Machine Learning Prediction of Stroke Occurrence: A Systematic Review
crossref(2024)
摘要
Background Machine learning is nowadays commonly used for disease prediction, including cardiovascular disease. There is growing evidence of the effectiveness of machine learning algorithms for stroke risk prediction models.
Aims A systematic review was conducted to identify and comprehensively evaluate the available evidence.
Summary of review Relevant studies were identified from the three electronic databases (i) MEDLINE via Pubmed, (ii) Scopus, and (iii) IEEE Xplore from inception to 1st December 2020. Out of 12,626 studies identified, 40 used machine learning for ischemic or hemorrhagic stroke risk prediction models. Synthesis without meta-analysis identified that a boosting algorithm (median C-statistics = 0.9 (interquartile range [IQR]: 0.88-0.92)), and neural network (median C-statistic = 0.80 (IQR: 0.77-0.92)) performed best among ML models in the low risk of bias studies. Moreover, a boosting algorithm also performed best in overall (both low and high risk of bias) studies (median C-statistic = 0.92 (IQR: 0.90-0.95)).
Conclusions The systematic review found promising results of the ML algorithm model performances compare with the gold standard conventional models, such as FSRP (C-statistic 0.653) and revised FSRP (C-statistic 0.716). In term of the algorithm, boosting and neural networks are robust, but are considered as black-box models, since they are composed of non-linearity and complex algorithms. It remains questionable whether a physician would adapt these algorithms to use in a real clinical setting. Moreover, less than half of the studies (16 out of 40) were at low risk of bias in our systematic review. More researches with good methodology and study design, alongside explainable and good performance models, may become available in the future.
Trial Registration Information The International Prospective Register of Systematic Reviews (PROSPERO) database (ID: CRD42021234081).
### Competing Interest Statement
The authors have declared no competing interest.
### Funding Statement
This study was granted by the National Research Council of Thailand (NRCT) N42A640323. The grant agency did not involve in review methods (selection of studies, risk of bias assessment, data extractions, data analysis, and interpretation of findings), writing the manuscript, and did not impose any restriction regarding the publication of the manuscript.
### Author Declarations
I confirm all relevant ethical guidelines have been followed, and any necessary IRB and/or ethics committee approvals have been obtained.
Yes
I confirm that all necessary patient/participant consent has been obtained and the appropriate institutional forms have been archived, and that any patient/participant/sample identifiers included were not known to anyone (e.g., hospital staff, patients or participants themselves) outside the research group so cannot be used to identify individuals.
Yes
I understand that all clinical trials and any other prospective interventional studies must be registered with an ICMJE-approved registry, such as ClinicalTrials.gov. I confirm that any such study reported in the manuscript has been registered and the trial registration ID is provided (note: if posting a prospective study registered retrospectively, please provide a statement in the trial ID field explaining why the study was not registered in advance).
Yes
I have followed all appropriate research reporting guidelines, such as any relevant EQUATOR Network research reporting checklist(s) and other pertinent material, if applicable.
Yes
Data sharing is not applicable to this article as no new data were created or analyzed in this study.
