Regularization for Nonlinear System Identification
Communications and control engineering series(2022)
摘要
AbstractIn this chapter we review some basic ideas for nonlinear system identification. This is a complex area with a vast and rich literature. One reason for the richness is that very many parameterizations of the unknown system have been suggested, each with various proposed estimation methods. We will first describe with some details nonparametric techniques based on Reproducing Kernel Hilbert Space theory and Gaussian regression. The focus will be on the use of regularized least squares, first equipped with the Gaussian or polynomial kernel. Then, we will describe a new kernel able to account for some features of nonlinear dynamic systems, including fading memory concepts. Regularized Volterra models will be also discussed. We will then provide a brief overview on neural and deep networks, hybrid systems identification, block-oriented models like Wiener and Hammerstein, parametric and nonparametric variable selection methods.
更多查看译文
关键词
nonlinear system identification,regularization
AI 理解论文
溯源树
样例
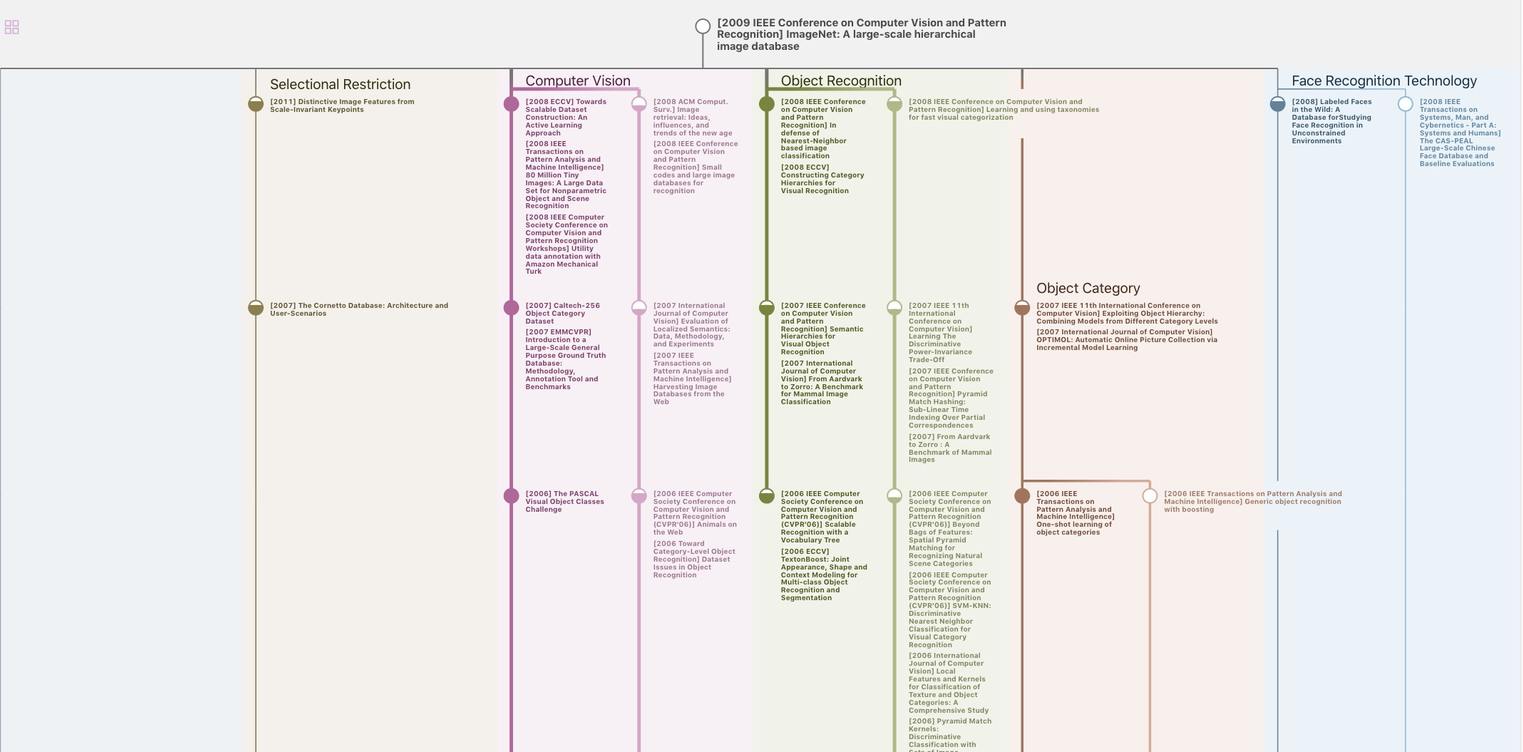
生成溯源树,研究论文发展脉络
Chat Paper
正在生成论文摘要