Deep Learning to Predict Structure-Property Relationships of Polymer Blends
Acs Symposium Series(2022)
摘要
Convolutional neural nets (CNN) are used to classify and predict bulk mechanical properties of a series of polymer blends based on their microstructure, as measured by atomic force microscopy (AFM) using both quantitative and qualitative imaging modes. The polymer blends were 3-component impact copolymers (ICPs) comprising of a polypropylene matrix and various distributions, density, morphology, and size of ethylene-propylene rubbers and associated ethylene inclusions. The models successfully classified the ICPs and predicted the Young’s modulus, flexural modulus, and yield strength. The model was unsuccessful at predicting the impact toughness of the material. The success or failure of the deep learning models provide insight into whether morphological or mechanical properties of the microstructure have a stronger influence on the various bulk mechanical properties.
更多查看译文
关键词
polymer,deep learning,structure-property
AI 理解论文
溯源树
样例
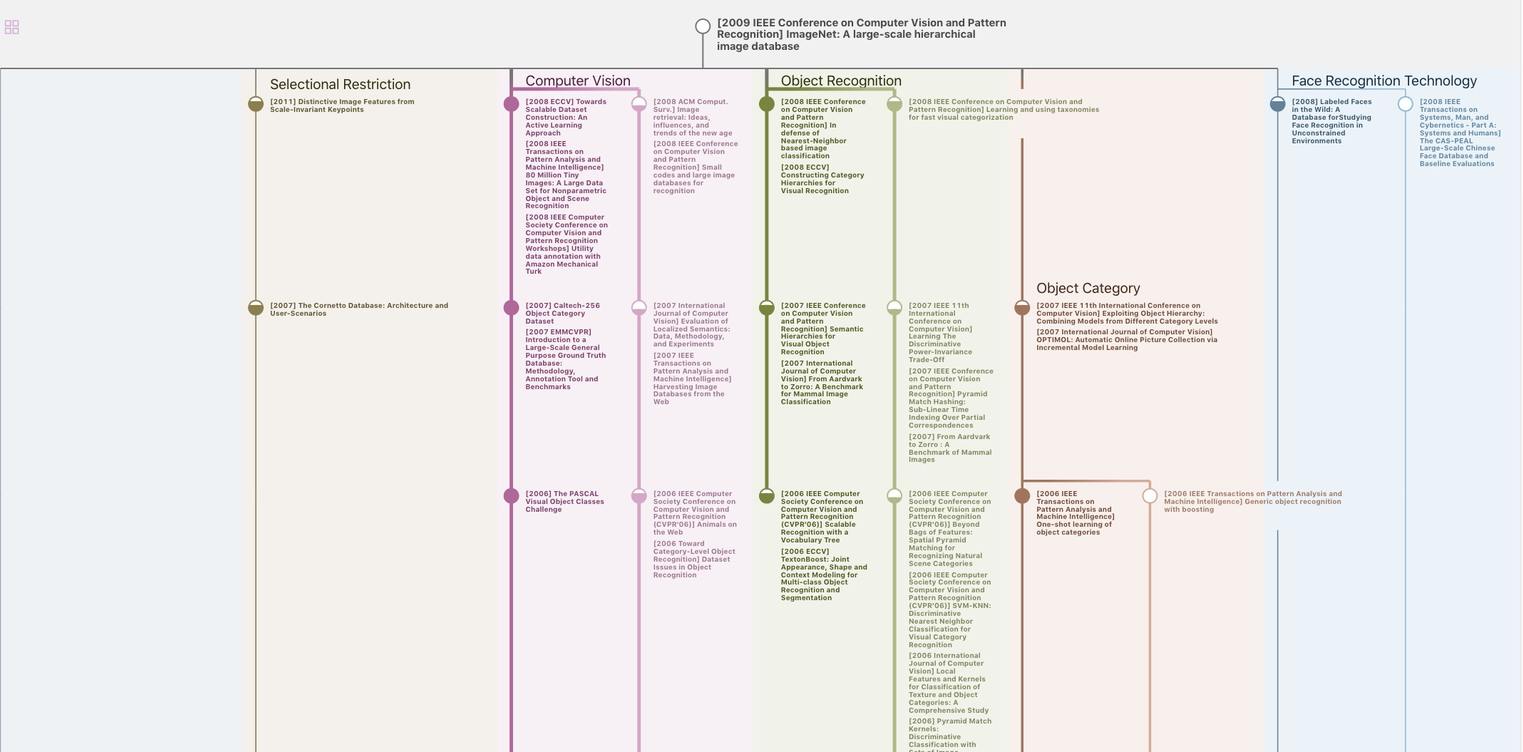
生成溯源树,研究论文发展脉络
Chat Paper
正在生成论文摘要