Identification of asymptomatic COVID-19 patients on chest CT images using Transformer-or CNN-based deep learning models
crossref(2022)
摘要
Abstract Backgroud To evaluate the feasibility of deep learning (DL) models in identifying asymptomatic COVID-19 patients, based on chest CT images. Methods In this retrospective study, six DL models (Xception, NASNet, ResNet, EfficientNet, ViT, and Swin), based on convolutional neural networks (CNNs)-or Transformer-architectures, were trained to identify asymptomatic patients with COVID-19 on chest CT images. Data from Yangzhou was randomly split into the training set (n = 2,140) and the internal-validation set (n = 360). Data from Suzhou was the external-test set (n = 200). Models’ performance was assessed by accuracy, recall and specificity and was compared with that of two radiologists. Results A total of 2,700 chest CT images were collected in this study. In the validation dataset, the Swin model achieved the highest accuracy of 0.994, followed by EfficientNet model (0.954). The recall and precision of the Swin model were 0.989 and 1.000. In the test dataset, the Swin model still was the best that achieved the highest accuracy (0.980). All the DL models performed remarkable than two experts. Lastly, the time on the test set diagnosis spent by two experts 42min17s (Junior) and 29min43s (Senior), was significantly higher than that of those DL models (all below 2min). Conclusions This study evaluated the feasibility of multiple DL models in distinguishing asymptomatic patients with COVID-19 from healthy subjects on chest CT images. It found a Transformer model, the Swin model, performed best.
更多查看译文
AI 理解论文
溯源树
样例
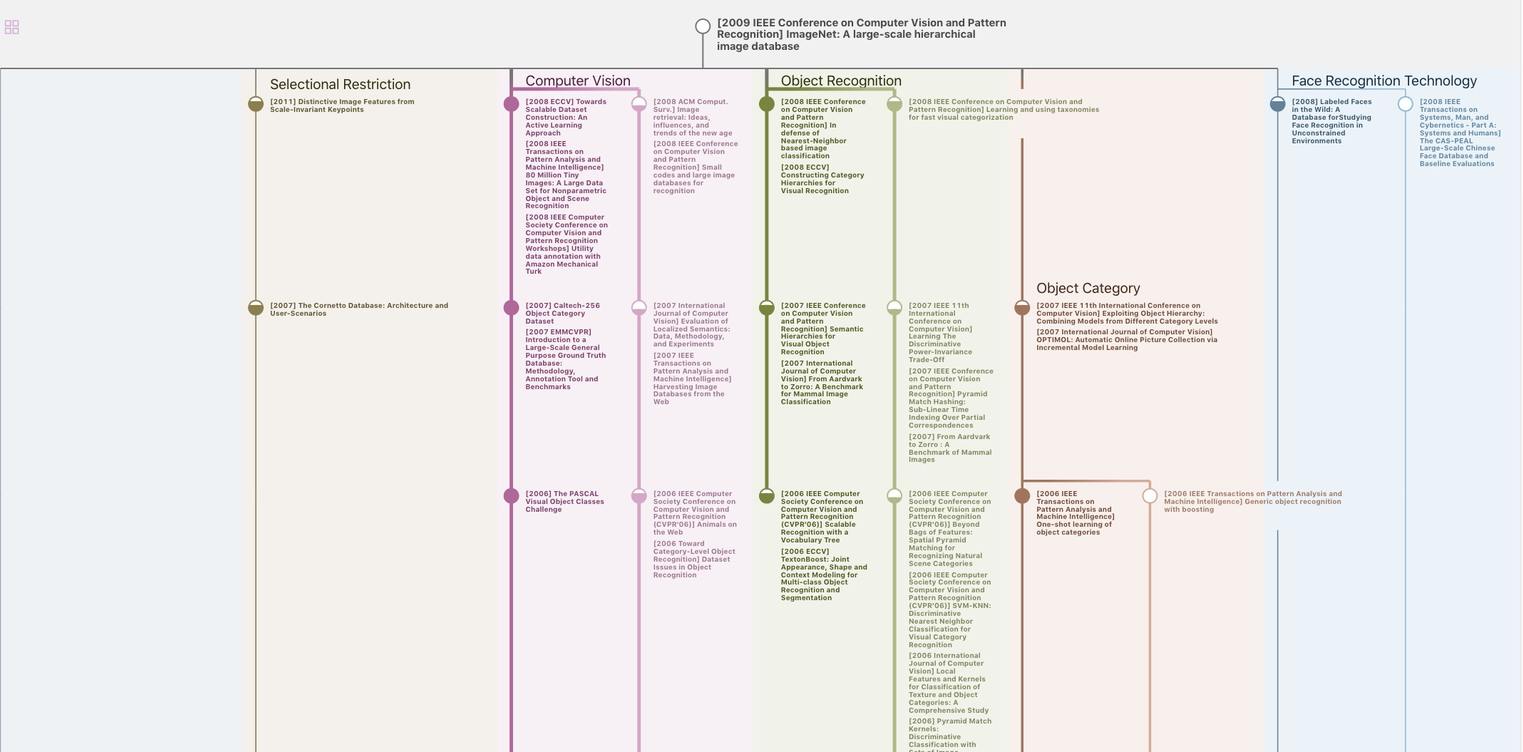
生成溯源树,研究论文发展脉络
Chat Paper
正在生成论文摘要