
样例
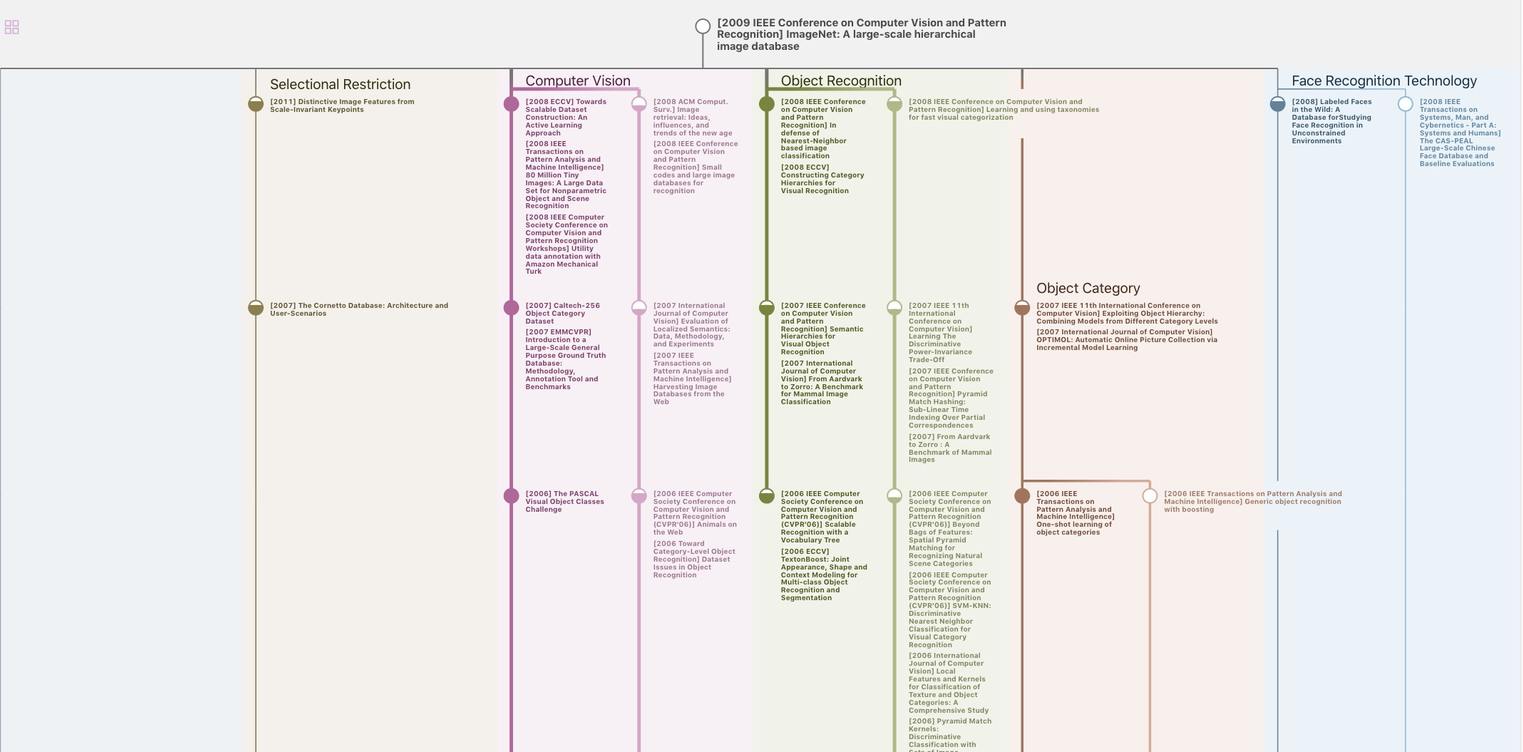
生成溯源树,研究论文发展脉络
Chat Paper
正在生成论文摘要