Neural scaling of deep chemical models
NATURE MACHINE INTELLIGENCE(2023)
摘要
Massive scale, in terms of both data availability and computation, enables important breakthroughs in key application areas of deep learning such as natural language processing and computer vision. There is emerging evidence that scale may be a key ingredient in scientific deep learning, but the importance of physical priors in scientific domains makes the strategies and benefits of scaling uncertain. Here we investigate neural-scaling behaviour in large chemical models by varying model and dataset sizes over many orders of magnitude, studying models with over one billion parameters, pre-trained on datasets of up to ten million datapoints. We consider large language models for generative chemistry and graph neural networks for machine-learned interatomic potentials. We investigate the interplay between physical priors and scale and discover empirical neural-scaling relations for language models in chemistry with a scaling exponent of 0.17 for the largest dataset size considered, and a scaling exponent of 0.26 for equivariant graph neural network interatomic potentials.
更多查看译文
关键词
neural scaling,models,chemical
AI 理解论文
溯源树
样例
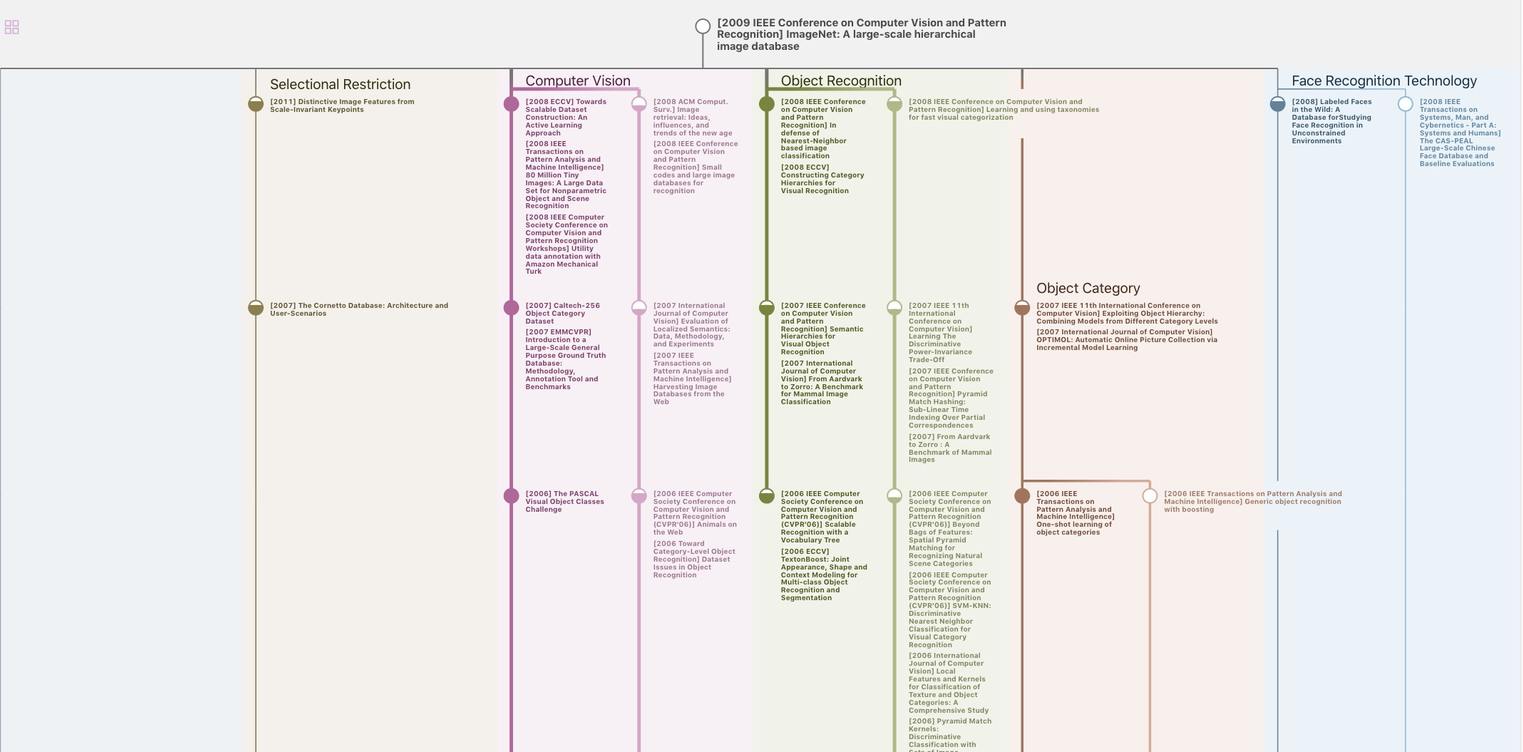
生成溯源树,研究论文发展脉络
Chat Paper
正在生成论文摘要