Improving Machine Reading Comprehension with Contextualized Commonsense Knowledge
arXiv (Cornell University)(2022)
摘要
In this paper, we aim to extract commonsense knowledge to improve machine reading comprehension. We propose to represent relations implicitly by situating structured knowledge in a context instead of relying on a pre-defined set of relations, and we call it contextualized knowledge. Each piece of contextualized knowledge consists of a pair of interrelated verbal and nonverbal messages extracted from a script and the scene in which they occur as context to implicitly represent the relation between the verbal and nonverbal messages, which are originally conveyed by different modalities within the script. We propose a two-stage fine-tuning strategy to use the large-scale weakly-labeled data based on a single type of contextualized knowledge and employ a teacher-student paradigm to inject multiple types of contextualized knowledge into a student machine reader. Experimental results demonstrate that our method outperforms a state-of-the-art baseline by a 4.3% improvement in accuracy on the machine reading comprehension dataset C^3, wherein most of the questions require unstated prior knowledge.
更多查看译文
关键词
machine reading comprehension,contextualized commonsense
AI 理解论文
溯源树
样例
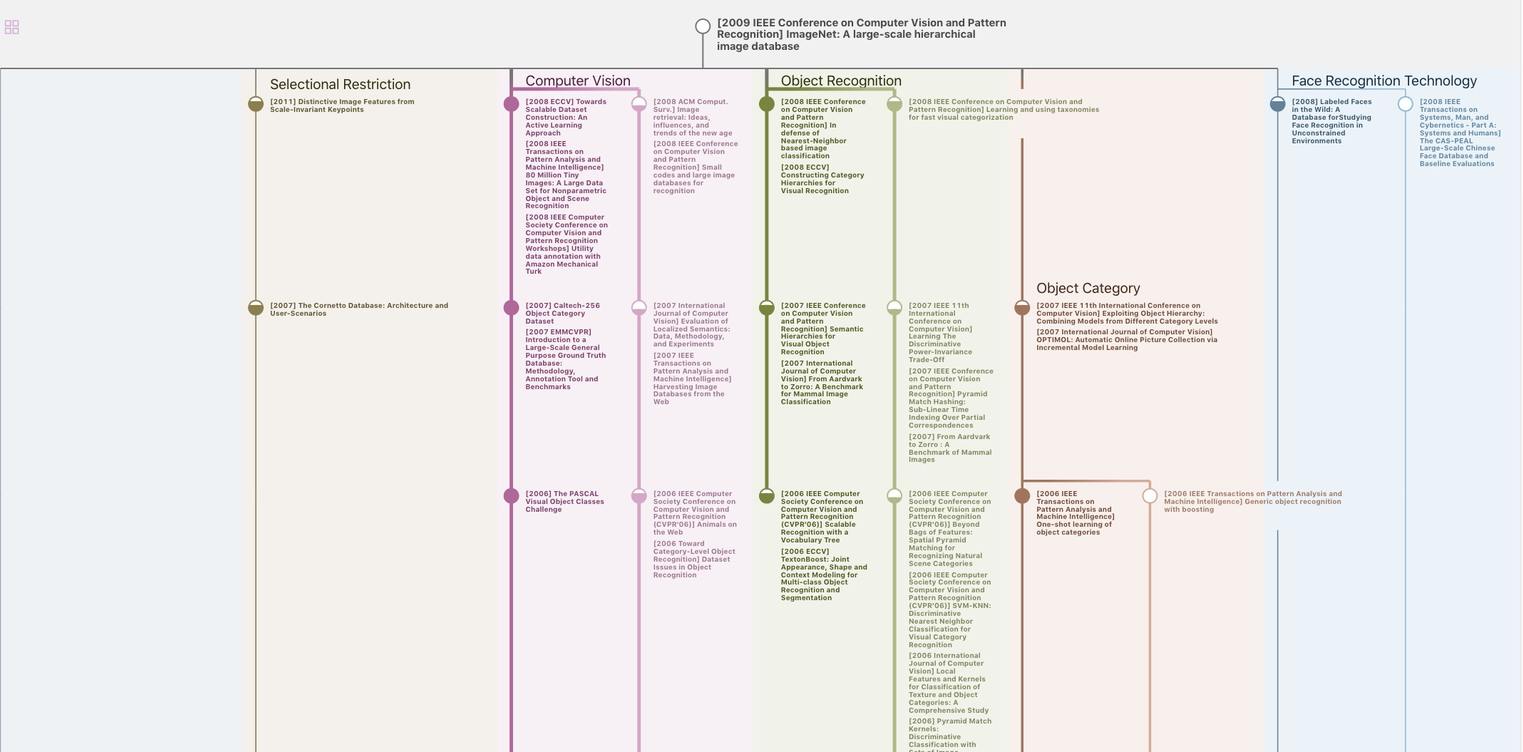
生成溯源树,研究论文发展脉络
Chat Paper
正在生成论文摘要