Performance Evaluation of Extracting Medical Information from Patient Notes using Natural Language Processing Methods (Preprint)
crossref(2022)
Abstract
UNSTRUCTURED Patient-generated health data (PGHD) is becoming a necessary component of remote monitoring and clinical decision-making as well as self-care and managing chronic conditions. Medical diaries and patient notes have been the primary source of unstructured PGHD, enabling patients to collect and communicate their health information remotely and in real-time to their providers. Yet there is no established mechanism or pipeline for processing free text patient notes (“unstructured PGHD”) or integrating these notes into clinical decision making in a low-resource setting (i.e., not depending on a large dataset or processing power). In the literature, there are a number of studies reporting NLP applications on clinical notes to identify symptoms and conditions, but these studies have been limited to public data (e.g. social media posts by patients). In this paper, we evaluate the performance of an hybrid (deep learning + rule-based) NLP pipeline on a low-resource PHGD dataset through an empirical evaluation of automatic component extraction where we measure the model’s ability to conduct automatic entity extraction using ontologies (medication-dose: RxNORM, symptoms: SNOMED) from patient notes.
MoreTranslated text
AI Read Science
Must-Reading Tree
Example
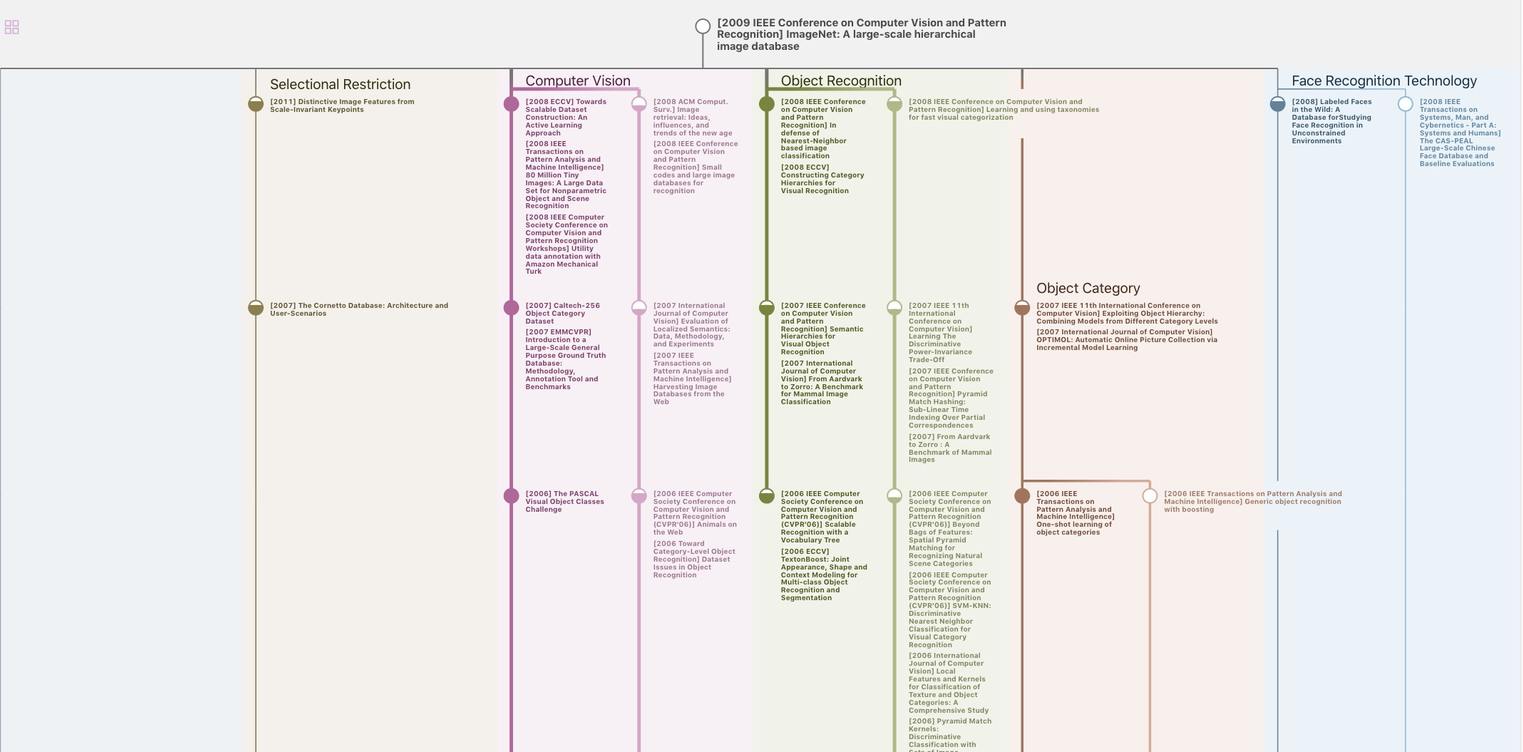
Generate MRT to find the research sequence of this paper
Chat Paper
Summary is being generated by the instructions you defined