Chipless RFID Tag Implementation and Machine-Learning Workflow for Robust Identification
IEEE TRANSACTIONS ON MICROWAVE THEORY AND TECHNIQUES(2023)
摘要
In this work, we describe a complete step-by-step workflow to apply machine-learning (ML) classification for chipless radio-frequency identification (RFID) tag identification, covering: 1) the tag implementation criteria for circular ring resonator (CRR) and square ring resonator (SRR) arrays for ML interoperability; 2) the data collection procedure to get a sufficiently representative dataset of real measurements; 3) the ML techniques to visualize the data and reduce its dimensionality; 4) the evaluation of the ML classifier to ensure high-accuracy predictions on new measurements; and 5) a thresholding scheme to increase the certainty of the predictions. The differences in the tags' frequency responses are maximized by optimizing the Hamming distance between the tag identifiers (IDs) and by controlling each resonator array's radar cross section (RCS) level. We show that the proposed workflow achieves perfect accuracy for the identification of four tags at a fixed distance of 160 cm. We also evaluate the performance of the proposed workflow to identify up to 16 tags within a flexible range (up to 140 cm), showcasing the tradeoff between the number of tags that can be correctly classified based on the reading range.
更多查看译文
关键词
Chipless radio-frequency identification (RFID),circular ring resonators (CRR),logistic regression (LR),principal component analysis (PCA),regularization,resonator arrays,square-ring resonators (SRRs)
AI 理解论文
溯源树
样例
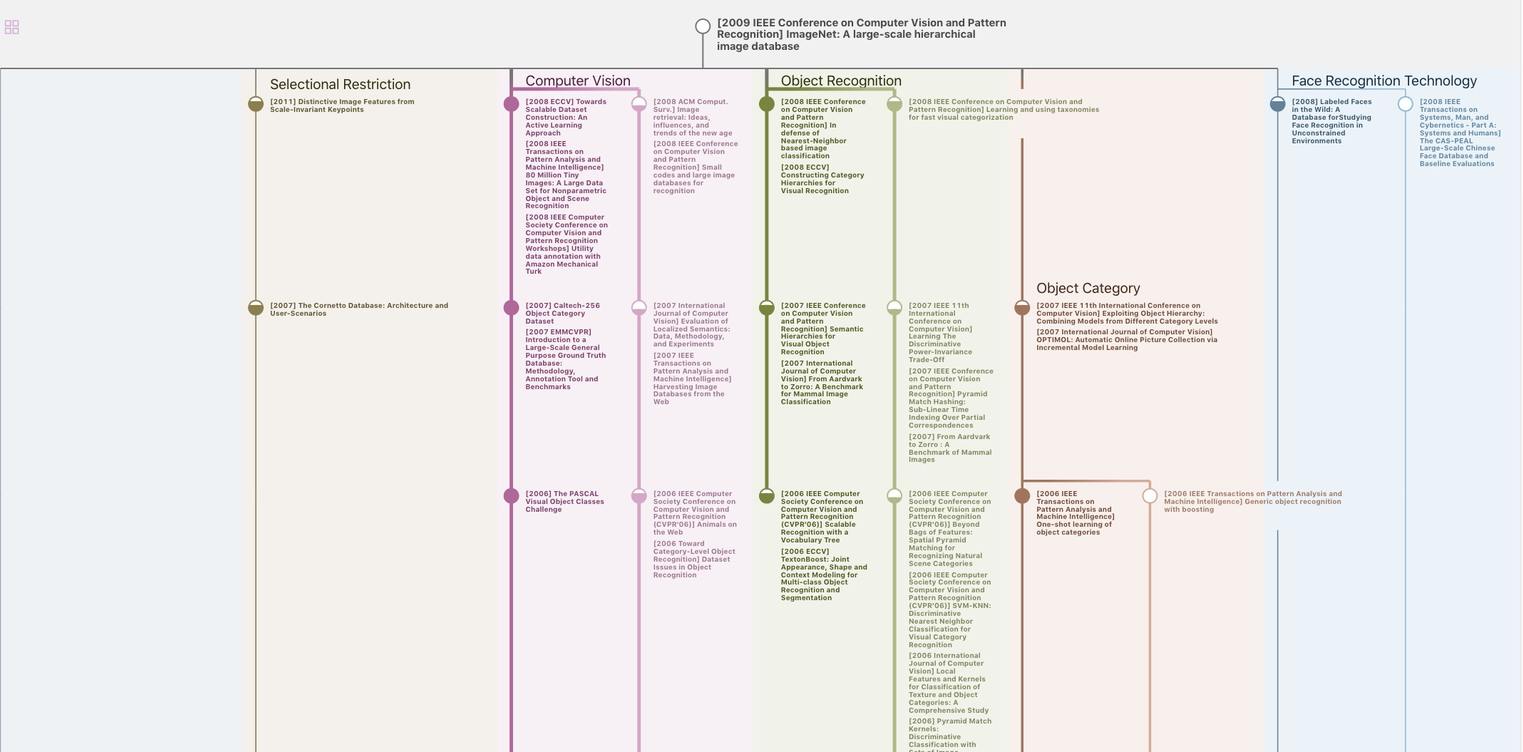
生成溯源树,研究论文发展脉络
Chat Paper
正在生成论文摘要