Abstract 2146: A quantitative in vivo pharmacogenomics platform uncovers biomarkers of therapy response
Cancer Research(2022)
摘要
Abstract Cancer is characterized by heterogeneous genetic alterations that drive changes in cell state that often impact responses to therapy. Recent successes in the development of therapies targeting oncogenes has dramatically improved cancer care for subsets of patients and generated a wave of interest in precision cancer medicines. However, efforts to broadly discover effective targeted therapies have been stymied by a limited understanding of how response to drugs is modulated by tumor genotype. Here, we describe an in vivo functional cancer pharmacogenomics (PGx) platform optimized for the discovery of genetic drivers of response to therapies. By coupling somatic genome editing, DNA barcoding, next generation sequencing, and robust statistical methods with genetically engineered mouse models of human lung cancer, the effects of different candidate therapies can be precisely quantified across thousands of tumors of diverse tumor suppressor genotypes. These tumors are initiated de novo in the native microenvironmental context in mice with an intact adaptive immune system. To illustrate the translational value of our platform, we tested a standard-of-care chemotherapy combination of carboplatin and pemetrexed (carbo/pem) in mice with oncogenic KrasG12D-driven lung tumors with inactivation of 22 putative tumor suppressors. Treatment after 12 weeks of tumor growth for 3 weeks led to greater than 75% reduction in tumor sizes relative to vehicle controls. Surprisingly, although chemotherapy affects cell proliferation pathways rather than a defined genetic component, we identified a diverse spectrum of genotype-specific sensitivity and resistance to carbo/pem treatment in our model. We next determined the extent to which our empirical PGx data could predict human clinical outcomes by analyzing clinicogenomic data from >200 patients with KRAS mutant lung cancer who received combination chemotherapy treatment. Training a multivariate Cox proportional hazards (PH) model on overall survival from treatment initiation using our 22 cancer-associated genes as binary features in combination with baseline clinical data revealed a remarkable agreement between the Cox-PH coefficients and our empirical PGx data. Further analysis of the human survival data using multiple machine learning (ML) methods identified Random Survival Forests (RSF) as the top performing model. Importantly, using mouse empirical PGx data to rank genes for feature selection significantly improved the predictive power of the ML model. Together, these results demonstrate how leveraging our PGx platform together with human data within a machine learning framework may improve the prediction of patient clinical outcomes, and thus profoundly impact our ability to realize the promise of precision medicine. Citation Format: Michael Rosen, David Amar, Ian Winters, Hira Rizvi, Wensheng Nie, Gregory Wall, Dmitri Petrov, Monte Winslow, Charles Rudin, Joseph Juan. A quantitative in vivo pharmacogenomics platform uncovers biomarkers of therapy response [abstract]. In: Proceedings of the American Association for Cancer Research Annual Meeting 2022; 2022 Apr 8-13. Philadelphia (PA): AACR; Cancer Res 2022;82(12_Suppl):Abstract nr 2146.
更多查看译文
关键词
biomarkers
AI 理解论文
溯源树
样例
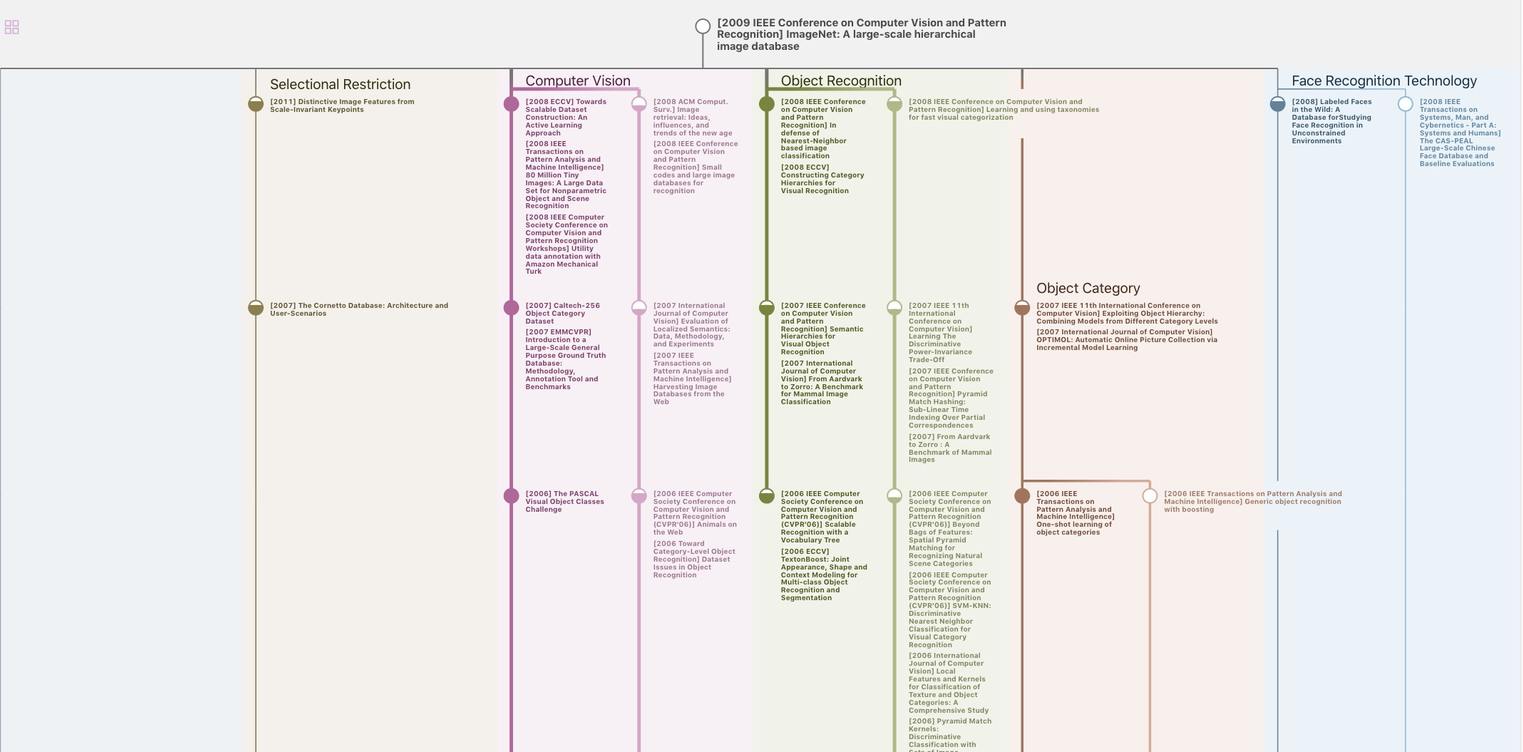
生成溯源树,研究论文发展脉络
Chat Paper
正在生成论文摘要