FCHM-Stream: Fast Closed High Utility Itemsets Mining over Data Streams
crossref(2022)
摘要
Abstract In the data stream scenarios such as the Internet of Things and network click streams, the high-speed, continuous, and endless characteristics of data stream make it a challenging task to quickly mine high utility itemsets in limited memory space. In the data stream scenario, the sliding window model has received extensive research and attention because of its emphasis on recent data. However, in the sliding window model, adjacent windows have a large number of communal batches resulting in a large set of identical itemsets in the resultsets of adjacent windows. At the same time, to provide users with a concise and lossless resultset, a novel algorithm is proposed for mining closed high utility itemsets from data streams, named FCHM-Stream. The algorithm devises a new utility list structure based on batch division. It presents a resultset maintenance strategy based on the skip-list structure to quickly and effectively update the resultset when the window slides which can effectively avoid repeated mining of redundant itemsets. Moreover, various experiments are carried out to compare our method with state-of-the-art algorithms both in static and stream environments. Extensive experimental results show that the proposed algorithm significantly reduces the running time compared to previous algorithms.
更多查看译文
AI 理解论文
溯源树
样例
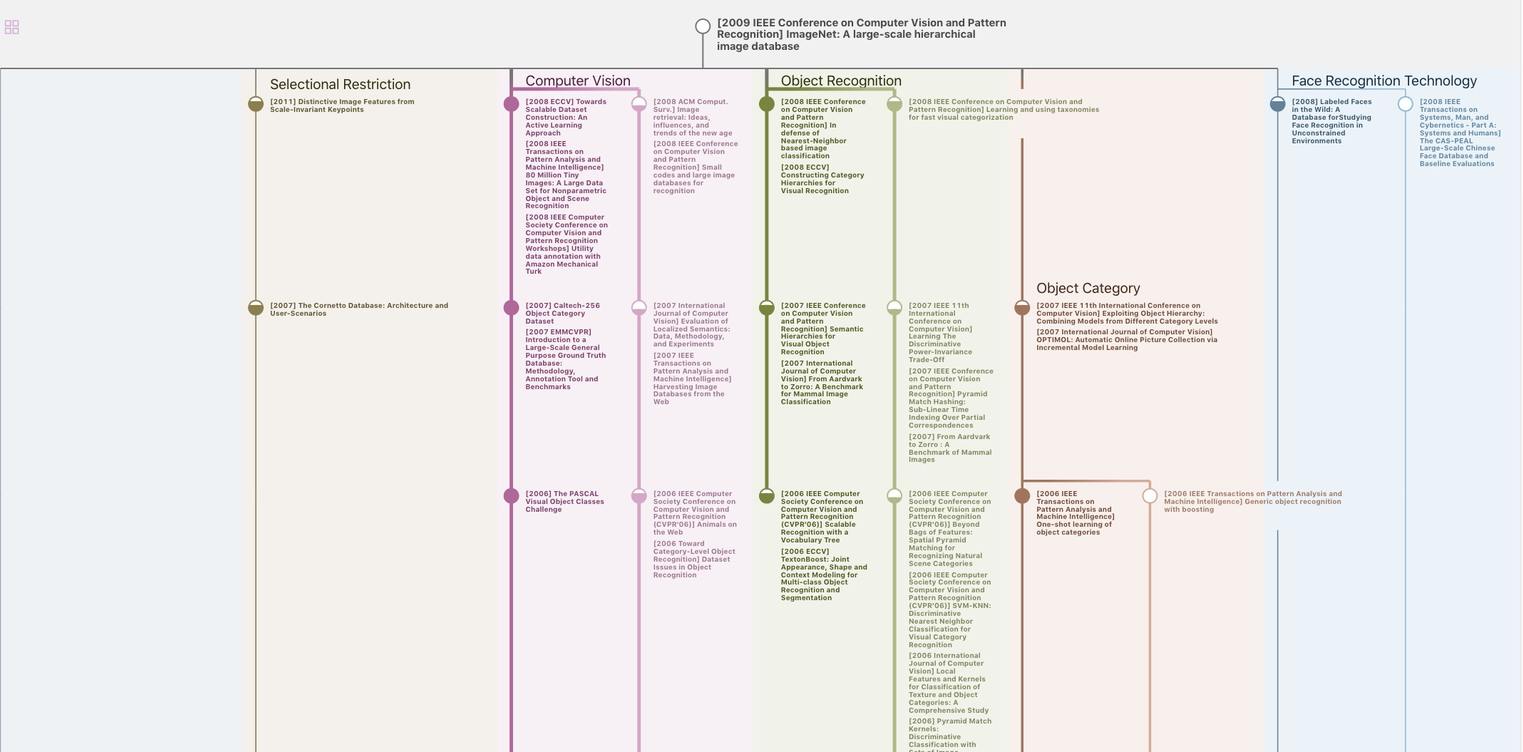
生成溯源树,研究论文发展脉络
Chat Paper
正在生成论文摘要