* AI
: Artificial Intelligence
BMI
: Body Mass Index
CVD
: Cardiovascular disease
DT
: Decision tree
EHR
: Electronic Health Record
FPR
: False Positive Rate
FSRP
: Framingham Stroke Risk Profile
IQR
: Interquartile Range
LASSO
: Least Absolute Shrinkage and Selection Operator
MACE
: Major Advanced Cardiovascular Event
ML
: Machine Learning
NHIRD
: the National Health Insurance Research Database
NN
: Neural network
PCA
: Principal Component Analysis
PRISMA
: Preferred Reporting Information for Systematic Reviews and Meta-Analysis
PROBAST
: The Prediction model study Risk of Bias Assessment Tool
PROSPERO
: The International Prospective Register of Systematic Reviews
RCT
: Randomized Controlled Trial
RoB
: Risk of Bias
RF
: Random Forest
RWD
: Real-world Data
SMOTE
: Synthetic Minority Over-sampling Technique
SVM
: Support vector machine
SWiM
: Synthesis without meta-analysis
UCI
: University of California, Irvine
更多查看译文
AI 理解论文
溯源树
样例
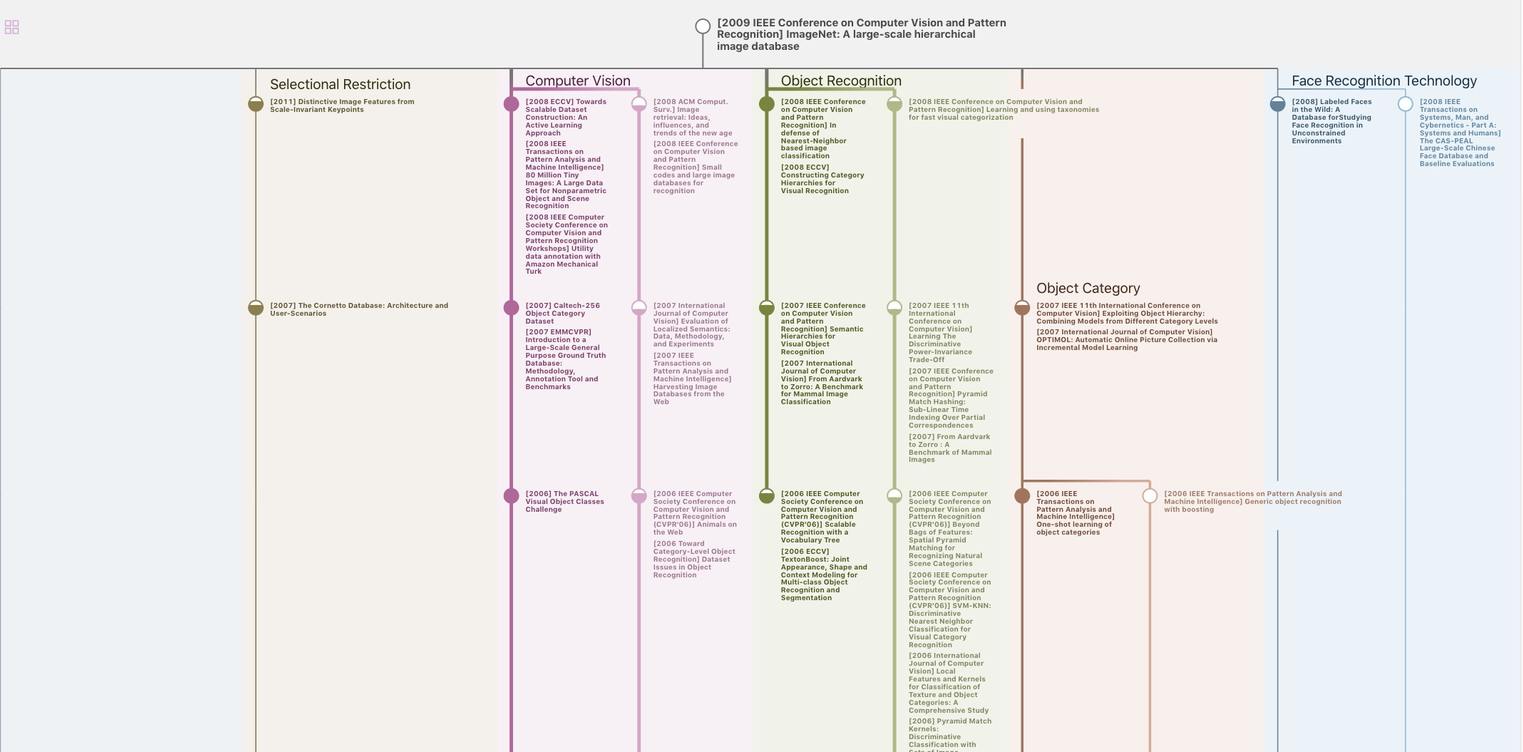
生成溯源树,研究论文发展脉络
Chat Paper
正在生成论文摘要