High-throughput deep learning variant effect prediction with Sequence UNET
bioRxiv (Cold Spring Harbor Laboratory)(2022)
摘要
AbstractUnderstanding the consequences of protein coding mutations is important for many applications in biology and medicine. The vast number of possible mutations across species makes comprehensive experimental characterisation impossible, even with recent high-throughput techniques, which means computationally predicting the consequences of variation is essential for many analyses. Previous variant effect prediction (VEP) tools, generally based on evolutionary conservation and protein structure, are often computationally intensive, making them difficult to scale and limiting potential applications. Recent developments in deep learning techniques, including protein language models, and biological data scale have led to a new generation of predictors. These models have improved prediction performance but are still often intensive to run because of slow training steps, hardware requirements and large model sizes. In this work we introduce a new highly scalable deep learning architecture, Sequence UNET, that classifies and predicts variant frequency directly from protein sequence. This model learns to build representations of protein sequence features at a range of scales using a fully convolutional U-shaped compression/expansion architecture. We show that it can generalise to pathogenicity prediction, achieving comparable performance on ClinVar to methods including EVE and ESM-1b at greatly reduced computational cost. We further demonstrate its scalability by analysing the consequences of 8.3 billion variants in 904,134 proteins detected in a large-scale proteomics analysis, showing a link between conservation and protein abundance. Sequence UNET can be run on modest hardware through an easy to use Python package.
更多查看译文
关键词
variant effect prediction,deep learning,sequence,high-throughput
AI 理解论文
溯源树
样例
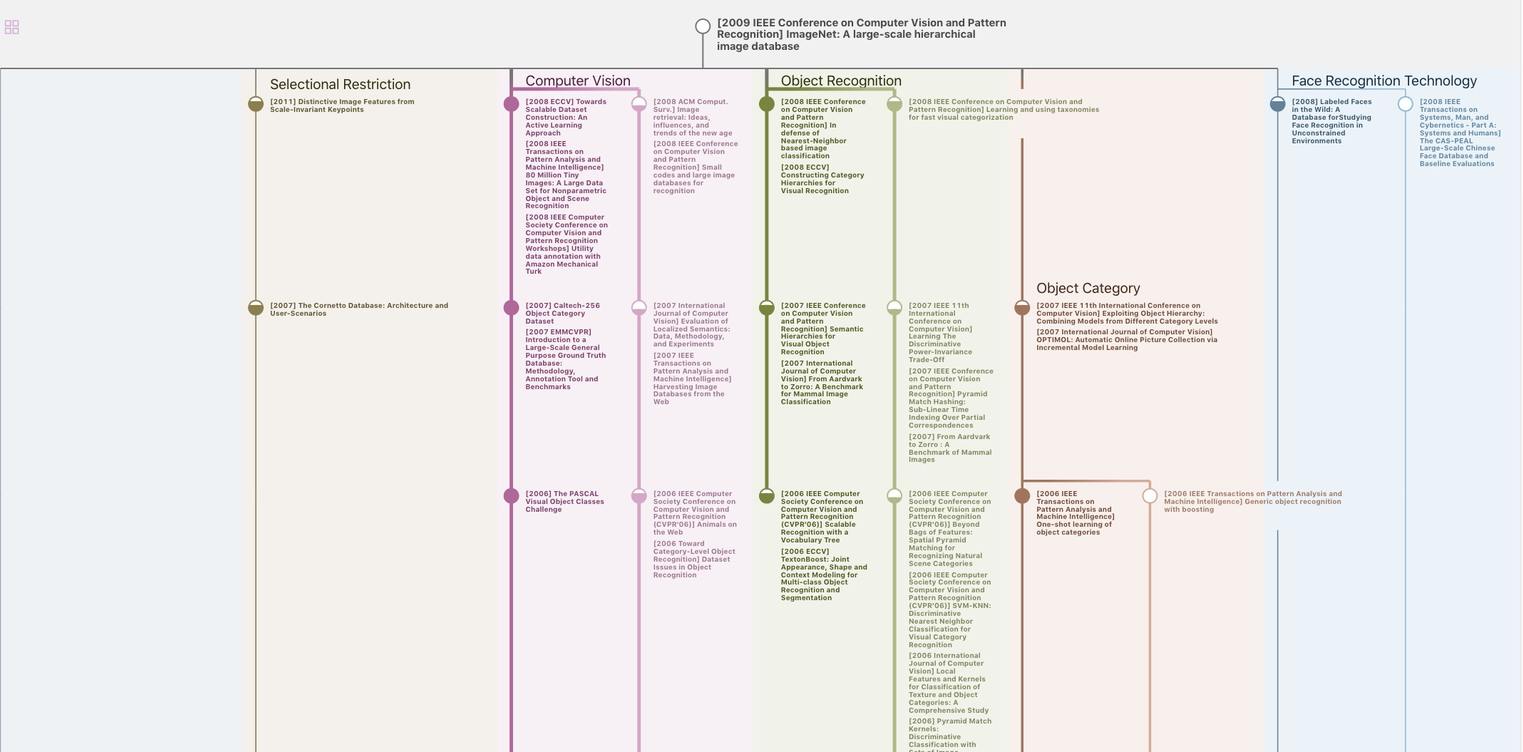
生成溯源树,研究论文发展脉络
Chat Paper
正在生成论文摘要