Variability in EHR Data About Race and Ethnicity As Observed in the National COVID Cohort Collaborative Data Enclave (Preprint)
crossref(2022)
摘要
BACKGROUND A significant technical challenge related to integrating race and ethnicity data across EHR systems is the lack of consistency in how data about race and ethnicity is collected and structured by healthcare organizations. OBJECTIVE To evaluate and describe variations in how healthcare systems collect and report information about the race and ethnicity of their patients, and how these data are integrated when it is aggregated into a large clinical database. METHODS At the time of our analysis, the National COVID Cohort Collaborative (N3C) Data Enclave contained records from 6.5 million patients contributed by 56 healthcare institutions. We assessed the quality of race and ethnicity data by analyzing its conformance to federal standards, then drilled into the non-conforming data. RESULTS “No matching category” was the second largest harmonized racial group in the N3C. 20.7% of the race data did not conform to the federal standard; the largest category was data that were missing. Hispanic or Latino patients were over-represented in the non-conforming racial data, and data from American Indian or Alaska Native patients were obscured. Although only a small proportion of the source data had not been mapped to the correct concepts (0.6%), Black or African-American and Hispanic/Latino patients were over-represented in this category. CONCLUSIONS The impact of data quality issues was not equal across all races and ethnicities, which has the potential to introduce bias in analyses and conclusions drawn from these data.The adverse impact of COVID-19 on marginalized and under-resourced communities of color has highlighted the need for accurate, comprehensive race and ethnicity data. Differences in how race and ethnicity data is conceptualized and encoded by healthcare institutions can affect the quality of the data in aggregated clinical databases. Transparency about how data has been transformed can help users make accurate analyses and inferences, and eventually better guide clinical care and public policy.
更多查看译文
AI 理解论文
溯源树
样例
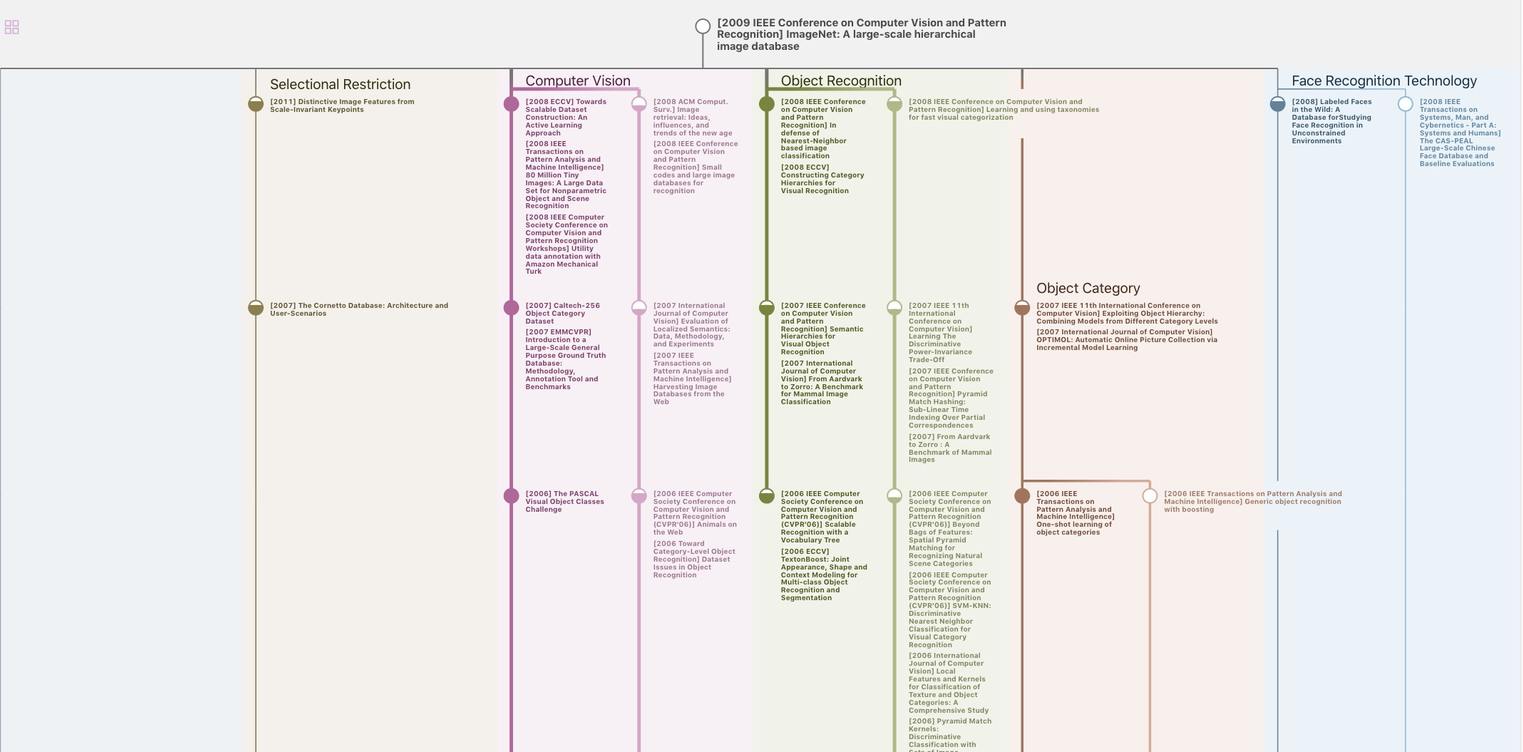
生成溯源树,研究论文发展脉络
Chat Paper
正在生成论文摘要