Assessment of sex and racial biases in electronic health records of emergency department patients with acute coronary syndrome
Research Square (Research Square)(2022)
摘要
Abstract Background Electronic health records (EHRs) contain individualized patient data that can be used to develop diagnostic and risk prediction models with artificial intelligence (AI) algorithms. Explicit and implicit sources of bias embedded in EHRs may hinder generalizable model performance and perpetuate bias. Objective This study explores the question of how existing sex and racial disparities in the clinical assessment and management of acute coronary syndrome (ACS) are reflected in patients’ EHRs. We outlined recommendations on how these sources of bias should be examined and mitigated within the framework of development and validation of AI-based diagnosis and risk stratification algorithms. Methods This retrospective study examined several previously unrecognized EHR-embedded biases in a multisite emergency departments (ED) setting. We assessed sex and race differences in EHR data missingness and timeliness of several key ED procedures following ACS suspicion. We additionally conducted a data-driven clustering analysis to detect latent groups of ACS patients. Results 10,043 ACS-associated ED visits were included. We identified sex and race differences in the prevalence of ACS symptoms, data missingness (vitals and labs), and waiting time for troponin order and treatment initiation within 24 hours post-admission. Our cluster analysis discovered four groups of ACS patients corresponding to common symptoms. Additionally, we identified differences in clinical management and patient demographics across these clusters. Conclusions We discovered several sources of bias inherent in the clinical practice of ACS diagnosis and treatment that are also present in EHR data. Our study supports the inclusion of a wider range of symptoms into AI-based diagnosis and risk stratification tools. These models should be also validated in ED admissions without chest pain and in patients who experience a longer waiting time for ED procedures.
更多查看译文
关键词
electronic health records,racial biases,emergency department patients,emergency department,acute
AI 理解论文
溯源树
样例
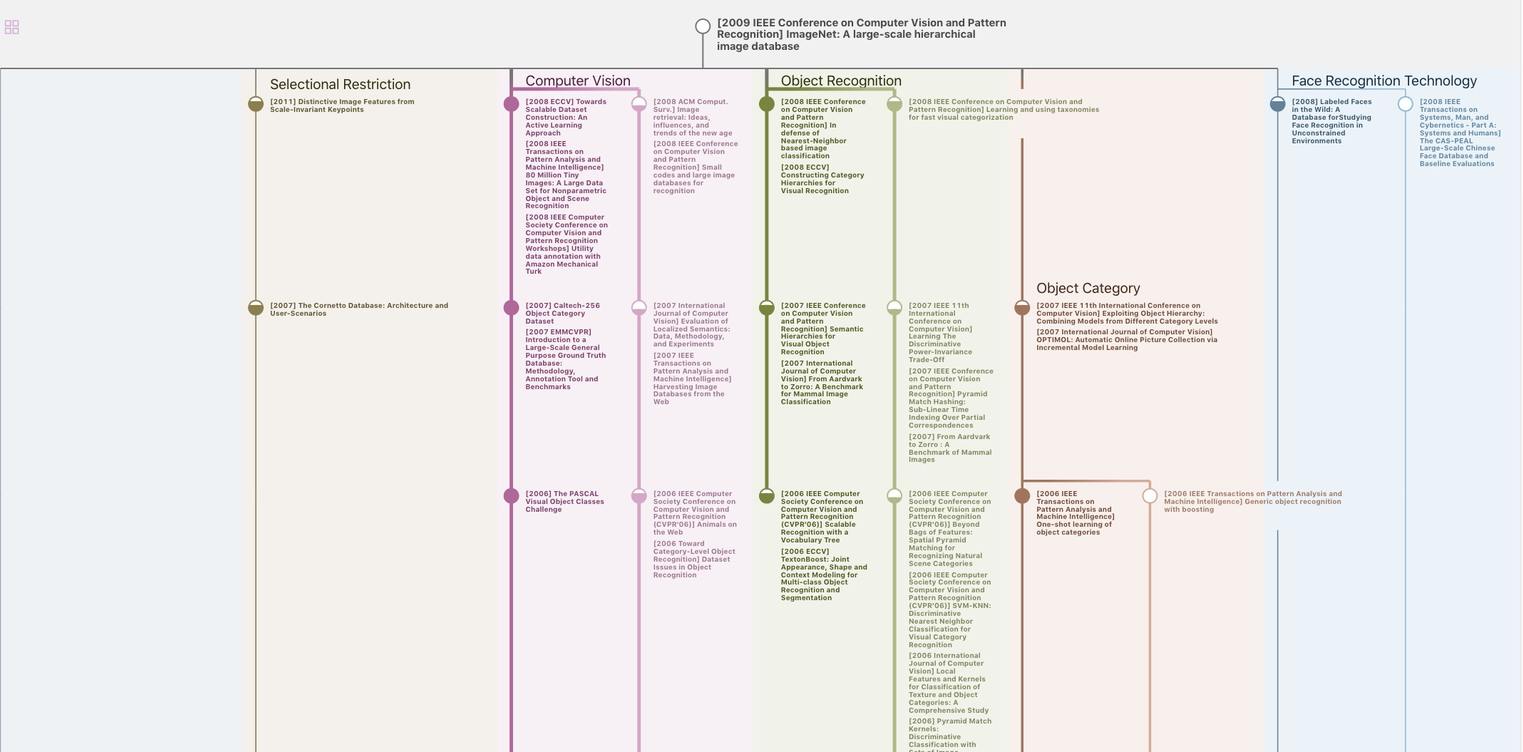
生成溯源树,研究论文发展脉络
Chat Paper
正在生成论文摘要