Early Prediction of Ventilator-Associated Pneumonia in ICU Patients Using An Interpretable Machine Learning Algorithm
crossref(2022)
摘要
(1) Background: Ventilator-associated pneumonia (VAP) causes high mortality among patients with respiratory disease and imposes major burdens on healthcare infrastructure. Models that use electronic health record data to predict the onset of VAP may spur earlier treatment and improve patient outcomes. We developed and studied the performance of interpretable machine learning (ML) models that predict the onset of VAP from electronic health records (EHRs); (2) Methods: We trained Logistic Regression (LR), full feature Explainable Boosting Machine (fEBM), and eXtreme Gradient Boosting (XGBoost) ML models on data from the MIMIC- III (v1.3) database. Model performance was measured by area under the receiver operating characteristic curves (AUCs). We trained a minimal-feature EBM model (mEBM) with features derived from white blood cell (WBC) counts, duration of ventilation, and Glasgow Coma Scale (GCS). Finally, model robustness was evaluated on randomly sparsified EHR datasets; (3) Results: The fEBM model outperformed the XGBoost and LR models at 24 hours post-intubation. The mEBM model maintained an AUC of 0.893. The fEBM model performance remained robust on sparsified datasets; (4) Conclusions: Our novel interpretable ML algorithm reliably predicts the onset of VAP in intubated patients. Integration of this EBM-based model into clinical practice may enable clinicians to better anticipate and prevent VAP.
更多查看译文
AI 理解论文
溯源树
样例
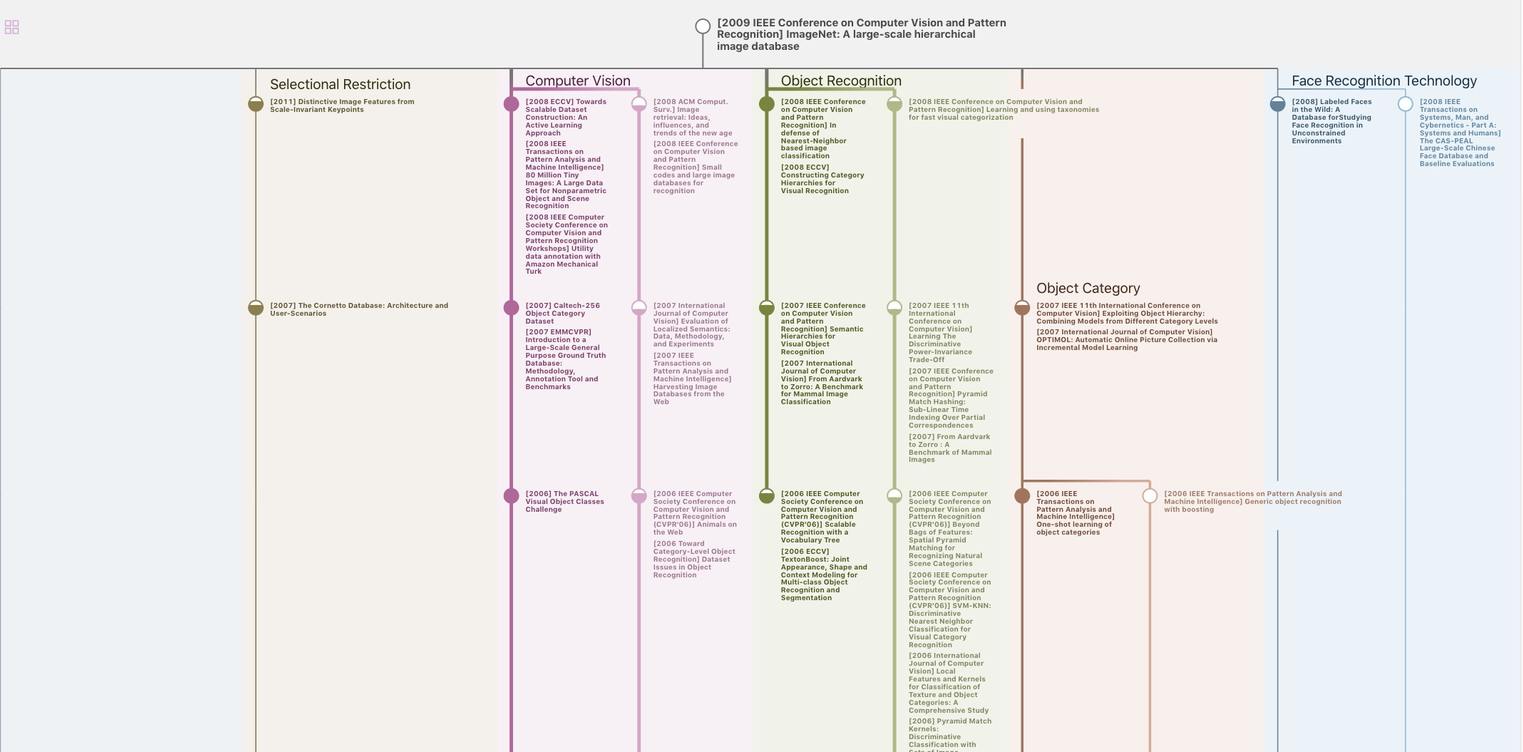
生成溯源树,研究论文发展脉络
Chat Paper
正在生成论文摘要